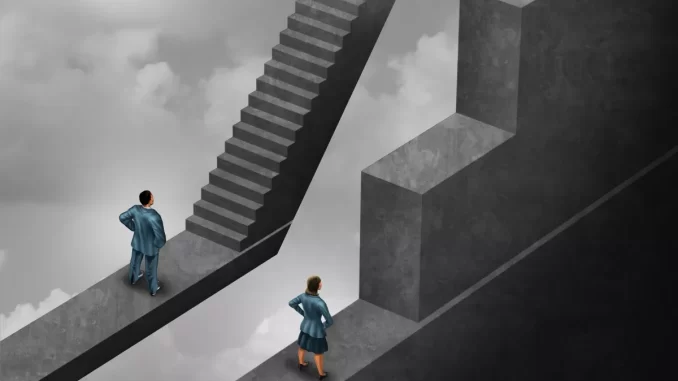
Introduction
Today, we live in an era surrounded by AI algorithms. Algorithmic decision-making with big data as the key driver has penetrated every aspect of our lives. From online shopping to social behavior and other aspects, algorithms are changing the way we live.
In an ideal state, the algorithm can transform low-value original data into high-value derived data and output meaningful calculation results (Gritsenkod, 2022). However, the subjective bias of algorithm engineers and the information bias of big data are often mixed in the process of operating the algorithm, resulting in the loss of the objectivity and neutrality of the algorithm technology in the real application of data processing. In turn, AI algorithms will have different treatment behaviors and discriminatory behaviors for different groups of people, which is what we call algorithmic discrimination.
Invisible Injustice on the Internet
Algorithmic discrimination is a discriminatory behavior carried out by means of algorithms, which refers to the systematic and repeatable unfair treatment of specific groups caused by data analysis (Nachbar, 2020). It has been existed for a long time and has yet to be fully solved. For example, in 2021 the recruitment company LinkedIn was accused of using a sexist recruitment algorithm and the good research also revealed that Google’s advertising system discriminates against the gender bias when displaying career-related ads in 2023.
As a computational method, the algorithm does have technical neutrality, but the application of any Internet algorithm will carry human will behavior, and the database used by the algorithm is also based on the data generated in the past reality, which often contains some unavoidable biased questions and attitudes left over from history. In the calculation process of the algorithm, due to the complexity of the algorithm itself and the exclusive business policy of technology companies, users sometimes do not know the calculation process and the hidden intention of the algorithm. Therefore, the algorithm is like an unknown black box to the public, which will bring hidden injustice into our society (Bartosz, 2023). That’s why we call it the invisible arbiter behind the Internet.
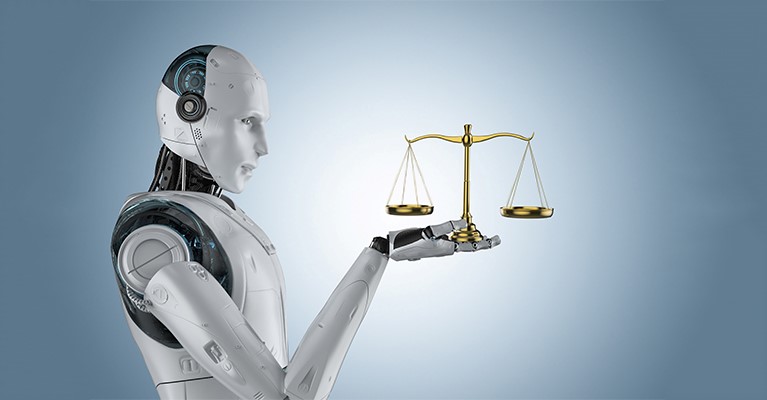
Characteristics of Algorithmic Discrimination
Once discrimination is placed in the environment of the Internet, it has already been a dangerous arbiter with two unique characteristics below, which often produces more serious and more widespread discriminatory consequences.
Concealment
Due to the high degree of professionalism, a huge “digital divide” has been formed between algorithm designers and ordinary users, which causes ordinary users simply cannot understand the process and decision structure of algorithm from the level of professional program code. Besides, the algorithm black box also makes the algorithm decision-making process in a state of opacity, which results ordinary users often can only get the final decision result, and passively accept the result. Therefore, it is well hidden behind the Internet, making people difficult to detect.
