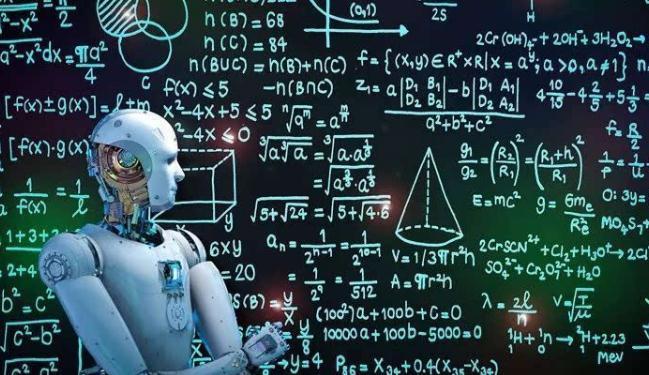
In the digital era, the network has become one of the main channels for people to obtain information and communicate. With the rapid development of technology, algorithms have gradually become an indispensable part of the network. Algorithms are playing more and more important roles in both personal life and corporate and governmental fields and are gradually influencing human decision-making and life. However, the application of algorithms brings convenience to people’s daily lives and at the same time brings many challenges. As the application of algorithms becomes more and more popular, its governance and regulation should also receive extensive attention. In this paper, we will explore the impact of algorithms on people’s lives and decision-making, analyze the pros and cons, and propose corresponding solutions.
What are Algorithms?
An algorithm is a set of instructions or commands that a computer can use to solve a task (Perez et al., 2021), with tools to solve a variety of tasks, such as search, data processing, machine learning, and so on. With the continuous development of modern technology, early simple algorithms have gradually evolved into complex deep-learning tools and become the core tool for solving various problems.
How Algorithms Influence Human Decision Making?
Algorithms have long been applied to all areas of people’s daily lives, from search engines to social media and e-commerce without showing their importance. It not only provides people with smarter and more convenient services but also gradually affects human decision-making.
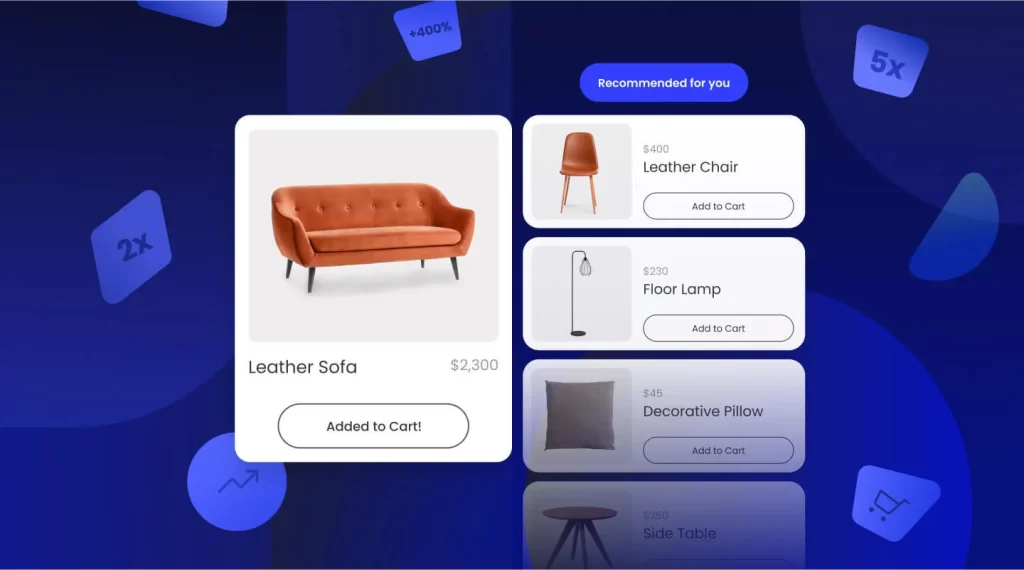
Personalized Recommendations
In today’s digital age, personalized recommendations are ubiquitous. In today’s digital age, personalized recommendations are ubiquitous and have become a standard feature on many online platforms. With the rise of online shopping, personalized recommendations have been widely used on major e-commerce platforms. This kind of personalized recommendation is subtly changing people’s shopping habits and decisions, and even shaping people’s consumption concepts.
As one of the world’s largest e-commerce platforms, Amazon also applies personalized recommendation systems to online sales. First, Amazon’s personalized recommendation system will record and analyze the user’s browsing history and search history to profile the user. For example, if the target user is young women, more products such as beauty products and clothing will be recommended to them. Secondly, Amazon’s personalized recommendation platform can also analyze gifts that users may be interested in in the future through the user’s purchase history and the number of clicks on different products, improving the accuracy of recommendations. For example, if a young male user has frequently searched for kitchenware and household products recently, the personalized recommendation system will predict that he is about to get married. In the future, product recommendations for this customer will gradually shift from game consoles to daily necessities.
