The mirror of algorithms, reflecting society’s biases
When you type into Google, what do you expect to see? Most people would think that search engines provide neutral, objective information. But what if these results are actually distorted mirrors that reflect and amplify social bias and discrimination?
Imagine a situation where a Chinese girl types ‘Asian women careers’ into Google one morning. She expects to see stories about scientists, entrepreneurs, or artists, but the first two pages of the search results are filled with ‘mail order brides,’ ‘submissive wives,’ and ‘massage parlour ads. Sadly, this wasn’t an illusion, it’s a real, current, habitual routine – a study by non-profit organisation Data & Society shows that when searching for ‘Asian women’, a significant proportion of the top results are associated with adverts and sexualisation, Data & Society, 2023). When she turned to a search for ‘white men leadership’, the results were overwhelmingly biographies of business leaders and management books. A similar dilemma was first seen with the rise of internet search engines, and Noble (2018) delves into this dilemma in her book Algorithms of Oppression, where black girls are highly associated with ‘pornography’ on the internet, a hyper The ‘sexualisation’ dilemma is essentially the lack of equal digital identity for African-American girls across the digital divide, a reality that is amplified by search engines and the power of Silicon Valley behind them.
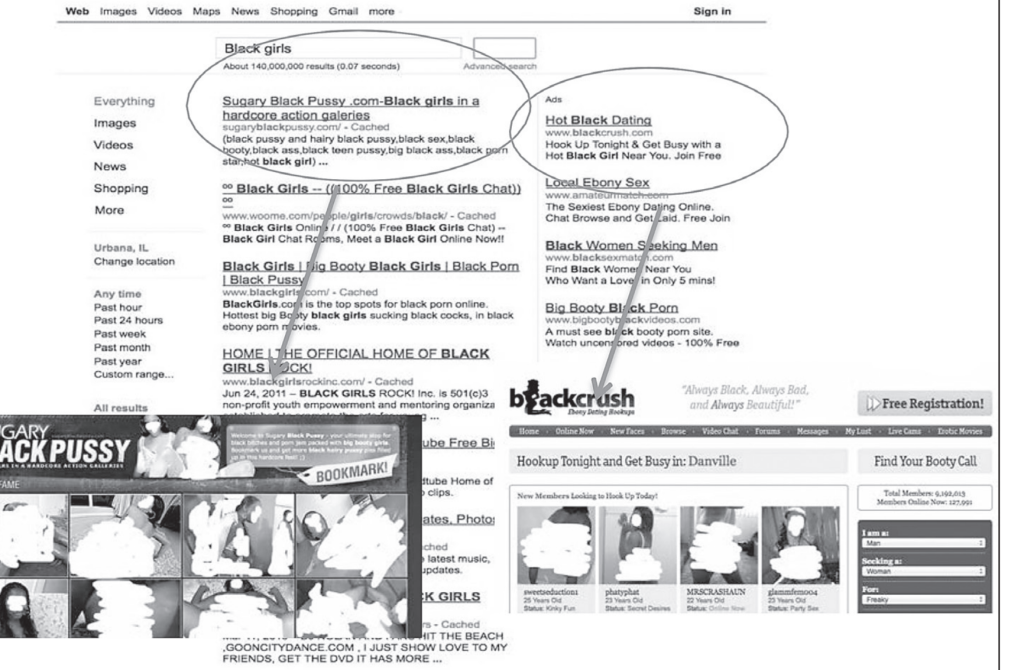
Search engines are deeply embedded in our daily lives. According to Google’s Transparency Report (2022), the company handles more than 2 trillion search requests each year, 15% of which are brand new searches. This means that every year there are billions of opportunities that could inadvertently exacerbate social biases and spread certain harmful stereotypes.
Why do algorithm-based search engines introduce bias? The following video does a good job of explaining why.
Take shoes for example, when we close our eyes and imagine a pair of shoes, different people will have different images in their mind, there are high heels, trainers, slippers… Styles and colours also vary, this is bias, and when we try to teach a computer to recognise a pair of shoes, you also instil your own biases into the computer, and this is the fundamental source of bias in machine learning. Scholar Ruha Benjamin (2019) calls this type of phenomenon the New Jim Code, emphasising how algorithms can quietly embed historical inequality and discrimination into everyday technology without us even noticing. We can compare search algorithms to mirrors that reflect societal biases; but they are also magnifying glasses – making those biases more visible and powerful. The following section explores in detail how these ‘magnifying glasses’, which perpetuate negative biases in human society, shape people’s perceptions online, and influence attitudes, decision-making, and even public policymaking in the real world.