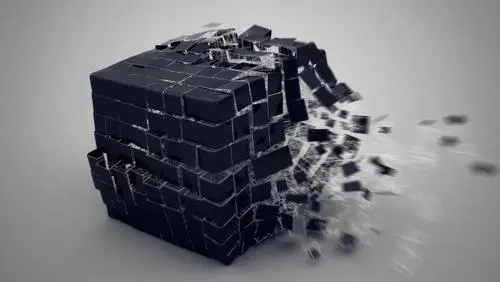
Irreversibility
The whole algorithm running program is the process from “input” to “output”. When algorithms output discriminatory outcomes or have discriminatory harmful consequences, there is simply no way to reverse them because the algorithm may decide from the start which groups of people should see what information and which content should not be recommended based on the user’s personal characteristics. Furthermore, the output of this discriminatory result will be further fed back to the original circulation system and used in the next calculation, resulting in further discriminatory circulation. Thus, because of the constant repetition and the linear damage, it is irreversible.
Case Study:How does Algorithmic Discrimination Harm Our Lives?
Discriminations under different user portraits
User portrait is a process of labeling based on user information data (Yuan, 2023). By obtaining the user behavior habits and establishing a large database is the underlying operating logic of AI algorithms to depict user portraits. The algorithm will apply some differential treatment based on the inherent “stereotype” created during the users’ Internet browsing; in simple terms, it will target and give distinct service supplies to different user groups in an unequal way. For example, the Internet may tend to push more violent and negative content to minority users, and it may display different investment ads specifically for men and women. These discriminatory acts deprive different groups of opportunities and channels to obtain information, making them live in their own information cocoons.
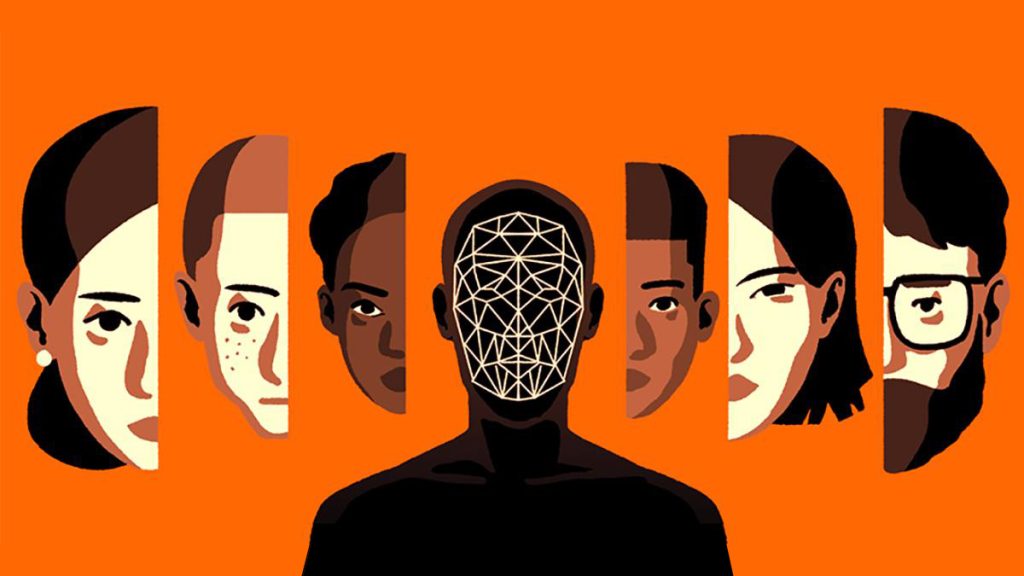
In May 2022, the U.S. Equal Employment Opportunity Commission (EEOC) filed a lawsuit against three of iTutorGroup’s companies – iTutorGroup, Inc., Shanghai Ping’An Intelligent Education Technology Co., Ltd., and TutorGroup Limited (collectively, iTutorGroup). According to the EEOC, iTutorGroup programmed its online recruitment software to automatically reject older applicants, disqualifying women over 55 and men over 60 from consideration. In this case, the behavior of the iTutorGroup company is a typical case of algorithmic discrimination by profiling users. The basic principle of algorithmic decision-making is to use algorithms to mine and identify the features and categories of big data users, and then make corresponding algorithmic decisions with the help of algorithmic models. Algorithms have the appearance of neutrality, while discrimination comes from people’s subjective perceptions. The company created a detailed portrait of older users, and discriminatory ideas about them permeate their hiring algorithms. For the users who used this recruitment platform, they had no chance to learn the internal algorithm mechanism of the recruitment platform. Thus, due to the concealment and injustice of these algorithms, discriminatory results eventually happened to the users, which not only caused the consequences of unequal job opportunities for job seekers but also caused the phenomenon of unfair career development. This kind of employment discrimination makes job seekers miss job opportunities in the process of job hunting because some personal characteristics unrelated to work have been screened by “big data-algorithm”, which is also a serious violation of the legitimate rights and interests of job seekers and workers and breaks the competition rules of the labor market.