Personalized recommendation accurately analyzes and reflects the user’s consumption preferences, allowing people to find interesting content and products more quickly, thus reducing the time in the browsing process, and improving the efficiency of the shopping experience. Moreover, personalized recommendations can also affect people’s shopping behavior to a certain extent, which directly influences people’s shopping decisions and indirectly improves the sales of e-commerce platforms. Amazon’s personalized search ranking will recommend products that generate higher profits (Zhou B et al,2023). Guided by personalized recommendations, users tend to buy items that are not on their shopping lists, thus completing more shopping.
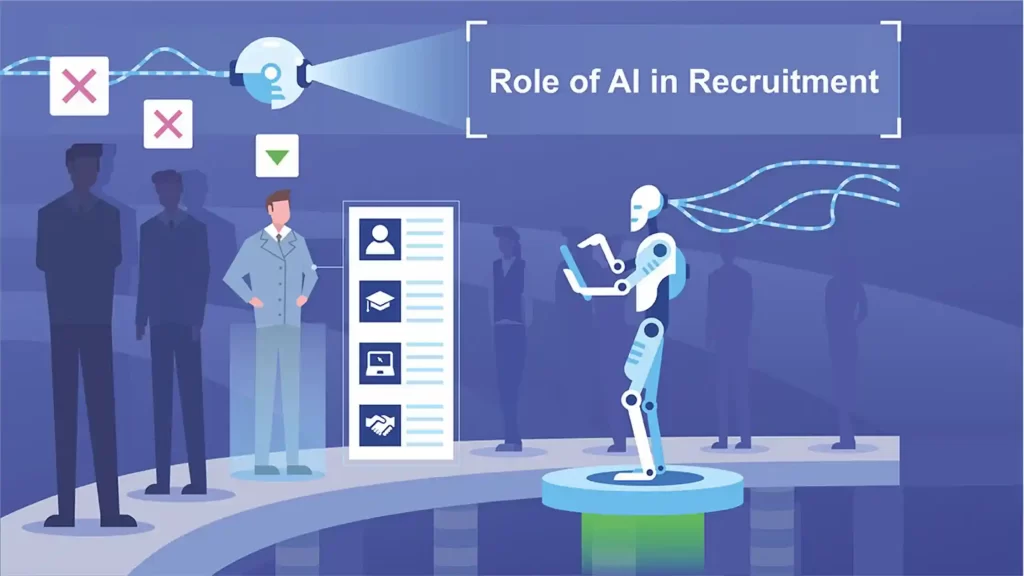
Intelligent Recruitment Systems
Intelligent recruiting systems are becoming an important tool in recruiting for many organizations. Using artificial intelligence and algorithms, smart recruitment systems help companies screen and match the most suitable candidates by analyzing and comparing candidates’ resumes with the company’s hiring needs. Amazon, as one of the world’s largest e-commerce platforms, had independently developed an intelligent recruitment system, whose automated selection process reduces a lot of labor costs. In addition, an intelligent recruitment system can also improve the accuracy and success rate of recruitment. This is because intelligent recruiting systems use a large amount of candidate data and hiring history as criteria to assess the full range of candidates. This data-driven assessment process is free from subjective human emotions and biases, thus enabling an objective assessment of each candidate’s abilities and adaptability, providing a more scientific and accurate basis for hiring decisions. Even if a human spends a reasonable amount of time evaluating applications or resumes, AI tools that utilize algorithms outperform humans by at least 25% in screening applicants (Kuncel, 2014) .
