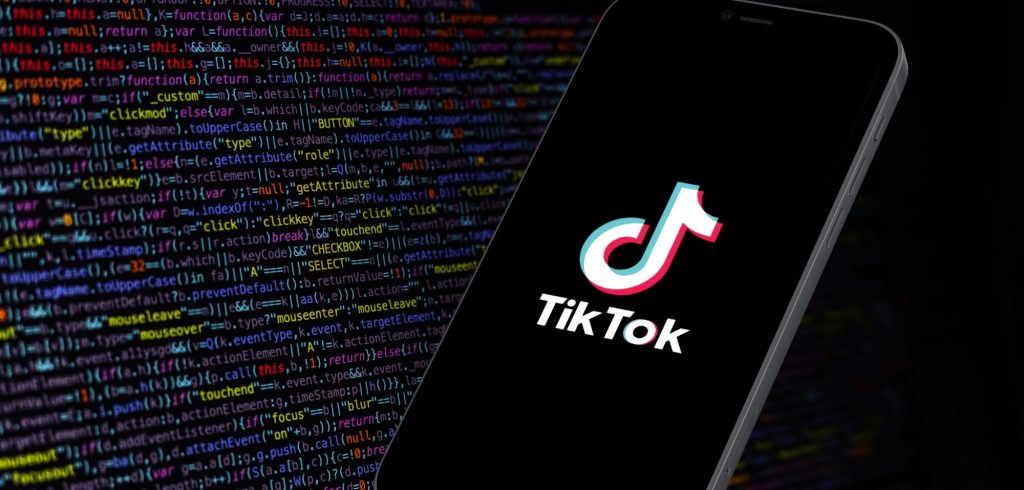
AI application in TikTok
Artificial intelligence is considered a transformative technology for the digital age and will be likened by many to the driving force of the digital age. (Chan-Olmsted, 2019). TikTok is a short video social media platform where people upload videos they create and shoot to document their lives and get traffic. In addition, the artificial intelligence technology used by TikTok mainly includes machine learning and data analysis to provide users with personalised content through a content recommendation system.
TikTok’s artificial intelligence system focuses on users’ various actions and habits when using the software, including likes, comments, retweets, viewing time, and interest preferences. Through inductive processing and analysis of these data, users’ interests and preferences can be predicted, and relevant content can be pushed. Artificial intelligence uses deep learning technology to tailor the content users are interested in pushing, thereby increasing the duration of software use and user engagement.
With the wide application of artificial intelligence technology, the ability of media companies to push large-scale personalised video content experiences has been rapidly improved (Chan-Olmsted, 2019). People’s requirements for Internet information are increasingly refined and customised, so personalised information services are also widely used by users. Therefore, the personalised recommendation system comes into being, aiming to deeply understand the specific interests, preferences and needs of users and provide users with highly customised information services.
The media sector is expected to benefit from the advancement of AI through deep video analysis. This will enable better classification and tagging of video content, further improving the accuracy of content linkage, search, and association (Chan-Olmsted, 2019). The application of AI on TikTok has not only significantly increased user engagement, content diversity, and user satisfaction through personalised content recommendations. However, it has also changed the traditional way content is discovered and distributed. Personalised service no longer meets the general interests of the public; it is more focused on meeting the specific needs of individual users in the subdivided areas of interest, whether the mainstream hot field or the long-tail niche field.
Process and outcome customisation is a primary objective and feature of algorithmic selection applications (Just & Latzer, 2017). TikTok’s “For You” page exemplifies this, featuring a mix of videos from established and budding bloggers that reward quality content based on views. This unique approach to content discovery and delivery not only helps users explore a wide range of content that matches their preferences and extends their stay on the platform, but it also facilitates the discovery of new ideas and creators and stimulates creative vitality within the platform.
In addition, personalised recommendations allow users to feel that their unique tastes are recognised and satisfied, which not only improves overall user satisfaction but also provides a channel for content creators vv. A primary goal and feature of algorithmic selection applications is the personalisation of processes and results (Just & Latzer, 2017). This win-win mechanism makes TikTok a highly attractive platform that continues to attract and retain users while supporting and encouraging diversity and innovation among content creators.
