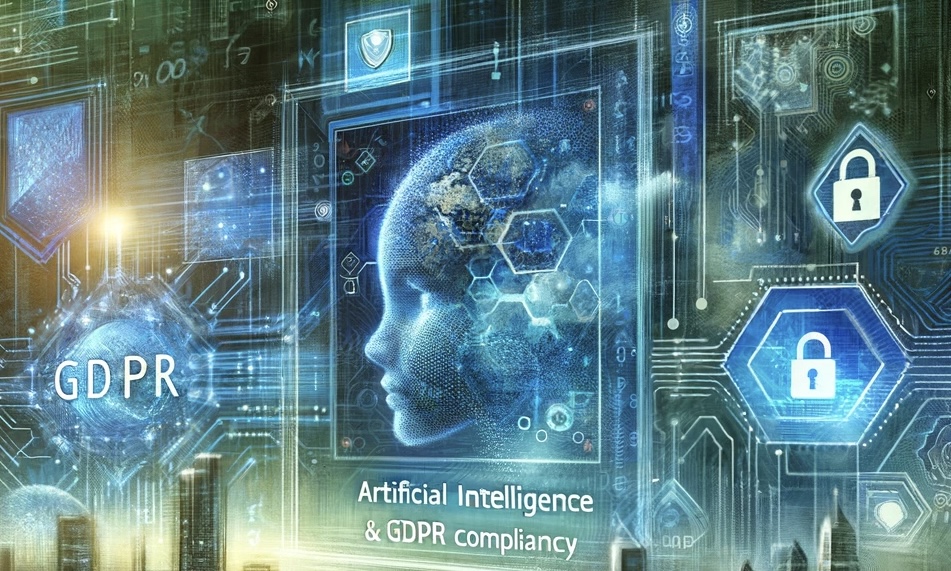
INTRODUCTION
In 2023, organizations are rapidly integrating artificial intelligence (AI) into their operations amidst the rapid growth of generative AI tools.
According to the McKinsey Global Survey, one-third of respondents say their organisations regularly utilise generative AI in at least one business function1.
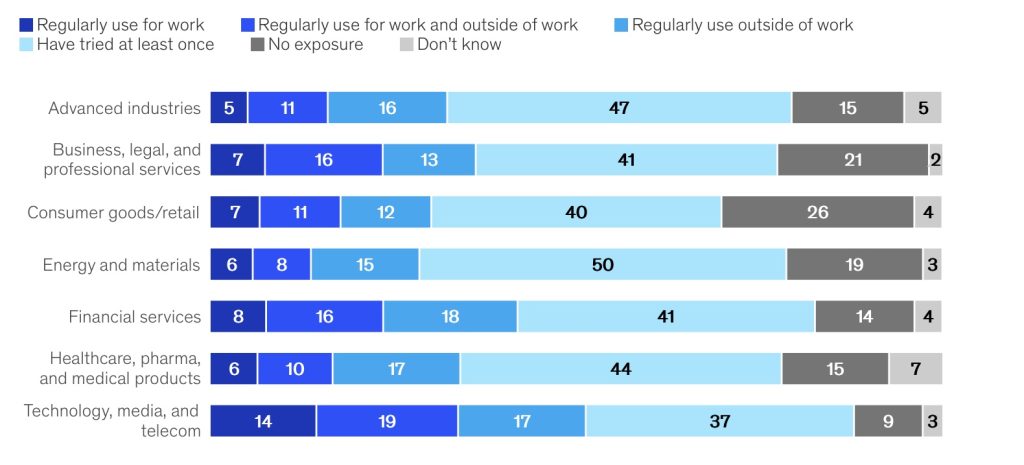
However, as AI reshapes the sector, it provides both significant issues and opportunity for privacy protection.
The European Union’s General Data Protection Regulation (GDPR) establishes strong privacy and data protection requirements, requiring not just compliance with these legislation but also ethical technology use.
In this era of the junction of AI and privacy, this research investigates how technologies such as Differential Privacy and Federated Learning might improve GDPR compliance, with complete support from current literature and real case studies.
