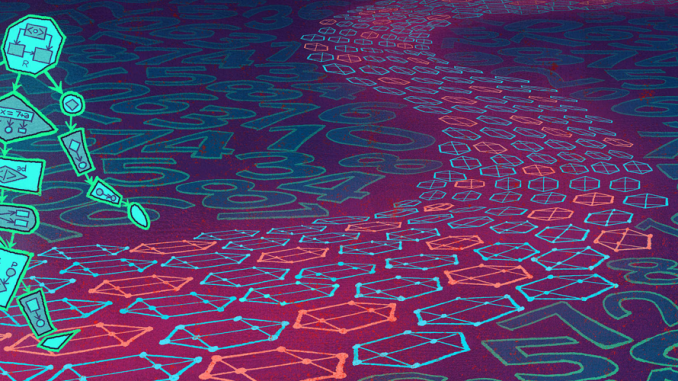
Algorithms: Personalised Recommendation and Public Decision-Making
Algorithms are making decisions for us in our daily lives. When we add friends on Facebook from the list of “people you may know” or buy something recommended by Amazon, the algorithms have an impact on our decisions. The platforms collect our actions and process them with algorithms. With these data, the platforms recommend content to us according to our habits and preferences. Although the recommendation is based on our personal information, algorithms are influencing our decisions and behaviors.
Public decision-making is also influenced by algorithms. Algorithms implement machine learning and prediction based on a large number of datasets, improving the efficiency of people analyzing data and helping to make decisions. Some algorithm models and algorithm-based artificial intelligence have been applied to automated decision-making in public fields such as healthcare, business, and public administration. For example, algorithms are employed in the United States to determine who would be eligible for early release from jail as well as for criminal punishment (Araujo, etc., 2020).
The reason algorithms play a role in decision-making is because of their data processing capabilities. An algorithm is a set of instructions or rules used to solve specific problems or perform specific tasks that extract useful information from data through processing and analysis to help make decisions. In many cases, algorithms can quickly and accurately analyze and process large amounts of data, enabling decision-makers to better understand and solve problems.
However, the involvement of algorithms in the personalized recommendation and public decision-making brings potential concerns, which raise requirements for algorithmic governance. I will discuss these in the following two parts.
Several Concerns in Algorithm Decision-Making
Invisibility of Algorithms
The invisibility of algorithms mainly refers to the opacity of data processing rules. People can only access the results output by an algorithm but cannot understand how it processes the data. Pasquale (2015) used the term “black box” to indicate the secrecy of algorithms to the public. Due to the inherent invisibility of algorithmic languages, it is impossible to achieve complete transparency in the automated decision-making process of algorithms. Users cannot correct the output of the algorithm, even if it misjudges their preferences, because they have no idea what actions are causing them to be recommended videos they don’t like or people they don’t know. As a result, we passively accept anything recommended by the algorithm, but cannot provide effective feedback based on what we expect.
In addition, because of the “black box” nature of the algorithms, public decisions made by them may not be transparent. This could lead to public mistrust and protests, which could affect the effectiveness of the decision’s implementation. As the decision-making process involving algorithms is opaque, the public may find it difficult to understand the rationality and accuracy of the decision, making it difficult for them to accept the decision’s results. Algorithms are not neutral technologies, they are designed with authority and unfairness (Pasquale, 2015). Data from the general population is unilaterally collected without allowing them to know how it will be used to make choices. This may also lead to unreasonable public decision-making, which harms the public interest.
Another invisibility of algorithms lies in the collection and use of user data. Algorithm recommendations are working with user information collected by the platforms. Platforms often acquire the right to collect data in the form of a Term of Use. However, people have a limited understanding of the specific purpose of the data. This leads to users losing autonomy over personal information. For example, according to the Term of Use of Reface (2021), they keep the right to change their services and costs “at any time for any reason without advance notice.” Users won’t be informed of the change, so their personal information could be used for unintended purposes. Similarly, during the #DeleteFacebook movement, some users found that Facebook still tracks you after you deactivate account, which means that deleting Facebook cannot avoid Facebook from continuously gathering their information (Alfred, 2019).