As a result, in 2023 the US District Court announced that iTutorGroup would pay $365,000 and furnish other relief to settle an employment discrimination lawsuit filed by the EEOC. This also provides a sample for our legal governance of algorithmic discrimination.
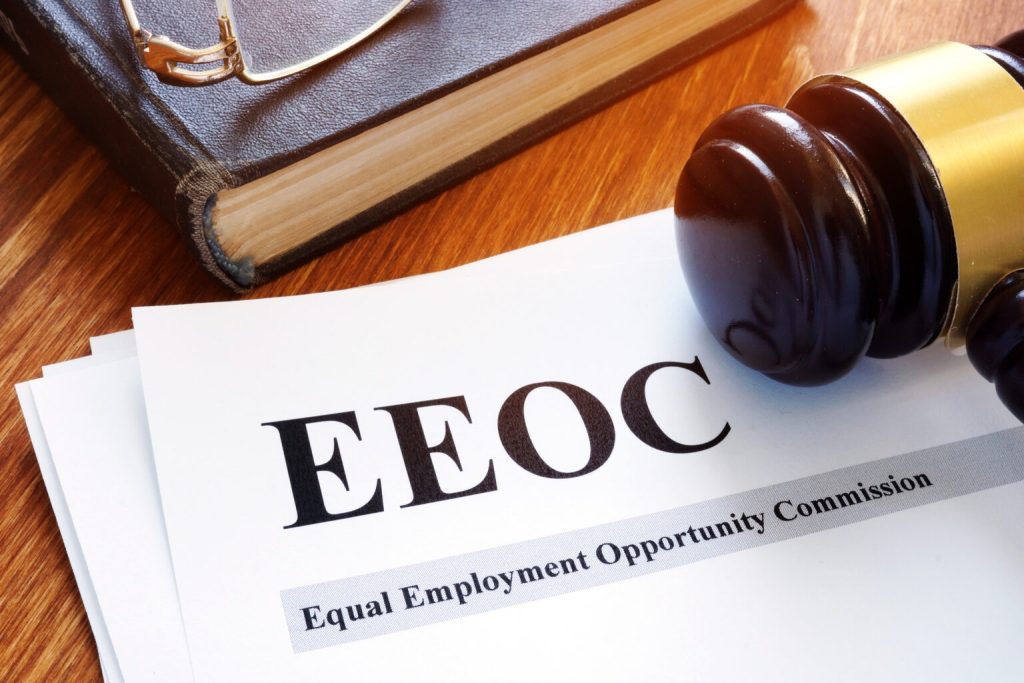
Big data discriminatory pricing led by algorithmic abuse
As an Internet arbiter that infringes the legitimate rights and interests of users unreasonably in a “reasonable” way for a long time, algorithmic discrimination implies the operation logic of maximizing the interests of algorithm operators.
Being the link between human and machine interaction, algorithm can realize the judgment and automatic decision by the machine itself through the human-coded settings and data input. However, the fairness of the algorithm does not depend on the algorithm technology itself but depends on the intentions and values reflected in the design of the algorithm, because the algorithm technology operator can decide the input data, calculation conditions and output results in the process of algorithm operation (Janssen, 2016). This also leads to the unfair result that the application of algorithm technology may kill specific groups or individuals, which constitutes the big data discriminatory pricing after the abuse of algorithms.
Through the research of Australia’s insurance price, Zofia (2022) found that insurance companies can browse your online data to price your insurance, and insurance may move further from a fair price when more personal data is available to insurance firms. The corresponding discrimination will be created not only in the pricing of insurance products but also in the situations of booking hotels, flights, and cars online. Thus, algorithmic platforms are taking advantage of users’ trust and information asymmetry to extract excess benefits. This discrimination not only infringes the rights and interests of users, but also deviates from the value principle of algorithmic fairness.
The above two cases are a good illustration of how algorithms rely to some extent on databases provided by humans, as well as the human discriminatory factors involved in the calculation process. We can see that in real life, the occurrence of algorithmic discrimination will solidify and magnify various prejudices existing in society. For some vulnerable groups, they will not only suffer prejudice in life, but also suffer secondary damage brought by algorithmic discrimination. At the same time, algorithmic discrimination also causes a huge injustice to the society, and this unfair treatment phenomenon often aggravates the social inequality problem.
The Governance of Algorithmic Discrimination is not just a Fight against Machines!