Literature Review
Contextual integrity
Privacy is more than just the protection of personal information; it also refers to the context in which information is processed and disseminated. This is entirely consistent with the GDPR’s demand for transparency regarding the context and purpose of data processing. For AI systems, this means that the design must consider the context in which the data will be utilised, as well as the user’s expectations, to guarantee that the technological solution is acceptable in various social and cultural settings. For example, AI recommendation systems that analyse user data to create personalised services must precisely limit what data can be used for what processing objectives while not exceeding users’ reasonable expectations2.
Governance and transparency Issues for Algorithms
Just and Latzer highlights the difficulty of aligning AI operations with the GDPR’s obligations for fairness and openness by pointing to the function of algorithm governance as evidence that automated decision-making frequently lacks adequate transparency and accountability3.
Transparency and interpretability of AI decision-making processes’ operations become crucial societal needs as their complexity rises. GDPR lays forth precise guidelines for automated decision-making, mandating that there be enough openness offered to enable consumers to comprehend and contest the conclusions made by AI. To put this into practice, developers must not only increase the algorithms’ capacity for explanation but also establish the necessary legal and policy frameworks and technical controls. For example, developers must audit the decision-making processes and results of AI systems to guarantee that they are objective and adhere to legal and ethical norms.
Broader human rights impacts of digital technologies
According to Karppinen, K., digital technologies affect human rights such as freedom of speech and privacy. Strict regulations are therefore required to guarantee that AI tools improve privacy rather than compromise it.
AI technologies have a mixed effect on privacy. While big data and AI have the potential to improve services and efficiency, they also have the potential to violate people’s right to privacy.By requiring privacy by design and privacy by default, the GDPR offers legal support for the preservation of individual privacy. AI system designers must ensure that privacy protection is a fundamental component of the system architecture from the beginning. This can be achieved, for instance, by incorporating data minimisation and end-to-end encryption to safeguard user data4.
Public concerns about online privacy
By utilising Australian people as the focus of their analysis, Gerard et al. explain how the GDPR was implemented in response to public concerns about online privacy by raising the bar for data processing and giving users more control over their personal data. A crucial element of implementing AI systems that handle sensitive or personal data is building public trust in the GDPR as a robust privacy framework5.
The intersection between GDPR and AI
Lawful basis for data processing: Legitimate foundation for data processing: Companies developing or deploying AI must ensure the validity of their data processing efforts and that all applicable legal duties are met.
Data Minimization and Purpose Limitation: AI systems should limit data collection and processing to what is required to achieve certain objectives. Data processing should be limited to what is necessary and used only to achieve reasonable, clear-cut purposes.
Anonymization and Pseudo-Anonymization:To enhance personal privacy protection, the GDPR encourages the use of anonymization and pseudo-anonymization techniques. While pseudo-anonymization reduces the likelihood that personal data will be re-identified, it does not alter the definition of personal data; anonymised data is not.
Data accuracy and storage limitations: AI systems that process personal data must keep the data accurate, up to current, and validated, and may not store it indefinitely.
Right to information about automated decision-making: The GDPR compels enterprises to notify data subjects and provide information about the logic of the processing and its material impact when making choices that are totally automated and have a legal or similarly material impact on individuals.
Privacy by design and privacy by default: The GDPR mandates data protection safeguards to be included in the design of AI systems and throughout their life cycle. Organisations must incorporate privacy protection technology into AI applications and guarantee that privacy settings are set to the strictest feasible level.
Data Protection Impact Assessments: The GDPR demands that AI applications that may represent a significant risk to individuals’ rights and freedoms undergo a data protection impact assessment in order to identify and mitigate possible risks.
Security and accountability: Organisations must accept responsibility for the data processing of AI systems and ensure that the relevant AI apps implement adequate security measures to protect personal data. At the same time, further suitable technical and organisational safeguards should be implemented based on the riskiness of the processing activities.
Cross-border data transfers: The GDPR emphasises the importance of implementing proper security measures for cross-border personal data transfers. As a result, companies utilising AI systems must apply appropriate controls when sending personal data worldwide.