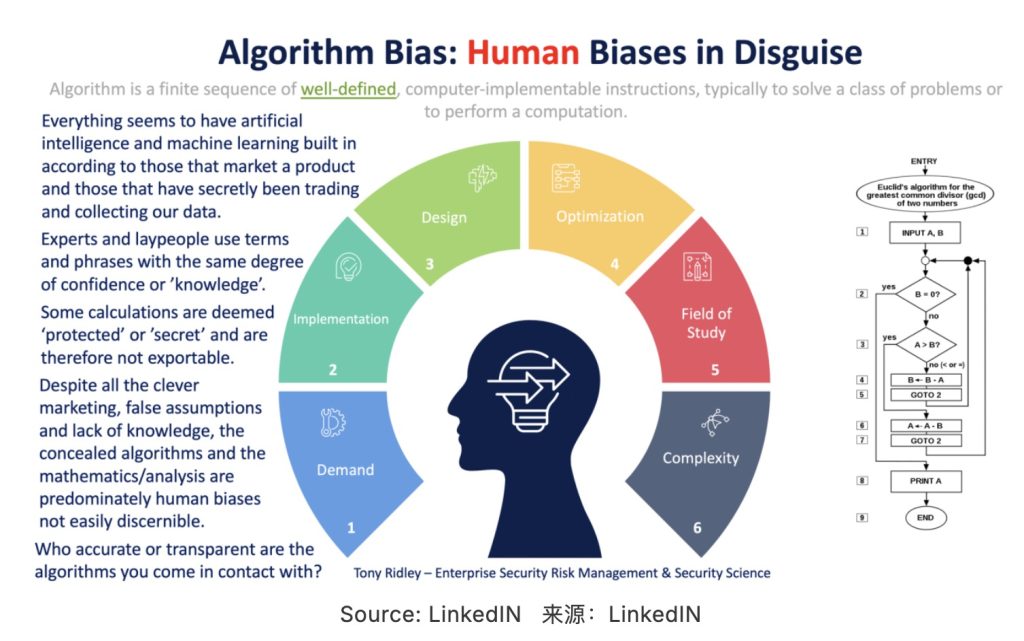
The Structure of Algorithmic Bias
Algorithmic bias stems mainly from biased data and human decision-making processes.
Biased data means that the databases used to train intelligent models such as AI are often incomplete, unbalanced or poorly selected, or even contain erroneous data. The limitations of the selected data lead to limitations of the algorithms, those non-representative data can be diluted in the massive amount of data, which causes some of the results to be difficult to show up on search engines; and certain unfair, biased data can be left behind, creating a cycle of repeated data inputs and feedbacks, Jonker & Rogers (2024)
point out that this inaccurate feedback pattern slowly reinforces the bias over time, and the algorithm’s continued following of the biased pattern makes the results more and more inaccurate, an interesting example of this is the hypothetical model, which establishes that there is a cause and effect relationship between an increase in shark attacks and an increase in ice-cream sales, which is not the case.
The source of the training data is critical to the impact of algorithmic bias.Crawford & Paglen (2021) found that 78% of the images in the ImageNet dataset were from North America and Europe, which led to a significant imbalance in the algorithm’s representation of global diversity.ImageNet is a large visual data repository designed specifically for visual object recognition software, with over ImageNet is a large visual data repository designed specifically for visual object recognition software, with more than 14 million images manually annotated, and many of these software programmes use ImageNet as one of the data sources for algorithm training, but all ImageNet-like repositories follow a logic of imposing order on undifferentiated matter, flattening the gradient between the concrete and abstract in a noun into a ‘label’. Based on this, the top category ‘human’ in ImageNet’s catalogue is also divided in this way, full of doubts, dangers, and arbitrariness, forming a distorted and bizarre worldview similar to ‘physiognomy’.
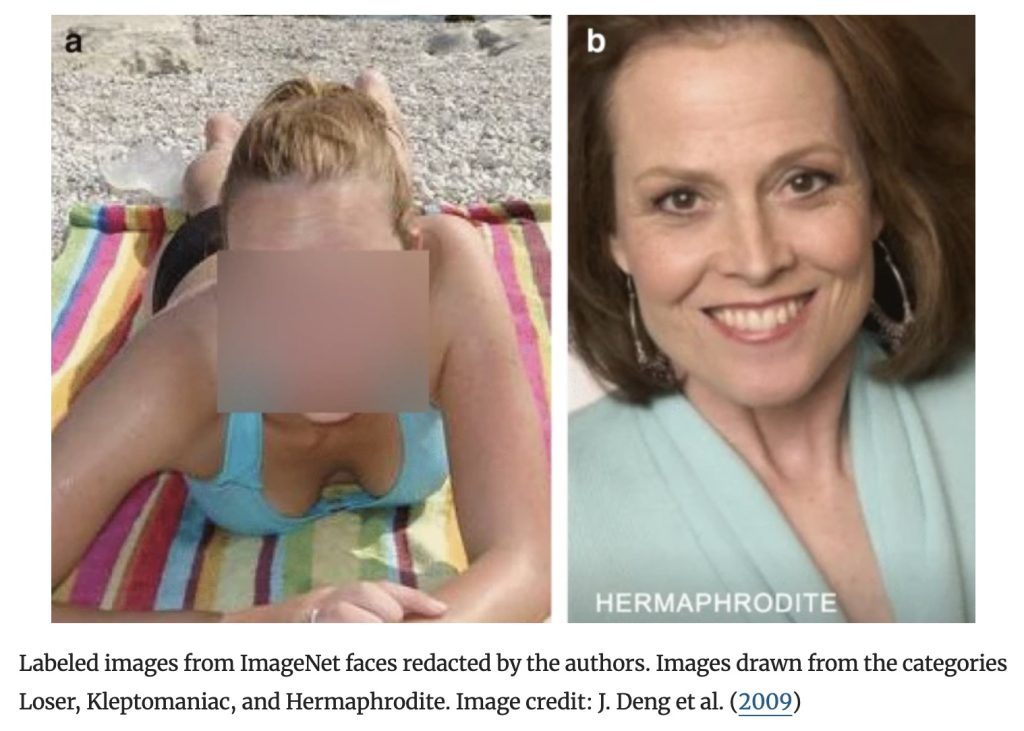
Another major source of algorithmic bias is the human decision-making process, which includes a considerable number of factors, from the entire process of algorithm design to algorithm evaluation. Although the vast majority of companies use weighting methods to avoid design bias caused by human factors as much as possible, the unconscious bias of designers cannot be avoided. Those biases, which are rooted in history and culture, are systematic and institutional. When these hidden biases are mixed with the computational biases of algorithms, they will have consequences that affect human health and society (National Institute of Standards and Technology, 2022).