After knowing the harm of algorithmic discrimination to society, how to correct it must be a topic that we need to pay attention to. In a Forbes article, AI expert Bruno (2019) pointed out that algorithmic discrimination comes from flaws in the data, designers’ biases, and inadequacies in human-computer interaction or the algorithm itself. To eliminate discrimination, Terry Flew (2021) put forward that we can identify a mixture of approaches from the technical and legal level to policies and regulations framed in ways that are recognizable from general public policy, including media and communications policy. Governing algorithmic discrimination is not only a fight against this invisible arbiter, but also a fight against people!
Upgrade from the technical level
The upgrading and application of algorithm technology is the prerequisite of algorithm governance. This also requires that we need to start from algorithm technology and technology supervision to achieve the governance of algorithm discrimination.
On the one hand, it’s necessary for us to expand the data range and dimension that the algorithm can select from, and increase the weight of high-quality content in the algorithm recommendation. Furthermore, we can also try to reverse thinking, based on accurately describing the user portrait, the algorithm can be used to recommend some content that is not consistent with users’ preferences but may meet potential needs of users. This can not only improve the information cocoon problem brought by the algorithm, but also better meet the diversified needs of users.
On the other hand, the government should also build an algorithm supervision platform and consolidate regulatory technology to achieve digital intelligent supervision of algorithm stations. The algorithm platform ought to use the benefits of big data to accomplish self-monitoring and enhance the effectiveness of supervision with more practical and efficient algorithm technology. For example, in 2023, Colorado amended its local anti-discrimination law, which stipulates that Internet platforms must conduct annual AI fairness audits to reduce the possibility of algorithm-based discrimination through self-regulation.
Improvement of the user’s own media literacy
In addition to the self-governance of the algorithm itself and its coders, the self-restraint and management of users are also important measures to govern AI algorithms. As the algorithm will conduct data analysis according to the user’s Internet usage habits, users are required to have a clear cognition of information and a higher level of media literacy in the face of various new things on the Internet. Only when users can treat things with a rational attitude, such as properly handling the content recommended by the algorithm, and treat things with a dialectical attitude, and actively voice in the network, can they explore the operational logic behind the algorithm and understand the essence of algorithm, to reduce the vicious circle influence brought by algorithm discrimination.
Make algorithms obey the law
The iTutorGroup’s fine is not only a successful revelation of our use of legal means to govern the algorithm but also a direction for our future governance. Nowadays, many algorithms lack transparency and interpretability, so we should use legal means to require algorithm developers to improve the transparency of the process of writing algorithms, so that the public can understand the workings of algorithms and internal logic. In 2022, the Spanish government proposed an algorithmic bill to address this phenomenon, requiring companies using algorithmic systems to disclose details of the operation of those systems. As the last line of defense against algorithmic discrimination, the legal system should require governments to monitor the proper use of algorithms by investigating the algorithmic models and data of technical activities that are used in online platforms and software.
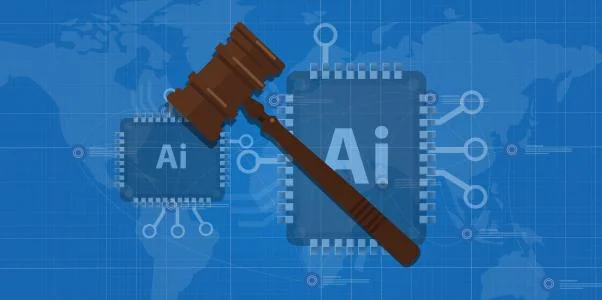
Since the personnel of algorithm design may be decentralized, it also makes the responsibility of algorithm coder difficult to be assigned and borne. Thus, it is also suggested that we need to create and improve algorithmic accountability, which requires each coder to bear the corresponding consequences and responsibilities for the algorithms they write. When Internet users suffer from algorithmic discrimination, someone can deal with the harm caused by the algorithms in a timely manner and assume the relevant responsibilities and obligations to mitigate the negative impact of algorithmic discrimination on society (Pasquale, 2015).
Conclusion
In the era of artificial intelligence, algorithms have already had an impact on many aspects of our lives, but the AI algorithms are not completely objective, neutral and fair, which also leads to the emergence of algorithmic discrimination. It discriminates against Internet users and causes a variety of social problems. For the governance of algorithm discrimination, we not only need to take certain improvement measures for the algorithm of the machine, but also need to regulate us who created the algorithm technology. The ultimate purpose of technology is to serve people, not to distinguish them. We should constantly improve its operation and constantly increase efforts to govern this arbiter hiding behind the Internet.