Individual rights: AI systems must respect and comply with the rights of data subjects under the GDPR, such as the right of access, the right to rectification, the right to erasure, etc. The GDPR also provides for a prohibition on processing that relies solely on automated decision-making, unless based on specific exceptions.
For more detailed information on the impact of GDPR on AI read Securiti’s expert insights.
Enhancing GDPR compliance through AI technology
Differential Privacy
This technique assures that individual data points cannot be linked back to any user by incorporating randomisation into data processing while maintaining data utility.
Also it consists with GDPR principles and fits the regulation’s objectives for data minimisation and privacy by design, as it protects personal data while allowing for meaningful analysis.
Federated Learning
Federated Learning allows multiple data holders to collaborate on training AI models without sharing their raw data. Models are updated locally and only model parameters or updates are shared.
This technology reduces the centralization and transfer of data, decreasing the risk of data breaches and helping to comply with privacy regulations on data protection and cross-border data transfers.
Case Study: Vodafone’s Implementation of Differential Privacy
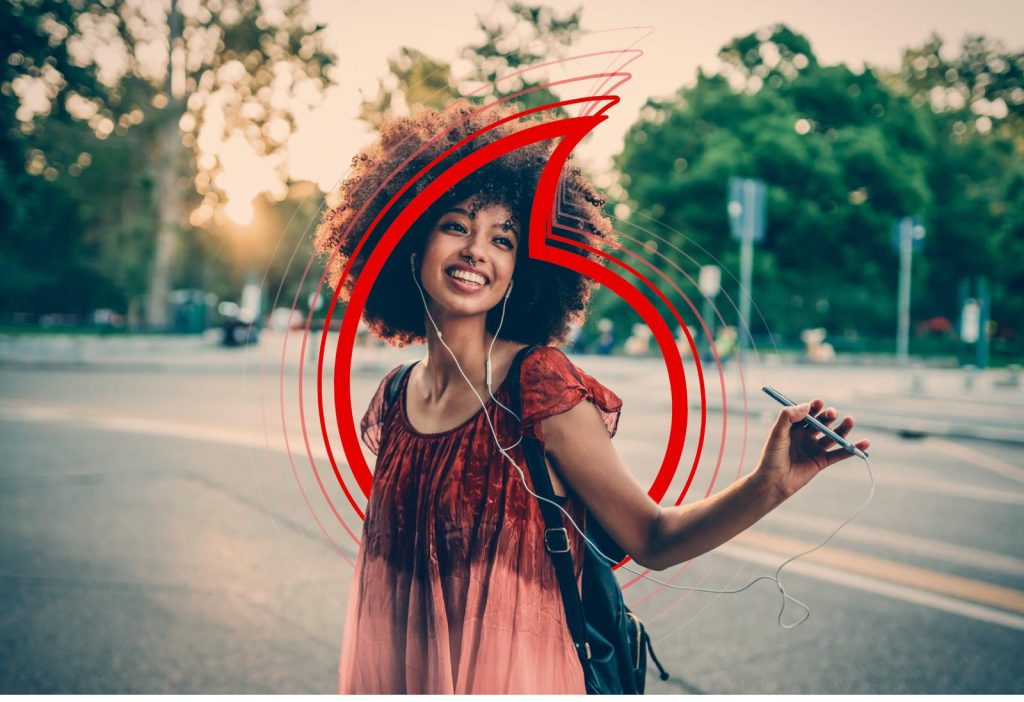
Background
Vodafone, a leading global telecommunications company, was faced with the challenge of optimizing network performance while complying with the strict privacy regulations of the GDPR. With a large amount of customer data to process on a daily basis to improve service delivery, the company needed a solution that would protect individual privacy without compromising the quality of data analytics.
The Challenge
The main challenge was to process customer data for network optimization without violating the GDPR principles of data privacy and security. Vodafone needed a way to ensure that data could not be traced back to any individual, while addressing both legal requirements and growing public privacy concerns.
The Solution
Vodafone employs Differential Privacy, a technology designed to provide a high level of privacy assurance. This approach involves adding controlled random noise to the data before it is analyzed for use, which masks the identity of individual data points while preserving the overall integrity of the data set. This enables Vodafone to gather insightful analysis of network usage and customer behavior without accessing or exposing any individual customer information.
