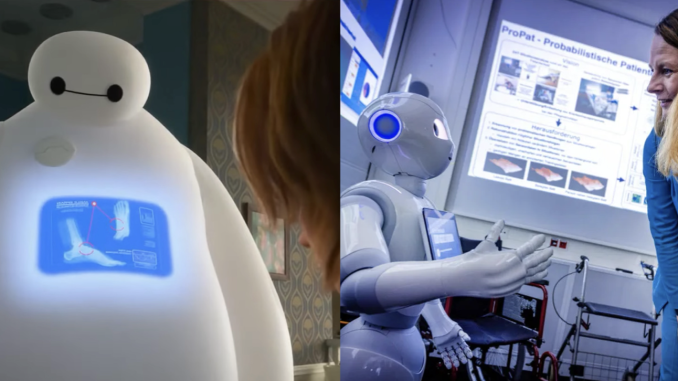
“Hello. I am Baymax, your personal healthcare companion”. In the Disney animation “Baymax!”, the AI robot Baymax was invented to provide healthcare services for patients (Disney+, 2022). Once Baymax hears a sound of distress from someone, he will be activated automatically (Hall, 2022). Then, by conducting a quick scan, Baymax can detect symptoms and treat diseases specifically (Hall, 2022). As technology has been developing rapidly, having AI in healthcare as the animation showed is no longer a fantasy. This year, eight AI-powered robots, which were invented to provide healthcare services to elderly patients, have successfully passed the testing phase with patients (Gordon, 2024). Additionally, some hospitals in Japan are utilising AI in almost every stage from entering the hospitals to the treatment, such as AI bacterial identification and AI robot dogs which can assist in child medical diagnosis (NHK WORLD-JAPAN, 2023).
There is no doubt that artificial intelligence (AI) has become an irreplaceable part of our society and life, and it has replaced human workers in some industries. Therefore, it is not surprising that AI is widely used in healthcare institutions to assist decision-making and improve the quality and efficiency of healthcare services (Lee & Yoon, 2021). Then, is it our future to have AI doctors rather than human doctors? Considering the diagnosis errors caused by AI and the breach of patients’ private information, this article argues that AI can not replace human doctors and it requires governance to make AI become a better healthcare assistant.
The Concerns Surrounding the Implementation of AI in Healthcare
The world’s first medical AI, Watson for Oncology of IBM, was launched commercially in 2015 to recommend appropriate chemotherapy regimens for cancer patients (Zhou et al., 2021). Since then, more and more AI technologies have been used in the healthcare industry to assist in diagnosis, nursing and management. For instance, scientists at CSIRO’s Australian e-Health Research Centre are using artificial intelligence to predict health risks, generate medical images and develop precision medicine to enable clinicians to be more efficient (CSIRO, 2023).
However, when we benefit from implementing AI in healthcare and look forward to its bright future, the concerns of diagnosis errors caused by AI and the breach of patients’ private information should not be ignored.
• Diagnosis Errors
As Stekelenburg (2024) argued, the accuracy of AI means the difference between life and death in healthcare. Therefore, the issue of diagnosis errors is the first and foremost concern surrounding the implementation of AI in healthcare.
Indeed, several documents and studies have substantiated the validity of the concern. For example, internal IBM documents showed that the Watson AI system recommended multiple unsafe and incorrect cancer treatment advice (Ross & Swetlitz, 2018). Additionally, although the past years have witnessed the great development of technology, AI diagnostic errors still occur. For instance, in 2022, a study that aimed to assess the incidence of diagnostic errors in an AI-driven medical history–taking system, found that the rate of diagnostic errors made by the AI system was 11% (Kawamura et al., 2022). Why do diagnostic errors arise in AI?
Crawford (2021) indicated that the AI system is not autonomous, rational, or able to discern things by itself. In other words, AI entirely depends on the training datasets and rules which are provided by humans. Therefore, it is inevitable that AI may have flaws in data learning and make errors in medical diagnosis.