Automation and personalisation
In today’s Internet era, with the continuous refinement of users’ demand for content, algorithms have played a crucial role in the media field, making the production and consumption process of media more and more dependent on the support of automation technology. Automation, as the core feature of the algorithm, is widely used in every link of data collection, processing, analysis, modelling and prediction, and the process is completely automated. For example, Google’s statistical machine translation model is a typical example, where the entire process, including data storage, analysis, processing, training, and model prediction to generate results, is entirely automated. This automated process minimises the impact of human cognitive limitations and subjective participation on the outcome, providing strong support for decision-making through automated interpretation and prediction of algorithms.
Mager (2012) points out that algorithms play a particularly prominent role in media, where media production and consumption processes are increasingly automated and determined by algorithms. This kind of efficient, automated machine algorithm that processes data is becoming increasingly popular as a fundamental tool for decision-making. Domingos (2015) further emphasises that, just as the Industrial Revolution automates handicrafts and the information Revolution liberates mental labour, the development of machine learning is the automation of automation itself, marking a new level of automation by algorithms and heralding broad economic and social changes that the intelligent algorithm revolution will bring (Kitchin, 2017).
Content management is almost completely automated in a digital entertainment platform like TikTok. The algorithm decides what content is recommended to the user without human intervention. This automation not only increases efficiency but also makes content distribution more precise, which in turn increases user engagement. Automation supports the scalability of TikTok content management, enabling the platform to manage and deliver enormous amounts of content effectively. Through sophisticated algorithms, TikTok automates the content recommendation and review process, which is critical to handling the platform’s vast and growing content library.
The benefits of automation are manifold, including significantly improved operational efficiency, the ability to manage a global platform, and the ability to quickly spot trending content. This efficiency is critical to keeping users engaged and supporting the platform’s rapid growth. By leveraging automated systems, TikTok can effectively manage its vast content library, ensuring that fresh and relevant choices are presented to users. Automation helps identify and promote popular content, enabling TikTok to quickly adapt to changing user interests and global trends, thus enhancing the vitality and appeal of the platform.
Algorithm governance
As seen from the above discussion, algorithms have become the key to transforming low-value raw data into high-value derived data in the era of big data (Gritsenko & Wood, 2022). They continue to extend personalisation and efficiency in the construction of society, introducing multiple risks and reducing the transparency and control of decision-making (Pasquale, 2015). This has led to deep thinking on the critical issue of algorithm governance. The goal of algorithm governance is to promote the development of artificial intelligence and automation technologies while ensuring that they comply with ethics and laws, promote the standardised application of algorithms in the digital economy era, and protect users’ rights and interests.
With the advent of algorithms, black-box and algorithmisation have been diffused in various fields of science, engineering and society. Each field has its algorithm system, and the internal operation of these algorithm systems is uncertain and unobtainable. Pasquale (2015) pointed out in his research that there is often a “black box” phenomenon behind algorithms, which means that the working principle of algorithms is opaque to most people. He believes there are three primary motivations for creating such black boxes. The first is Real secrecy, which means that while people emphasise the objectivity and neutrality of algorithms, they ignore the process and rules used to select them. Then there is “Legal secrecy,” in which companies or individuals restrict or refuse to disclose their algorithms on the grounds of protecting trade secrets or complying with existing legal frameworks. Finally, “Obfuscation” increases regulatory difficulty by providing information that is too complex or difficult to understand, thus making it difficult for the government and the public to monitor the use of algorithms. In short, Pascual stressed the importance of algorithmic transparency and pointed out some of the challenges that exist in reality.
TikTok’s recommendation algorithm was selected as one of MIT Technology Review 2021’s “Top 10 Global Breakthrough Technologies.” personalised push mechanism. By analysing user behaviour data to achieve accurate content recommendations, this approach may lead to user stereotypes, information leakage risk and content homogenisation problems. In addition, the pricing strategy of the platform also reflects algorithmic discrimination, that is, different pricing strategies are adopted for different users through user data analysis. This phenomenon of “big data killing” is a violation of the rights and interests of consumers. The selectivity of content push and the influence of social relationships are also essential aspects of platform algorithm discrimination, which tends to push popular or platform-first content, ignoring high-quality but not popular content, intensifying unfair competition for content, and making it difficult for some content creators to get exposure. Recommendations based on a user’s social connections can lead to the marginalisation of specific users, exacerbating social estrangement and group bias.
In addition, the US media and Congress have successively questioned TikTok, fearing that it may be used as a tool to incite the American people and carry out social mobilisation. Such concerns are based on the assumption that the Chinese government uses soft power to manipulate Bytedance to influence TikTok’s video recommendation algorithms. Although this allegation is unsubstantiated, it highlights the urgency and complexity of algorithmic governance globally.