Reference
Arimetrics. (n.d.). What is Black box algorithm. https://www.arimetrics.com/en/digital-glossary/black-box-algorithm
Bartosz, Brożek., Michael, Furman., Marek, Jakubiec., Bartłomiej, Kucharzyk. (2023). The black box problem revisited. Real and imaginary challenges for automated legal decision making. Artificial Intelligence and Law. https://doi.org/10.1007/s10506-023-09356-9
Beatriz B. (2024, March 4). ‘Why Not Bring Weapons to School?’: How TikTok’s Algorithms Contribute to a Culture of Violence in Brazilian Schools. Global Network on Extremism&Technology. https://gnet-research.org/2024/03/04/why-not-bring-weapons-to-school-how-tiktoks-algorithms-contribute-to-a-culture-of-violence-in-brazilian-schools/
Campanha Nacional pelo Direito à Educação. (2022). O extremismo de direita entre adolescentes e jovens no Brasil: ataques às escolas e alternativas para a ação governamental. https://campanha.org.br/acervo/relatorio-ao-governo-de-transicao-o-ultraconservadorismo-e-extremismo-de-direita-entre-adolescentes-e-jovens-no-brasil-ataques-as-instituicoes-de-ensino-e-alternativas-para-a-acao-governamental/
Cofone, I. (2019, May 14). Algorithmic discrimination is an information problem. Columbia Business School Research Paper, 70 Hastings Law Journal 1389 (2019). https://ssrn.com/abstract=3387801
Flew, T. (2021). Issues of Concern. In T. Flew, Regulating platforms (pp. 79–86). Polity.
Forbes. (2019, March 27). Managing The Ethics of Algorithms. https://www.forbes.com/sites/insights-intelai/2019/03/27/managing-the-ethics-of-algorithms/?sh=350b0fdf3481
Gritsenko, D., & Wood, M. (2022). Algorithmic governance: A modes of governance approach. Regulation & Governance, 16(1), 45-62. https://doi.org/10.1111/rego.12367
Janssen, M., & Kuk, G. (2016). The challenges and limits of big data algorithms in technocratic governance. Government Information Quarterly, 33(3), 371-377. https://www.sciencedirect.com/science/article/pii/S0740624X16301599
Krista B. (2023, September 29). Google Shows Men Ads for Better Jobs. The Good Search. https://tgsus.com/diversity/google-shows-men-ads-for-better-jobs/
Lauren Ashcraft. (2023, September 29). Colorado passes law to prevent AI-generated discrimination in life insurance. EMARKETER. https://www.emarketer.com/content/colorado-passes-law-regulating-ai-in-insurance
Nachbar, T. B. (2020). Algorithmic fairness, algorithmic discrimination. Fla. St. UL Rev., 48, 509. https://heinonline.org/HOL/Page?handle=hein.journals/flsulr48&div=15&g_sent=1&casa_token=&collection=journals
Noble, Safiya U. (2018). A society, searching. In Algorithms of Oppression: How search engines reinforce racism. New York: New York University. pp. 15-63. https://www.jstor.org/stable/j.ctt1pwt9w5.5
Pasquale, Frank (2015). ‘The Need to Know’, in The Black Box Society: the secret algorithms that control money and information. Cambridge: Harvard University Press, pp. 1-18. https://raley.english.ucsb.edu/wp-content/Engl800/Pasquale-blackbox.pdf
Sheridan W. & Hilke S. (2021, June 23). LinkedIn’s job-matching AI was biased. The company’s solution? More AI. MIT Technology Review. https://www.technologyreview.com/2021/06/23/1026825/linkedin-ai-bias-ziprecruiter-monster-artificial-intelligence
U.S. Equal Employment Opportunity Commission. (2022, May 5). EEOC Sues iTutorGroup for Age Discrimination. https://www.eeoc.gov/newsroom/eeoc-sues-itutorgroup-age-discrimination
U.S. Equal Employment Opportunity Commission. (2023, November 9). iTutorGroup to Pay $365,000 to Settle EEOC Discriminatory Hiring Suit. https://www.eeoc.gov/newsroom/itutorgroup-pay-365000-settle-eeoc-discriminatory-hiring-suit
Yuan, T. (2023). User Portrait Based on Artificial Intelligence. In J. C. Hung, N. Y. Yen, & J.-W. Chang (Eds.), Frontier Computing (pp. 359–366). Springer Nature Singapore. https://doi.org/10.1007/978-981-99-1428-9_44
Zofia B. (2022, June 20). Insurance firms can skim your online data to price your insurance — and there’s little in the law to stop this. Australian Privacy Foundation https://privacy.org.au/2022/06/20/insurance-firms-can-skim-your-online-data-to-price-your-insurance-and-theres-little-in-the-law-to-stop-this/
Be the first to comment