On the one hand, insufficient training datasets can lead to AI diagnosis errors. As Haseltine (2023) argued, like the large language model of AI needs large amounts of data from search engines to work, the medical AI system also requires mountainous health data to diagnose correctly. In other words, insufficient data in the training dataset will make AI analyse diseases one-sidedly and inaccurately. Fragmented medical histories and poor data management of the dataset are the main contributors to data insufficiency (Haseltine, 2023). For example, a study found that AI has difficulty diagnosing specific patients because of their medical history and specific symptom data deficiency(Kawamura et al., 2022).
Additionally, a study about breast cancer screening AI found that the sensitivities and specificities of the algorithm are lower than radiologists’ because of the unrepresentative dataset (Marinovich et al., 2023). In summary, inadequate training datasets can decrease the accuracy and specificity of AI healthcare systems, which will result in diagnostic errors and patient harm.
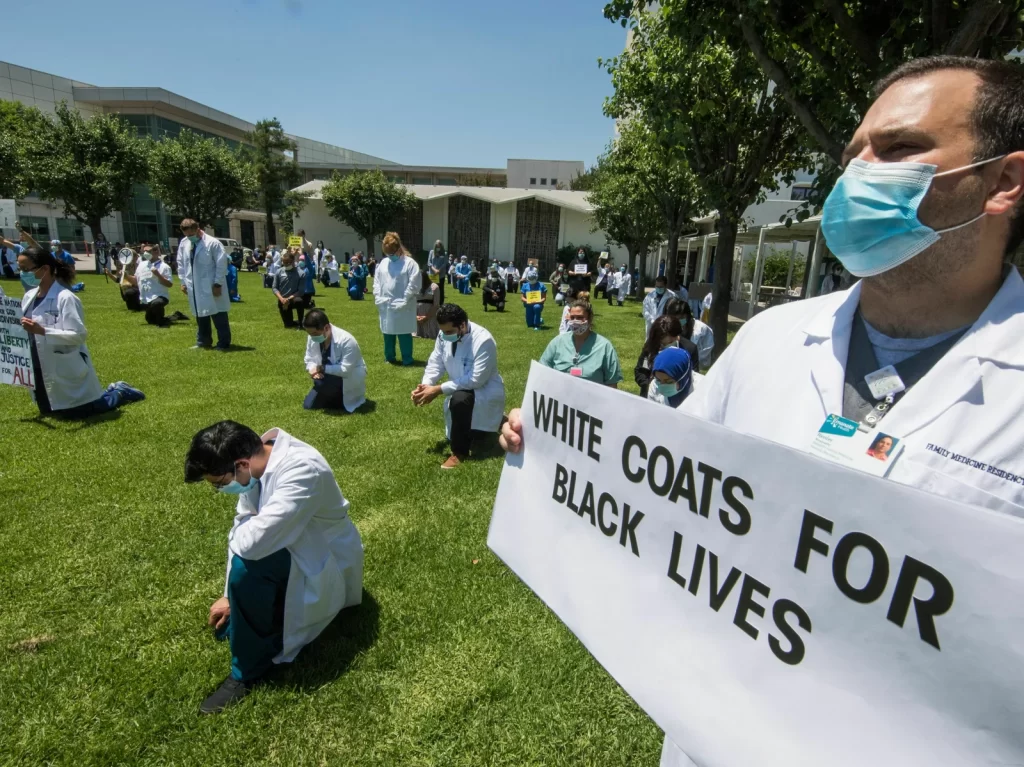
On the other hand, the bias and inequality in training datasets will also lead to healthcare AI making diagnosis errors. As Barrowman (2018) indicated, data is never raw because even the initial data collection involves intentions, assumptions, and choices. In other words, training datasets have been pre-processed and may collect biased and unequal data in this process. Therefore, AI may make diagnosis errors with biases and inequalities because they exist in the training dataset. For instance, one research shows that clinicians sometimes make different treatments for white patients and patients of colour, and when these treatment data are utilised in training healthcare AI, it will result in unequal diagnosis for patients of colour (Levi & Gorenstein, 2023).
• The Breach of Private Information
Another concern surrounding the implementation of AI in healthcare is the breach of the patient’s private information. The breach of private information may arise in both data collection and data sharing.
