With the rapid development of modern technology, Artificial Intelligence (AI) has become one of the key factors driving the growth of technology and society. Facial Recognition Technology (FRT) is an important application area of AI that has made significant progress in recent years. FRT is an AI technology that uses machine learning and deep learning to identify face features from face images or video frames. The steps of FRT involve first recognizing faces in larger images or scenes and distinguishing them from the surrounding environment. Then, it analyses the facial features of the identified person, such as the shape of the eyes, mouth, nose, and other parts of the face. Finally, a facial template is created by extracting these features, and a matching algorithm is used to compare it to a database of known faces, ultimately achieving face recognition (Innovatrics, 2023). In our daily lives, we use FRT widely, from unlocking mobile phones to face swipe payment to all kinds of “just swipe your face” services. However, there are some problems and challenges associated with the convenience that FRT brings to our lives. The blog uses FRT in law enforcement as a case study to focus on the issues of bias and risk in its application and to highlight the challenges of technology governance in the digital age.
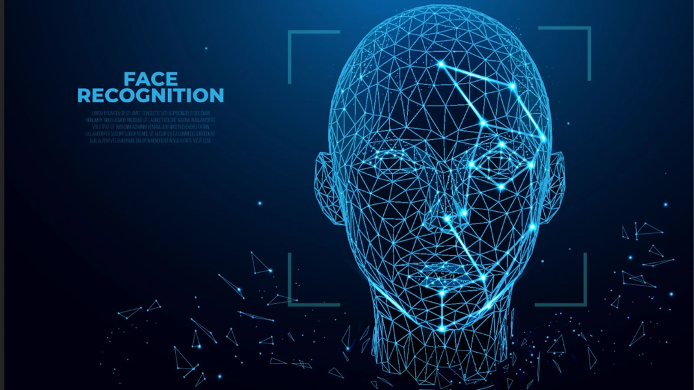
Facial recognition technology uses AI algorithms to analyze facial features
FRT in law enforcement: Wrongful arrests of innocent black man
FRT has a wide-ranging role in law enforcement, where it can be used to compare a suspect’s facial image with a database of suspects to help confirm identity and track the suspect’s movements and location. For example, when investigating fraud, the police can compare a suspect’s photo with the face in the ATM surveillance video to track down the suspect. However, while FRT can bring convenience to public safety, it also has limitations. On the second day of Thanksgiving in 2022, Randal Quran Reid (a black man) was wrongfully arrested by police outside of Atlanta for allegedly stealing $15,000 from a credit card in Louisiana (Negussie, 2023). According to Reid’s lawsuit, police used the FRT to mistake Reid for a suspect and arrest him. But the truth is that Reid had never been to Louisiana and had not done the things he was accused of. After six days in jail, Reid was acquitted after his lawyer provided several photographs of Reid to prove he was not a suspect. Reid concluded that his experience had made him lose faith in the justice system because innocent people can be imprisoned for things they never did.
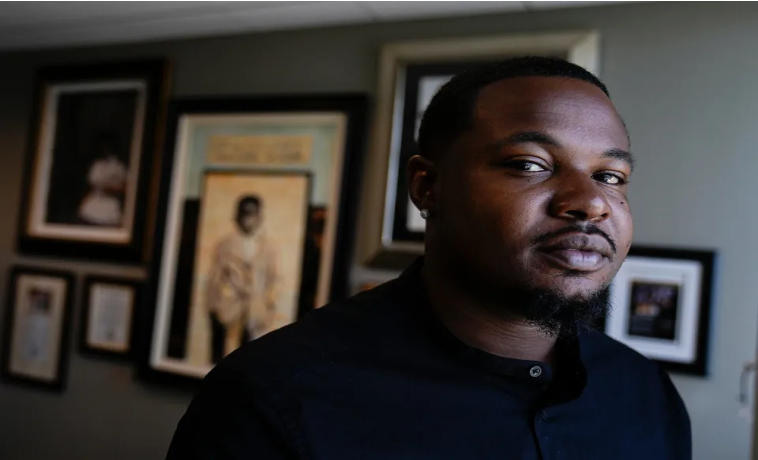
Portrait of Randal Quran Reid at the Atlanta Lawyer’s Office
Biased algorithms: FRT’s racial bias
The example of Reid exemplifies the severe problems with AI technology in law enforcement. FRT can help the police find suspects, but if we look at the whole process of developing, deploying, and using technology in FRT, we can see its potential impacts. The National Institute of Standards and Technology (2020) conducted a study to examine differences in the accuracy of FRT in identifying people of different genders, ages, and ethnic backgrounds. The results show that Asian, African American (especially African American women), and Native American groups have higher false alarm rates for facial images compared to whites. The study shows that the algorithm used in the core of the system, the application that uses the algorithm, and the data entered are all factors that affect FRT results. In addition, the Pew Research Center (2022) examined Americans’ views on law enforcement’s use of FRT, which showed that 66% believe that FRT is used to monitor Black and Hispanic communities more often than other communities, and 53% believe that FRT will lead to frequent wrongful arrests if it is used extensively by the police. These results highlight not only the low accuracy of the FRT in identifying people of color but also the limitations of the FRT in terms of fairness.