Implementation
Vodafone’s implementation of Differential Privacy involved several key steps:
1. data collection: data is collected from various network points, aggregated and anonymized.
2. Adding noise: Controlled noise is added to the aggregated data before any analysis is performed, ensuring that the information cannot be used to identify any individual customer.
3. Data analysis: Analysts use the modified data to identify patterns and make network improvement decisions, ensuring that all derived insights are based on general data patterns rather than individual data points.
4. Ongoing monitoring and tuning: Noise addition parameters are reviewed and tuned periodically, adjusting for changes in data size and the specific requirements of different analytic tasks.
Results
The adoption of Differential Privacy enabled Vodafone to improve its network efficiency and customer service by
– Ensuring compliance with GDPR: by implementing Differential Privacy, Vodafone complies with GDPR regulations, in particular with regard to data minimization and privacy by design requirements.
– Maintaining customer trust: This proactive approach to data privacy has helped Vodafone maintain and build customer trust, reassuring customers that their personal data is being handled responsibly.
– Enabling data-driven decision-making: Despite anonymization, the quality of insights remains high, enabling Vodafone to make informed decisions, optimize network performance and improve customer satisfaction.
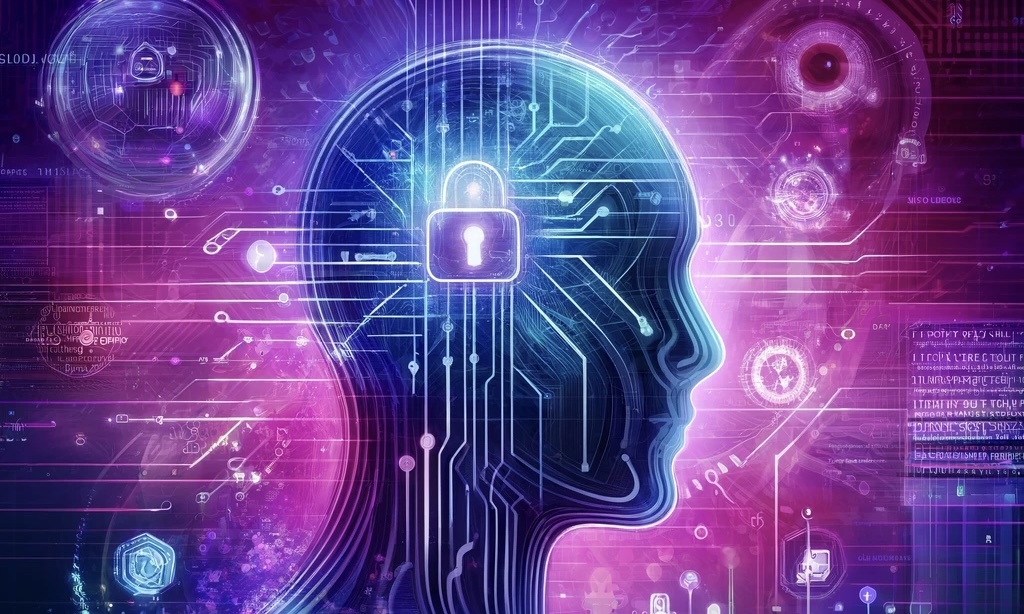
The best practice for AI to enhance GDPR practice
Data minimisation and clarity of purpose
The GDPR emphasises that data collection should be minimal and purposeful, and AI systems should be created and implemented with a strategy for collecting just the data required to perform a specific function. For example, while establishing an intelligent recommendation system, businesses should minimise the collection of users’ personal information and only handle data that is directly relevant to making recommendations. This not only complies with GDPR, but also lowers the danger of data leaking.
Privacy protection design and default settings
Companies are obligated by the GDPR to implement data protection safeguards from the very beginning of product design, sometimes known as “privacy by design”. In the case of AI systems, this entails integrating modern encryption technologies to protect data transit and storage. Furthermore, organisations should have default privacy settings to ensure that no extra personal information is collected without the user’s explicit consent. For example, social media sites can configure their settings to hide a user’s date of birth or contact information by default, requiring users to affirmatively opt in to reveal this information.