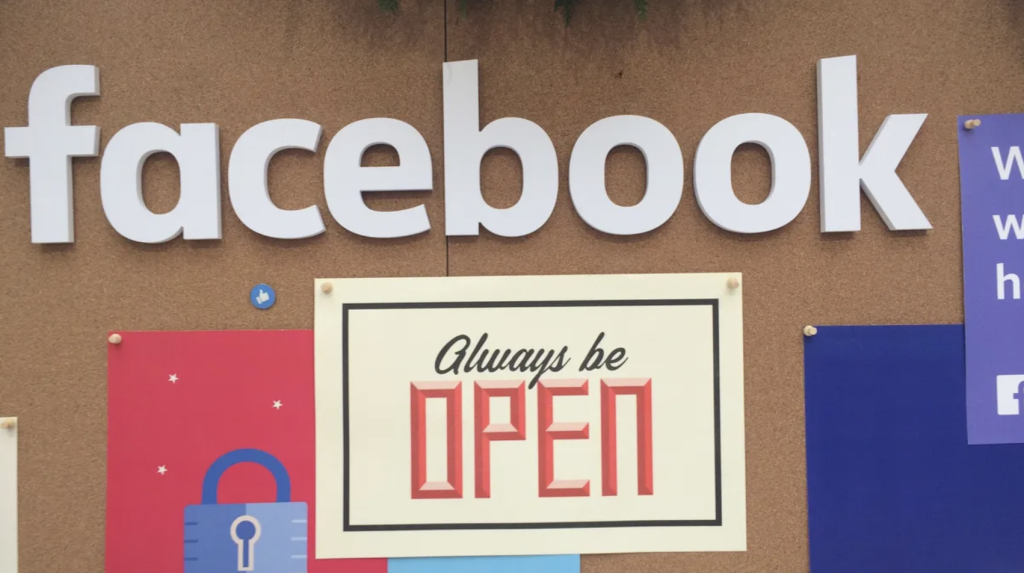
A sign from Facebook’s privacy pop-up in New York. (Alfred, 2019)
The Filter Bubbles
Pariser (2011) proposed the concept of “filter bubbles”, which refers to the exclusive information space created by the media by separating different opinions and preferences. Algorithms could potentially lead to filter bubbles due to their reinforcement of original user preferences. An algorithm’s recommendation is based on big data computing, accurately targeting user actions and meeting their emotional needs. The user’s original attitude and viewpoint are affirmed, and their exposure to dissenting information is reduced. As a result, users may be unaware of alternative perspectives, which can limit their understanding of complex issues and lead to a polarization of opinions. This can be particularly challenging in political elections, where the social media algorithm recommends content that benefits the voters’ originally preferred candidate and where filter bubbles can potentially limit exposure to diverse political voices.
Nevertheless, some people believe that there is insufficient evidence to demonstrate a clear correlation between algorithms and filter bubbles. During the 2016 US presidential election, when using Google Search with Hillary and Trump as keywords, users from different geographical locations and political inclinations received similar content (Nechushtai & Lewis, 2019). The debate about “filter bubbles” reflects people’s different views on how algorithms affect people’s judgments and decisions. The focus of the debate is whether the algorithm only provides content that meets the user’s original preferences. It depends on the logic of the algorithm, which is created by developers. From a business perspective, catering to user preferences is often a more profitable option. The logic of algorithm recommendation is consistent with the formation of filter bubbles. Therefore, we can conclude that the algorithm has at least the potential to enhance the filter bubbles.
Algorithmic Bias and Unfairness
Algorithms are increasingly being employed at the public level to decide how to allocate resources, create policies, and enforce the law. However, algorithmic bias has led to some inappropriate public decisions. To identify places where crime is likely to develop, for instance, some cities use predictive policing algorithms, intending to stop it before it starts. These algorithms have drawn criticism for continuing racial prejudice and unfairly favoring communities of color. The Chicago police’s 2017 “predictive policing” based on big data and algorithm was criticized for its embedded bias. This algorithm aimed to predict potential violent offenders and take measures such as warning against violent crimes (William & Andi, 2017). It was pointed out that the data tracked by the police reflected “long-standing institutional biases along income, race, and gender lines.” Therefore, the expanded use of predictive policing will harm vulnerable groups.
The reasons for algorithmic bias are complex. It can be due to the biases of algorithm developers or logical flaws in the algorithm itself, as well as biased information in the database used for algorithm learning. These algorithmic biases are reflected in their output, as well as the recommendations and decisions, which can lead to infringement on individuals and unfairness towards groups. For example, algorithms recommend discriminatory content to users, and some groups are tagged with negative tags. According to Noble (2018), the suggestions and content generated by Google Search reflect racism and sexism. An abandoned Amazon recruitment algorithm downgrades resumes containing the word “woman” (Jeffrey, 2018). That is to say, algorithmic bias has a negative impact on both personalized recommendations and public decision-making.