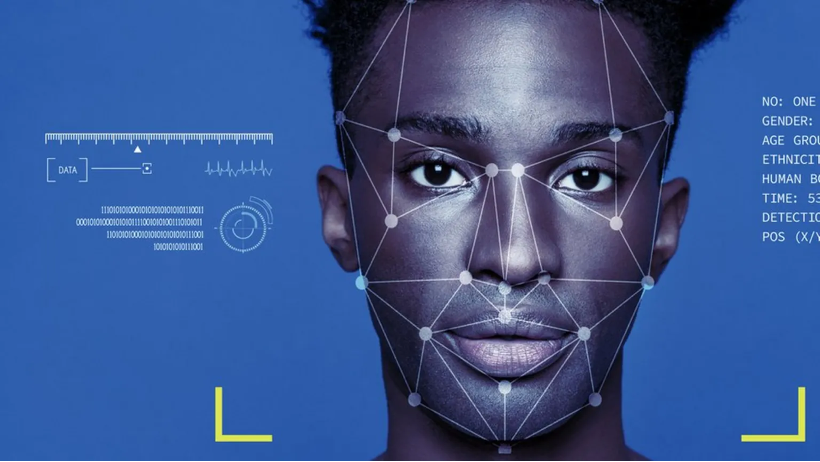
Police facial recognition system less accurate when analyzing non-white faces
The bias in gender and ethnicity identification in FRT reflects bias in the dataset and the algorithm. It also demonstrates the problem of ‘garbage in, garbage out.’ That is, there is a lack of diversity in the datasets used to train the FRT (Sampath, 2021). The lack of diverse perspectives in the companies developing FRT technology leads to bias problems in practical applications. Furthermore, Gentzel (2021) states that using biased FRT is incompatible with classical liberalism because it violates the principle that individuals should be treated equally under the law. This argument is correct in that the use of biased FRT by law enforcement can lead to the misperception that certain ethnic groups are being targeted, thus violating individual rights and the principle of equality for all. This result also exacerbates the structural inequality that persists in tech companies, which directly impacts social and political bias.
Is FRT neutral?
FRT itself as a technology is neutral, it can be used as a tool to help law enforcement quickly match suspects in a huge amount of facial data. In this sense, it is the same as the duty of the police to keep the peace in the community. However, it can be politicized based on developer bias, data influence, and law enforcement’s use of FRT. Like the example of Robert Moses’ construction of overpasses in the 20th century, Moses built the overpasses exceptionally low, thereby preventing poor people and people of color who could only travel by bus from accessing Jones Beach (Bloomberg, 2017). The construction of the overpasses highlights Moses’ use of seemingly neutral urban infrastructure to fulfill his racial segregationist political intentions. This example shows that neutral technology becomes non-neutral through human influence in development and use. Similarly, Reid’s case illustrates that FRT is not a neutral technology in law enforcement and that its algorithms and data bias result in minorities being more likely to be identified as suspects, thus exacerbating racial discrimination in society. Crawford (2021) stated that AI is not autonomous and rational and that AI models need to be highly dependent on large datasets for training to make decisions and, in the process, cannot avoid the ingrained biases of humans. Therefore, AI is dependent on political and social structures, and law enforcement agencies should not only focus on the benefits that FRT can bring but also be aware of the social impacts that it may have when used.