Firstly, since AI is based on huge datasets, privacy concerns arise in terms of data collection (Lee & Yoon, 2021). As Crawford (2021) argued, AI attempts to make its own atlases. In other words, AI systems in healthcare not only collect professional treatment and knowledge of clinicians and medical scientists to learn from them, but also collect personal information and symptoms of patients to expand its dataset. However, the latter collection always be conducted without the consent of patients. In other words, it means that patients can not be aware of their personal information being collected, which increases the risk of privacy infringement. Additionally, medical information collected by AI can be hacked into and utilized for malicious purposes (Farhud & Zokaei, 2021), which makes patients worry about privacy leakage as well.
Secondly, healthcare database interworking makes private information being shared without consent from patients possible. Moreover, in the Internet era, database interworking sometimes will not be confined to the health industry, which means that the data collected from healthcare might be shared with other industries to train their database. For example, thousands of private medical photos were found in the LAION image set to train generative artificial intelligence (Edwards, 2022). Although at first these photos were collected during healthcare to make accurate diagnoses and complete specific medical histories, finally they were used in other industries for different usages without any permission. In short, considering that medical photos collected by AI involve not only personal medical records but also the facial information of patients, the risk of privacy leakage surrounding the implementation of AI in healthcare should be paid more attention.
Governance
Even though, the positive aspects surrounding the implementation of AI in healthcare can not be ignored. For one thing, it can improve medical efficiency significantly. The doctors at the National Center for Child Health and Development are using AI to identify bacterial types in less time (NHK WORLD-JAPAN, 2023). It always takes about three days to culture enough bacteria and then the identification can be conducted, but with the AI system, it will only take about ten seconds to get the results of the bacterial identification (NHK WORLD-JAPAN, 2023).
For another, it can provide patients with a more comfortable treatment experience. For example, AI-powered robot dogs in the National Center for Child Health and Development can learn words and actions from child patients and make suitable responses to each child, which can make children feel secure and be willing to share their feelings of pain that are hard to tell doctors and parents with robotic dogs (NHK WORLD-JAPAN, 2023). In short, considering both the positive and negative sides of applying AI in healthcare, it is necessary to conduct governance to make AI a better assistant for humans in healthcare.
• Governance of AI Accuracy
Pasquale (2015) argued that information is power, and the information collected by institutions can lead to vitally important consequences. In other words, the breach of private information should be the priority for governance. However, considering that the implementation of AI in healthcare has a more significant impact on human life safety — which can be the difference between life and death (Stekelenburg, 2024), the governance of AI accuracy should be paid more attention.
Firstly, doctors should review the results generated by AI before treatment. As the cases mentioned above, numerous studies have proved that AI-generated diagnoses are not perfect. Therefore, the review of doctors can be a double assurance of the diagnosis accuracy. For example, although the Keio University Hospital utilises AI-powered robots to dispense drugs in the Department of Pharmacy, pharmacists will double-check the medicine to ensure safety (NHK WORLD-JAPAN, 2023).
Secondly, the diversity of medical data should be improved. The main reason for the diagnosis errors made by AI is the limited quality and quantity of data. Therefore, the datasets of AI in healthcare should be checked and updated regularly to ensure that the datasets represent the different patient groups, diseases and medical facilities as much as possible (Evans & Snead, 2023). Additionally, cases, data and technology sharing between hospitals are also necessary to improve the diversity of data.
• Governance of Privacy Breach
Nevertheless, it does not mean that the governance of privacy breach is inessential. Especially when diversifying the dataset of AI in healthcare with more data and information, the issue of privacy needs to be taken more seriously. To govern the breach of private information, as Pasquale (2015) argued, mass surveillance is necessary.
First, patients should be able to surveil the details of information collection and sharing. Specifically, patients should be given the right to give and revoke personal information depending on the context in which the information is used (Australia’s National Science Agency, 2024).