Algorithmic Governance
Visibility and Transparency
Algorithm developers should consider enhancing visibility and transparency, as this can improve the accuracy and credibility of the algorithms. Then they can better meet the requirements of users and the public. Firstly, enhancing visibility can help users better understand how the algorithm recommends content to them. Users can see how the algorithm processes its data and what rules it employs to make recommendations. This can help users better grasp how the algorithm works and better correct errors in the algorithm’s output. Secondly, enhancing transparency can help the public better understand the algorithm’s involvement in public decision-making processes. This can increase public trust and support for decisions and reduce public dissatisfaction and protests.
Protecting Digital Rights and Privacy
The platform should protect users’ digital rights and privacy when collecting and processing their data. Firstly, users have the right to know and decide how their data is collected and used. Besides the Term of Use, the platform should inform users of the specific usage of their data. Secondly, the platform should grant users permission to access, correct, or delete their data. Users’ information autonomy should not be infringed by algorithms. In addition, the platform should protect user data to avoid the risk of unauthorized access, leakage, or abuse.
Diversity and Balance
To avoid creating “filter bubbles,” algorithms should generate diverse and balanced content. In addition to content based on user preferences, various types of content should be recommended rather than only content that is similar to the user’s previous browsing history. This ensures that users receive a wider range of information. Moreover, the algorithm should ensure that the recommended content sources are relatively balanced and avoid being too biased towards a specific source. This enables users to have access to diverse perspectives and voices, rather than being limited to their original ones.
Avoiding Algorithm Bias
Avoiding algorithmic bias requires attention at each stage. Developers should consider the needs and interests of different groups when designing algorithmic logic to ensure the fairness of the algorithm. In addition, they should make sure of the diversity and comprehensiveness of the algorithm database, which calls for work in both data collection and data screening. A review and feedback system should also be established to correct biased outputs and improve algorithms.
Government Involvement
Governments are crucial for algorithmic governance. They should focus on the technical ethics of algorithms and develop laws or policies to ensure the rational use of algorithms, protect digital rights and privacy, and make provisions for issues such as algorithmic bias. Some relative documents have been published on algorithmic issues. For example, European Data Protection Supervisor (2015) published Meeting the Challenges of Big Data: a Call for Transparency, User Control, Data Protection by Design and Accountability, proposing the fairness of the algorithm and the importance of protecting vulnerable groups in big data.
Conclusion
The ability of algorithms to process data makes them effective in personalized recommendations and public decision-making. However, the algorithm itself has invisibility and leads to issues such as filter bubbles, algorithm bias, and unfairness. These have put forward requirements for the governance of algorithms. It is unrealistic to rely solely on algorithms for fully automated decision-making. The joint decision-making between humans and algorithms is the focus of algorithm governance, which requires the cooperation of developers, platforms, governments, and other parties.
Reference
Alfred, N (2019). Facebook still tracks you after you deactivate account. Retrieved from https://www.cnet.com/news/privacy/facebook-is-still-tracking-you-after-you-deactivate-your-account/
Araujo, T., Helberger, N., Kruikemeier, S., & de Vreese, C. . (2020). In AI we trust? Perceptions about automated decision-making by artificial intelligence. AI & Society, 35(3), 611–623. https://doi.org/10.1007/s00146-019-00931-w
European Data Protection Supervisor. (2015). Meeting the Challenges of Big Data: a Call for Transparency, User Control, Data Protection by Design and Accountability. https://edps.europa.eu/sites/edp/files/publication/15-11-19_big_data_en.pdf
Jeffrey, D (2018). Amazon scraps secret AI recruiting tool that showed bias against women. Retrieved from https://www.reuters.com/article/us-amazon-com-jobs-automation-insight-idUSKCN1MK08G
Nechushtai, E., & Lewis, S. C. (2019). What kind of news gatekeepers do we want machines to be? Filter bubbles, fragmentation, and the normative dimensions of algorithmic recommendations. Computers in Human Behavior, 90, 298–307. https://doi.org/10.1016/j.chb.2018.07.043
Noble, S. U. (2018). Algorithms of Oppression: How Search Engines Reinforce Racism. NYU Press. https://doi.org/10.18574/9781479833641
Pariser, E. (2011). The filter bubble : what the Internet is hiding from you. Viking.
Pasquale, F. (2015). The black box society : the secret algorithms that control money and information. Cambridge: Harvard University Press.
Reface. (2021). Terms of use. Retrieved from https://hey.reface.ai/terms-history/
William, I., & Andi, D. (2017). Column: Why big data analysis of police activity is inherently biased. Retrieved from https://www.pbs.org/newshour/nation/column-big-data-analysis-police-activity-inherently-biased
Be the first to comment