Ethical issues arising from technological automation
Reid’s case also illustrates the over-reliance of the police on the FRT. The police attempted to replace and automate crime-solving with the FRT. One of the significant impacts of AI design is the increase in automation and efficiency, an automated process that significantly reduces manual and labor costs. However, the result also affects people’s over-reliance on AI technology; in the long term, people may lose the ability to think independently. Andrejevic (2019) states that automated systems suffer from a “post-social” bias, which attempts to separate human social decision-making from machine data processing, allowing machine processes to replace human judgment. FRT systems attempt to simplify crime governance through automated data analysis, but ethical issues need to be considered during the process. Previously, Australian Privacy Commissioner Angelene Falk found that Clearview AI’s facial recognition tool breached the Australian Privacy Act 1988 (Lomas, 2021). Clearview AI has seriously breached the public’s right to privacy by collecting Australians’ biometric information online without their permission to train FRT algorithms and selling it to law enforcement and other organizations. This illustrates the data ethics issues facing FRT in law enforcement and highlights the data colonialism brought about by algorithms.
Couldry and Mejias (2019) argued that algorithms are critical to the implementation of ‘data colonialism,’ which is a new form of exploitation. The Clearview AI incident reflects the concept of data colonization. Australians are being unknowingly colonized by data that does not provide any personal benefit to the user but is used for commercial gain. Therefore, personal data has become a new type of raw material as important as capital and labor. The big data revolution is capturing and analyzing huge amounts of personal data to transform 21st-century economies and societies (Flew, 2019, p. 79). Clearview AI is just one example, and many more companies may be covertly collecting our facial information for commercial gain. Driven by interests, data infringement is likely to become increasingly common. In such circumstances, strict regulation and governance mechanisms are essential in protecting individuals’ personal data rights and interests.
Governance of FRT in law enforcement
The governance of FRT will vary from country to country depending on the local legal environment and social values. For example, the EU’s General Data Protection Regulation follows the principles of necessity and proportionality, which means that it must ensure that the processing of data is lawful and reasonable even without the public’s knowledge, while Chinese law emphasizes the need for citizens to give prior consent to the processing of data and the purpose of the data (Bu, 2021). However, their ultimate purpose is to protect the privacy and data rights of individuals. Hill et al. (2022) stated that the key to avoiding the risk of the FRT in law enforcement is establishing a rational governance framework and that public views need to be incorporated into the technology policy in this process. This means the public is not a spectator but a participant in constructing the policy. The public needs to be involved in developing the FRT, the collection and use of data, the evidence of the effectiveness of the FRT, and the plans for ongoing regulation of the FRT. Ireland’s Citizens’ Assembly provides a good direction for the realization of a policy of public participation through the random selection of a representative group of people (these populations can reflect the age, gender, and social class of the Irish population) to discuss the most pressing issues facing Ireland. For example, Ireland’s Citizens’ Assembly legalized abortion in Ireland (Involve, 2018). This type of innovative approach can also be used in the governance of the FRT, where public views are incorporated into decision-making on the FRT through mechanisms such as random sampling, listening to expert knowledge, and policy advice.
Four key principles for developing FRT governance
Lyria Bennett Moses (2023) suggests that we need to build constraints into the laws that authorize the use of AI systems. This also applies to placing in the governance of FRT, and she makes the following four recommendations.
i. Legal constraints built into authorizing legislation
FRT’s testing and evaluation process needs to be open and transparent, and there should be clear laws and regulations regarding FRT from the beginning. For example, the regulatory authorities can take the first step to regulate the collection, preservation, and utilization of face data, thus preventing face data from being illegally sold at the source.
ii. Protection for privacy and autonomy
The use of FRT by law enforcement or other agencies requires the protection of public privacy and strict control over data collection and use.
iii. Detailed standards
In the practical application of FRT, individual organizations should develop achievable policy standards. This includes data privacy protection, algorithm transparency, the purpose of using AI, etc.
iv. Educated citizenry
The public needs to be actively involved in the governance of FRT and raise awareness of FRT and its potential harms and risks. And the public needs to protect its values to prevent flawed systems from being introduced by the government.