Second, to protect the personal information of each patient, establishing and improving the legal framework of information access and sharing for AI in healthcare is necessary (Lee & Yoon, 2021). For instance, the Singapore government has developed the Artificial Intelligence in Healthcare Guidelines (AIHGle) to provide AI developers and implementers with good practices and complement the regulations for AI medical devices (Ministry of Health, 2021).
Last but not least, developing governance by technologies can improve the efficiency of surveillance. For example, scientists recently created a new system for inherently privacy-preserving vision which can obscure the visual information before it is digitised (Taras et al., 2024). By applying this system to AI in healthcare, the private information of patients captured by AI cameras will be protected better. Additionally, Just and Latzer (2017) argued that governance by algorithms is essentially an example of governance by technology. By assigning relevance to selected information, algorithms can identify the required message more quickly and comprehensively (Just & Latzer, 2017), which will improve the efficiency of surveillance AI in healthcare and protect the private information of patients.
Conclusion
Recent years have witnessed AI playing a significant role in our society, which has replaced human workers in many industries. However, AI in healthcare will have no chance to replace human doctors. For one thing, due to insufficient data, bias and inequality in training datasets, AI in healthcare is not perfect now and may cause diagnosis errors. For another, concerns surrounding the breach of the patient’s private information arise in terms of AI data collection and sharing. Even though, it is undeniable that AI in healthcare can make great contributions to improving medical efficiency and providing better treatment experience. Therefore, this article argues that AI can be an assistant rather than a substitute for the human doctor, and it is necessary to conduct governance to make AI a better healthcare assistant. First, for the governance of AI accuracy, doctors should review the results generated by AI before treatment, and the diversity of medical data should be improved. Second, for the governance of privacy breach, mass surveillance through patients, laws and advanced technologies is necessary. By governing the concerns, AI will be a valuable assistant in healthcare to improve healthcare services and protect patient privacy.
References
Australia’s National Science Agency. (2024). AI trends for healthcare. https://aehrc.csiro.au/wp-content/uploads/2024/03/AI-Trends-for-Healthcare.pdf
Barrowman, N. (2018). Why Data Is Never Raw. The New Atlantis. https://www.thenewatlantis.com/publications/why-data-is-never-raw
Crawford, K. (2021). Introduction. The Atlas of AI: Power, Politics, and the Planetary Costs of Artificial Intelligence (pp. 1-21). Yale University Press. https://doi.org/10.2307/j.ctv1ghv45t.3
CSIRO. (2023, September 1). How can Artificial Intelligence improve healthcare?. [Video]. Youtube. https://www.youtube.com/watch?v=sj_tFotOToo
Disney+. (2022). Baymax!. https://www.disneyplus.com/en-au/series/baymax/1D141qnxDHLI
Edwards, B. (2022, September 22). Artist finds private medical record photos in popular AI training data set. Ars Technica. https://arstechnica.com/information-technology/2022/09/artist-finds-private-medical-record-photos-in-popular-ai-training-data-set/
Evans, H., & Snead, D. (2023). Why do errors arise in artificial intelligence diagnostic tools in histopathology and how can we minimize them?. Histopathology, 84 (2), 279-287. https://doi.org/10.1111/his.15071
Farhud, D. D., & Zokaei, S. (2021). Ethical Issues of Artificial Intelligence in Medicine and Healthcare. Iranian Journal of Public Health, 50(11), i–v. https://doi.org/10.18502/ijph.v50i11.7600
Gordon, A. (2024, January 31). Robots Created to Help Patients in Hospitals Pass Testing Phase. TIME. https://time.com/6590440/robots-hospital-patient-testing-phase-ai-assistance/
Hall, D. (Creator). (2022). Baymax!. [Animation episode series]. Disney+.