Crawford, K. (2021) proposed that, in the long run, understanding and controlling system accountability models depend on the concept of transparency. There are severe limitations to making algorithms transparent enough to gain insight into how they work and are managed. In the field of artificial intelligence in particular, there is no single “black box” that needs to be uncovered, but rather a complex interlace of many power systems that makes full transparency an unattainable goal.
Limited transparency
While it is a principle that algorithms are not public, it is also a principle that people should have the right to demand fairness. Algorithms with a monopoly position and intended to provide a universal public service should have the right to demand their disclosure. Because algorithms with monopoly status limit people’s choices and exert a significant impact on individuals, people should have the right to know.
Transparency may seem like the right thing to do in some cases to help the public understand how decisions are made, but it doesn’t work when it comes to national security. Once the inner workings of a particular black box are made public, someone can circumvent the secrecy system and invalidate the algorithm (Dormehl, 2014).
The government stepped in to strengthen the supervision of third-party algorithms
The platform, users, government and society have jointly built a standardised mechanism integrating supervision, evaluation and accountability by virtue of their respective advantages. According to the differences in the types, causes and consequences of short-video platform algorithms, different coping strategies and punishment measures are applied, respectively, to achieve differentiated accountability for different responsible subjects.
In 2017, the Public Policy Committee of the American Computer Society issued six guidelines for algorithm governance: 1) The knowledge principle, which requires the designers and interested parties of algorithms to disclose the possible bias and potential harm of algorithms; 2) The principle of inquiry and appeal to ensure that those negatively affected by the algorithm can question and appeal; 3) Algorithm responsibility identification; 4) Interpretation principles, requiring interpretation of algorithmic decision principles and results; 5) Data source disclosure; 6) Auditable principle (Xu, 2019).
Legal statute
Law is the logical bottom line of agile governance and a powerful weapon to maintain algorithmic fairness. To control platform algorithms’ discrimination, the government regulates the research, development, and application of platform algorithms through legislative means, standardises and prevents the infringement of users’ legitimate rights and interests by short video platforms, and issues guiding laws and regulations in a timely manner to improve the legislative system.
In addition, algorithms that discriminate against a particular class of people and violate citizens’ right to equality, although it does not necessarily need to be disclosed to the public, people have the right to Sue and have it reviewed by a judge. The non-disclosure of algorithms is the principle, and the disclosure is the exception. If there is a need for disclosure, there also needs to be a law clarifying which algorithms should be made public and how.
Conclusion
The analysis above highlights the complexity and multi-dimensionality of algorithmic governance, which necessitates multi-party participation and continuous efforts. While discussing how AI and personalised recommendation systems can enhance the user experience, it is also essential to consider the potential challenges related to privacy, autonomy, and social impact these technologies may pose. As a product of the significant data era, algorithms have the potential to transform the value of data and promote economic development and social progress. However, it is essential not to ignore the attendant risks and challenges. By implementing and following the principles and standards of algorithmic governance, we can strike a balance between promoting technological development and safeguarding social justice.
Reference
Crawford, K. (2021). The Atlas of AI: Power, Politics, and the Planetary Costs of Artificial Intelligence. Yale University Press.
Dormehl, L. (2014). The formula: How algorithms solve all our problems . . . and create more. Perigee Books.
Domingos, P. (2015). The Master Algorithm: How the Quest for the Ultimate Learning Machine Will Remake Our World. Basic Books.
Gritsenko, D., & Wood, M. (2022). Algorithmic governance: A modes of governance approach. Regulation & Governance, 16(1), 45-62.
Just, N., & Latzer, M. (2017). Governance by algorithms: reality construction by algorithmic selection on the Internet. Media, Culture & Society, 39(2), 238-258. https://doi.org/10.1177/0163443716643157
Kitchin, R. (2017). Thinking critically about and researching algorithms. Information, Communication & Society, 20(1), 14-29.
Mager A. Algorithmic ideology. Information[J]. Communication & Society, 15(5), 269–787.
PASQUALE, F. (2015). INTRODUCTION: THE NEED TO KNOW. In The Black Box Society: The Secret Algorithms That Control Money and Information (pp. 1–18). Harvard University Press. http://www.jstor.org/stable/j.ctt13x0hch.3
Xu F. (2019). Legal regulation of artificial intelligence algorithm black box: A case study of intelligent investment Counsel. Oriental law (6), 78-86. The doi: 10.19404 / j.carol carroll nki DFFX. 2019.06.002.
Be the first to comment