The four principles proposed by Moses provide constructive assistance to government agencies or technology companies in structuring FRT governance. This is critical to mitigating the potential harm caused by FRT and upholding the rights of citizens.
Conclusion
The use of FRT in law enforcement is a “wake-up call” for AI in governance. AI technology is a “double-edged sword” which on the one hand, brings technological changes to improve people’s quality of life and social well-being, but on the other hand, it also raises a series of risks and challenges such as social bias, personal data rights, and ethical issues. To deal with these challenges, governments and tech companies are required to develop these technologies with stricter laws and regulations and in a publicly responsible manner. And the public should be alerted to using these technologies to avoid being exploited maliciously. AI technology can serve life better if it operates correctly under legal and ethical constraints.
Reference
Andrejevic, M. (2019). Automated Media (1st ed.). Routledge. https://doi.org/10.4324/9780429242595
Bloomberg. (2017). Robert Moses and His Racist Parkway, Explained.
https://www.bloomberg.com/news/articles/2017-07-09/robert-moses-and-his-racist-parkway-explained
Bu, Q. (2021). The global governance on automated facial recognition (AFR): ethical and legal opportunities and privacy challenges. International Cybersecurity Law Review (Print), 2(1), 113–145. https://doi.org/10.1365/s43439-021-00022-x
Crawford, K. (2021). Atlas of AI: Power, Politics, and the Planetary Costs of Artificial Intelligence. New Haven: Yale University Press. https://doi.org/10.12987/9780300252392
Couldry, N., & Mejias, U. A. (2019). Data colonialism: Rethinking big data’s relation to the contemporary subject. Television & New Media, 20(4), 336-349.
Flew, T. (2021). Regulating Platforms. Polity.
https://bookshelf.vitalsource.com/books/9781509537099
Gentzel M. (2021). Biased Face Recognition Technology Used by Government: A Problem for Liberal Democracy. Philosophy & technology, 34(4), 1639–1663. https://doi.org/10.1007/s13347-021-00478-z
Hill, D., O’Connor, C. D., & Slane, A. (2022). Police use of facial recognition technology: The potential for engaging the public through co-constructed policy-making. International Journal of Police Science & Management, 24(3), 325-335. https://doi.org/10.1177/14613557221089558
Innovatrics. (2023). Facial Recognition Technology – Innovatrics – How it Works. https://www.innovatrics.com/facial-recognition-technology/
Lomas, N. (2021). Clearview AI told it broke Australia’s privacy law, ordered to delete data. TechCrunch. https://techcrunch.com/2021/11/03/clearview-ai-australia-privacy-breach/
Negussie, T. (2023). Lawsuit: Man claims he was improperly arrested because of misuse of facial recognition technology. ABC News. https://abcnews.go.com/US/lawsuit-man-claims-falsely-arrested-misuse-facial-recognition/story?id=103687845
NIST study Evaluates effects of race, age, sex on face recognition software | NIST. (2020). NIST. https://www.nist.gov/news-events/news/2019/12/nist-study-evaluates-effects-race-age-sex-face-recognition-software
Pew Research Center. (2022). Public views of police use of facial recognition technology. Pew Research Center: Internet, Science & Tech. https://www.pewresearch.org/internet/2022/03/17/public-more-likely-to-see-facial-recognition-use-by-police-as-good-rather-than-bad-for-society/
Sampath, P. G. (2021). Governing artificial intelligence in an age of inequality. Global Policy, 12(S6), 21–31. https://doi.org/10.1111/1758-5899.12940
The Citizens’ Assembly behind the Irish Abortion Referendum | Involve. (2018). Involve. https://www.involve.org.uk/news-opinion/opinion/citizens-assembly-behind-irish-abortion-referendum
TEDx Talks. (2023). Should we worry about AI and algorithms in government? | Lyria Bennett Moses | TEDxSydneySalon [Video]. YouTube. https://www.youtube.com/watch?v=y6FDBDGNYFc
Be the first to comment