Haseltine, W. A. (2023, September 29). The Synergy Of Artificial Intelligence And Robots In Medical Practice. Forbes. https://www.forbes.com/sites/williamhaseltine/2023/09/29/the-synergy-of-artificial-intelligence-and-robots-in-medical-practice/?sh=7ae737ad5935
Just, N., & Latzer, M. (2017). Governance by algorithms: reality construction by algorithmic selection on the Internet. Media, Culture & Society, 39(2), 238-258. https://doi.org/10.1177/0163443716643157
Kawamura, R., Harada, Y., Sugimoto, S., Nagase, Y., Katsukura, S., & Shimizu, T. (2022). Incidence of Diagnostic Errors Among Unexpectedly Hospitalized Patients Using an Automated Medical History-Taking System With a Differential Diagnosis Generator: Retrospective Observational Study. JMIR medical informatics, 10(1), e35225. https://doi.org/10.2196/35225
Lee, D., & Yoon, S. N. (2021). Application of Artificial Intelligence-Based Technologies in the Healthcare Industry: Opportunities and Challenges. International Journal of Environmental Research and Public Health, 18(1). https://doi.org/10.3390/ijerph18010271
Levi, R., & Gorenstein, D. (2023, June 6). AI in medicine needs to be carefully deployed to counter bias – and not entrench it. NPR. https://www.npr.org/sections/health-shots/2023/06/06/1180314219/artificial-intelligence-racial-bias-health-care
Marinovich, M. L., Wylie, E., Lotter, W., Lund, H., Waddell, A., Madeley, C., Pereira, G., & Houssami, N. (2023). Artificial intelligence (AI) for breast cancer screening: BreastScreen population-based cohort study of cancer detection. EBioMedicine, 90, 104498. https://doi.org/10.1016/j.ebiom.2023.104498
Ministry of Health. (2021, October). Artificial Intelligence in Healthcare Guidelines (AIHGle). https://www.moh.gov.sg/docs/librariesprovider5/eguides/1-0-artificial-in-healthcare-guidelines-(aihgle)_publishedoct21.pdf
NHK WORLD-JAPAN. (2023, September 3). AI Hospitals: A Step Towards the Future – Science View. [Video]. Youtube. https://youtu.be/gfEspHh6xdw?si=hxv2h3D_srlR9Zap
Pasquale, F. (2015). INTRODUCTION: THE NEED TO KNOW. In The Black Box Society: The Secret Algorithms That Control Money and Information (pp. 1-18). Harvard University Press. https://www.jstor.org/stable/j.ctt13x0hch.3
Pasquale, F. (2015). TOWARD AN INTELLIGIBLE SOCIETY. In The Black Box Society: The Secret Algorithms That Control Money and Information (pp. 189-218). Harvard University Press. https://www.jstor.org/stable/j.ctt13x0hch.8
Ross, C., & Swetlitz, I. (2018, July 25). IBM’s Watson supercomputer recommended ‘unsafe and incorrect’ cancer treatments, internal documents show. STAT. https://www.statnews.com/2018/07/25/ibm-watson-recommended-unsafe-incorrect-treatments/#:~:text=Internal%20IBM%20documents%20show,and%20physicians%20around%20the%20world
Stekelenburg, N. (2024, April 4). AI for healthcare is as easy as ABC. CSIRO. https://www.csiro.au/en/news/All/Articles/2024/April/AI-healthcare-trends
Taras, A.K., Sünderhauf, N., Corke, P., & Dansereau, D.G. (2024). Inherently privacy-preserving vision for trustworthy autonomous systems: Needs and solutions. Journal of Responsible Technology, 17. https://doi.org/10.1016/j.jrt.2024.100079
Zhou, J., Zeng, Z., & Li, L. (2021). A meta-analysis of Watson for Oncology in clinical application. Scientific Reports, 11. https://doi.org/10.1038/s41598-021-84973-5
Images
Büttner, J. (2024). A system programmed by the Rostock scientists for the care of stroke patients in Germany. In Robots Created to Help Patients in Hospitals Pass Testing Phase. https://time.com/6590440/robots-hospital-patient-testing-phase-ai-assistance/
Ralston, M. (2023). Healthcare professionals at a hospital in California protest racial injustice after the murder of George Floyd. In AI in medicine needs to be carefully deployed to counter bias – and not entrench it. NPR. https://www.npr.org/sections/health-shots/2023/06/06/1180314219/artificial-intelligence-racial-bias-health-care
Wellins, D. (Director). (2022). Cass. In Baymax! [Animation episode series]. Disney+.
Be the first to comment