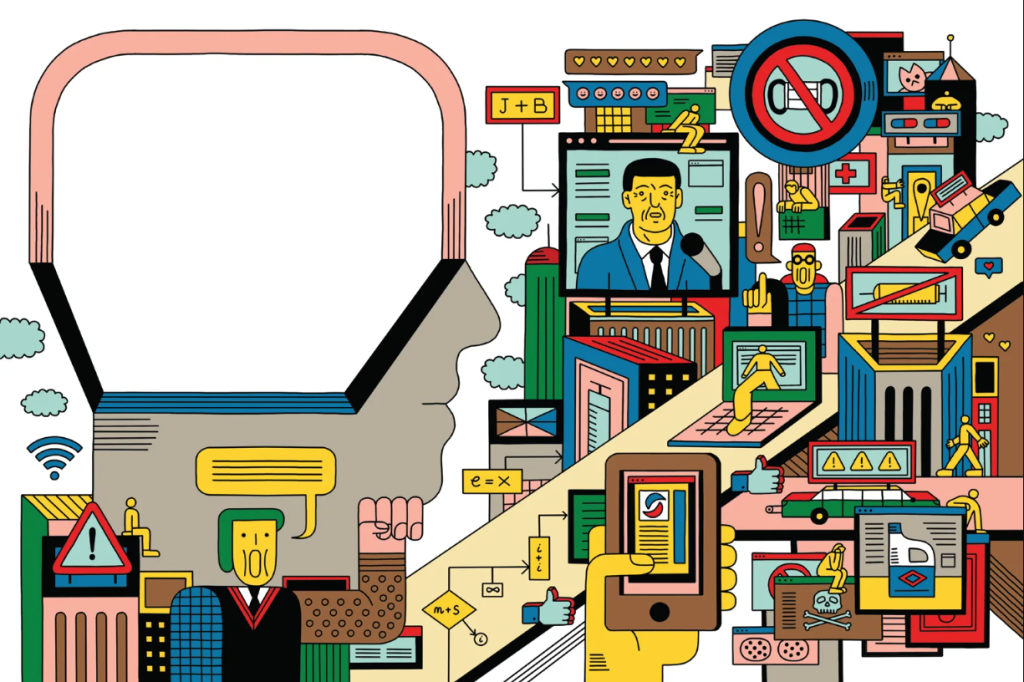
The impact of social media on political decision-making
With the arrival of the digital era at any time, social media has become the main channel for people to communicate and contact. In recent years, social media have influenced public discourse and communication in society (Stieglitz,s et al, 2013), and gradually evolved into an important platform for political opinion and voter decision-making. This is because information dissemination and opinion formation on social media are often influenced by algorithms. Personalized recommendation systems shape users’ political perceptions and attitudes by recommending political news and opinions that match their political inclinations and interests, which in turn affects voters’ decisions and the outcome of political decisions.
Facebook, one of the world’s largest social networking platforms uses algorithms to recommend personalized content to its users. However, in 2016 facebook’s personalized recommendation algorithms were responsible for influencing voters’ political decisions and thus the outcome of the U.S. presidential election. facebook’s algorithms recommend content to users that matches their political preferences based on their browsing history, liking behavior, and social interactions. In the 2016 U.S. election, some political groups took advantage of this feature of personalized recommendation algorithms to target the dissemination of political information and push political advertisements to specific groups of voters. This behavior influences the democratic political decision-making process by intelligently exposing voters to information that shares their political views while ignoring other possible choices.
Algorithms are used in preventive policing
Predictive policing utilizes data and algorithms to predict trends and the likelihood of crime occurring, thus allowing police departments to take preventative measures to reduce crime rates and improve public safety. Algorithms predict the likelihood of a person’s involvement in criminal activity by evaluating data about the person, such as age, gender, substance abuse history, and criminal record. Based on the analysis, the police target enhanced surveillance to prevent crime from occurring. U.S. police have pioneered the application of algorithms to preventive policing. As of 2019, the system has assigned “high risk” scores to more than 400,000 people and is seen as a key tool in preventing violent crime, dramatically improving the efficiency and results of police work.
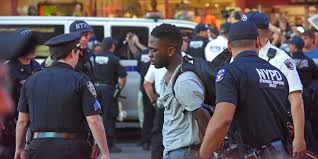
The impact of Algorithms on Human Decision Making
The use of algorithms is subtly changing the way people live and work. In various fields, algorithms have become one of the most important tools to improve efficiency. Algorithm-based artificial intelligence systems possess information processing and decision-making capabilities far beyond those of humans (Black, J.S. & van Esch, P., 2020). From personalized recommendations to smart recruitment systems, the use of algorithms is leading to more efficient decisions and operations. Intelligent recruiting systems remove human bias to automatically screen resumes, thus helping companies improve their hiring success. Personalized recommendation algorithms analyze an individual’s purchase history, browsing behavior, and preference data to recommend goods and services that match his or her interests, thus helping individuals find what they need faster and spend less time shopping. The application of these algorithms has become an integral part of life and work, bringing significant improvements to people’s lives and work efficiency.
Algorithms are gradually playing an important role in people’s decision-making lives, but they are also facing some challenges. Data privacy issues are one of the main obstacles to the application of algorithms. Data privacy is the right of an individual or an organization to protect their information from being accessed and used by third parties. Personal data often includes personal information, behavioral habits, and social relationships. If not properly protected it may lead to risks such as privacy leakage and identity theft. However, with the popularity of personalized recommendation systems, data privacy is gradually facing serious threats. This is because, on the one hand, the collection, storage, and transmission of personal data have become extremely convenient, and on the other hand, personalized recommendation and intelligent decision-making systems require a large amount of personal data as input for algorithms to learn and analyze. For example, personalized recommendations will collect users’ browsing history, and intelligent decision-making will analyze users’ past behaviors. As a result, the collection and utilization of data by governments and enterprises may involve the leakage and misuse of personal privacy information. This makes how individuals to protect privacy in the age of algorithms an important challenge.