Data Protection Impact Assessment (DPIA)
Before introducing new AI applications or systems, the GDPR requires organizations to conduct a Data Protection Impact Assessment (DPIA).AI can play a key role in this process by helping organizations assess potential risks by modeling and predicting the likely impact of data processing activities. For example, an AI model can help analyze and predict the consequences of a personal information breach, enabling organizations to take appropriate mitigation measures before deployment.
Improve the transparency and interpretability of AI systems
The complexity and opacity of AI decision-making is a major challenge for GDPR compliance. Organizations need to work to improve the explainability of AI systems to ensure that users and regulators can understand how AI processes data and makes decisions. This includes the development of explainable machine learning models and the adoption of technical means, such as visualization tools, to help non-technical users understand the AI’s decision-making process. In addition, the addition of Human-in-the-loop (HITL) designs, which incorporate human vetting into key decision-making processes, can increase the transparency and fairness of the system.
Management of cross-border data transfer
In a globalized market environment, data often needs to be transferred across borders.The GDPR has strict regulations on cross-border data transfers that require appropriate protection measures.AI technologies can support this process, for example, by automatically identifying data flows and monitoring compliance issues in international data transfers. In addition, using AI to localize data, for example by processing and storing data within the country of origin, is also a strategy to comply with the GDPR and protect data from external threats.
Conclusion
This blog explored how AI technology can play an important role in GDPR compliance. From data processing legality to user privacy protection, AI technology not only optimises data processing but also improves data security and privacy using approaches such as Differential Privacy and Federated Learning. The article uses real-world examples, such as Vodafone’s Differential Privacy application, to demonstrate how these technologies can help organisations comply with the GDPR’s strict requirements in practice, as well as to highlight the compliance details that organisations must consider when implementing these technologies.
Key Takeaways
Technology-driven compliance: AI technologies, particularly Differential Privacy and Federated Learning, provide organizations with powerful tools to meet GDPR requirements. These technologies help organisations build trust and compliance globally by enhancing the transparency and security of data processing.
Case study: Case studies from organizations such as Vodafone demonstrate how AI can achieve GDPR compliance in the real world. These cases provide quantifiable evidence of how adopting new technologies can help organizations effectively manage and protect personal data.
Ongoing Technology Integration and Evaluation: To remain GDPR compliant, organizations need to continually evaluate and integrate new AI technologies. This requires organizations to not only focus on current compliance requirements, but also anticipate possible future regulatory changes.
Importance of privacy by design: the GDPR emphasizes the importance of privacy by design. Organizations must integrate privacy protections early in the design and development of AI systems to ensure that privacy and data protection concerns are addressed at the root.
Increased Education and Transparency: Improving employee and customer understanding of how AI handles personal data is critical. Education and transparent communication can help deepen understanding and support for corporate GDPR compliance efforts.
Footnotes
- Chui, M., Hall, B., Singla, A., Sukharevsky, A., & Yee, L. (2023, August 1). The state of AI in 2023: Generative AI’s breakout year. McKinsey & Company. https://www.mckinsey.com/capabilities/quantumblack/our-insights/the-state-of-ai-in-2023-generative-ais-breakout-year# ↩︎
- Nissenbaum, H. (2018). Respecting Context to Protect Privacy: Why Meaning Matters. Science and Engineering Ethics, 24(3), 831–852. https://doi.org/10.1007/s11948-015-9674-9 ↩︎
- Just, N., & Latzer, M. (2017). Governance by algorithms: reality construction by algorithmic selection on the Internet. Media, Culture & Society, 39(2), 238–258. https://doi.org/10.1177/0163443716643157 ↩︎
- Karppinen, K. (2017). Human rights and the digital. In H. Tumber & S. Waisbord (Eds.), The Routledge Companion to Media and Human Rights (pp. 95–103). https://doi.org/10.4324/9781315619835 ↩︎
- Goggin, G. (2017). Digital Rights in Australia. The University of Sydney. https://ses.library.usyd.edu.au/handle/2123/17587 ↩︎
Be the first to comment