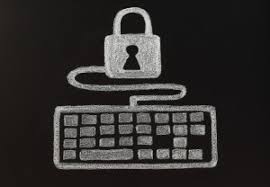
The opacity of algorithms leads to unfair and discriminatory decision-making. Algorithmic analysis can lead to discriminatory outcomes (Lyon, 2005) . This is because algorithms rely on historical data for training and optimization. These data are derived from human life and often contain existing biases and discriminatory information. However, the algorithms themselves are unable to analyze and make judgments on historical data on their own. As a result, the algorithm learns and reinforces unfair information in the data, ultimately generating unfair decision outcomes. For example, Amazon’s intelligent hiring system has been controversial for having made unfair decisions based on gender. Amazon’s AI hiring system uses resumes of employees who have successfully joined the company over the last decade as training data. However, there is a serious gender imbalance in the traditional computer industry, with Amazon having a 60% male workforce. This means that most of the resumes learned by the algorithm are from men. This results in the algorithm negatively evaluating resumes for the word “female”. As a result, intelligent recruiting systems prioritize male applicants when all other competencies of the applicant are equal.
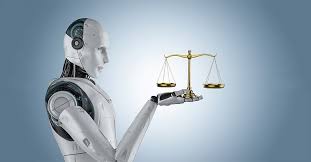
In addition, the application of algorithms in predictive policing shows a certain degree of racial discrimination tendency. First, the lack of transparency in the way preventive policing operates makes these algorithms a “black box” that cannot be audited or evaluated by any outside party. Second, the training databases provided by the police may tend to be racially biased. This is because datasets may disproportionately contain data about certain groups of people, leading to over-monitoring and over-regulation of these groups (Ferguson, 2017). According to the U.S. Department of Justice in 2020, African Americans are two times more likely than whites to be arrested without probable cause. Algorithms use biased data as a learning sample, which can ultimately lead to unfair predictions. The over-reliance of law enforcement agencies on algorithms does not achieve objectivity but rather solidifies long-standing racial discrimination and violates the rights of minorities to be free from arbitrary arrest and detention. The question of how to ensure the impartiality of preventive policing algorithms is therefore an urgent one.
Solutions
Legal Policies
Current versions of algorithms and artificial intelligence are dependent on a range of political and social structures (Crawford, 2021). This means that the establishment of strict laws and regulations can help govern and standardize the problem of algorithm abuse. The law is like a ruler that clearly regulates the scope of use of algorithms, and behavior that exceeds the law will be punished. The European Union recently passed and implemented the Data Protection Regulation (GDPR), which on the one hand sets clear standards for companies and governments to collect, store and use personal data, and on the other hand clarifies the penalty system. In addition, the government should also strengthen enforcement and vigorously punish those who only pursue interests and infringe on personal data privacy.
Algorithm Review Mechanism
Improving algorithm transparency is an effective means to ensure the fairness of algorithm results. Based on the design and operating principles, the operating mechanism of the algorithm is complex and difficult to monitor. But for this reason, companies and governments should make their processes as transparent as possible. For example: simplifying its operating principles and publishing them on the official websites of enterprises and governments to allow them to be subject to public supervision. Under the joint supervision of the public, enterprises, and governments, even if the algorithm’s calculation results are unfair, they can be quickly corrected.
Conclusion
Algorithms are gradually being integrated into people’s daily lives, affecting every daily decision-making. Because of this, people also need to see and avoid algorithm loopholes and their consequences as much as possible. This will maximize the positive impact of the algorithm and allow it to truly serve human needs.
Reference
Crawford, K. (2021). The atlas of AI: Power, politics, and the planetary costs of artificial intelligence. Yale University Press.
Ferguson, A. G. (2017). The rise of big data policing: Surveillance, race, and the future of law enforcement. In The Rise of Big Data Policing. New York University Press.
Kuncel, N., Klieger, D. and Ones, D. (2014). HBR.ORG IDEA WATCH In Hiring, Algorithms Beat Instinct.
Lyon, D. (2005). Surveillance as social sorting: Computer codes and mobile bodies. In Surveillance as social sorting (pp. 27-44). Routledge.
Perez Vallejos, E., Dowthwaite, L., Creswich, H., Portillo, V., Koene, A., Jirotka, M., … & McAuley, D. (2021). The impact of algorithmic decision-making processes on young people’s well-being. Health Informatics Journal, 27(1), 1460458220972750.
Stieglitz, S., & Dang-Xuan, L. (2013). Social media and political communication: a social media analytics framework. Social network analysis and mining, 3, 1277-1291.
Zhou, B., & Zou, T. (2023). Competing for recommendations: The strategic impact of personalized product recommendations in online marketplaces. Marketing Science, 42(2), 360-376.
Be the first to comment