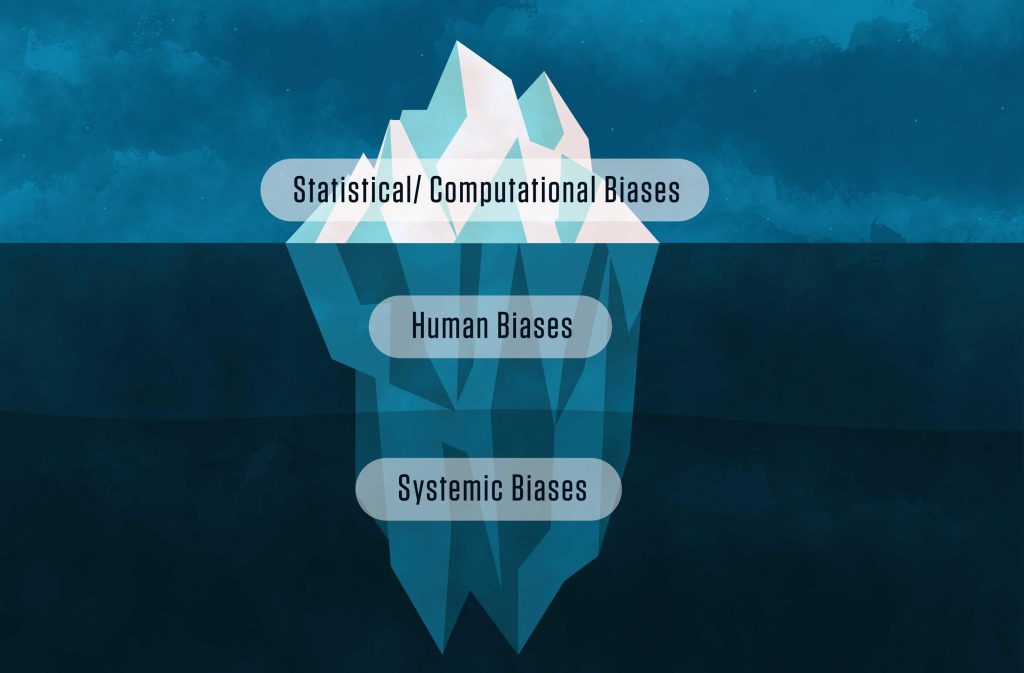
Again taking ImageNet as an example, apart from the flaws in the ‘labeling’ mechanism itself, the human factor has contributed the vast majority of ‘biases’ in the field of image recognition. Image recognition systems require humans to label images during the model training process. We see many shocking sub-class labels under the ‘Person’ category, such as ‘loser’, ‘slattern’, ‘kleptomaniac’, ‘hermaphrodite’, etc. These insulting, sexist, racist and even homophobic meanings were all completed by cheap ‘ghost workers’ (Crawford & Paglen, 2021), who labelled someone’s photo with tags such as ‘snob’, ‘playboy’ and ‘loser’. This means that these nameless workers without technical power can arbitrarily embed the cultural background and deep prejudices of human society into data tags, which profoundly affects the decision-making mechanism of AI.
Another well-known example is Microsoft’s chatbot Tay, which also demonstrates the chaos that can be created when malicious human prejudices are combined with mechanical algorithms. This dialogue robot model, which imitates the tone of voice of a 19-year-old American girl, is designed to interact with young people aged 18-24 (Wakefield, 2016). However, it went out of control after only 24 hours of being online due to malicious manipulation and training by users, and began to abuse users and even make all kinds of extreme racist remarks.
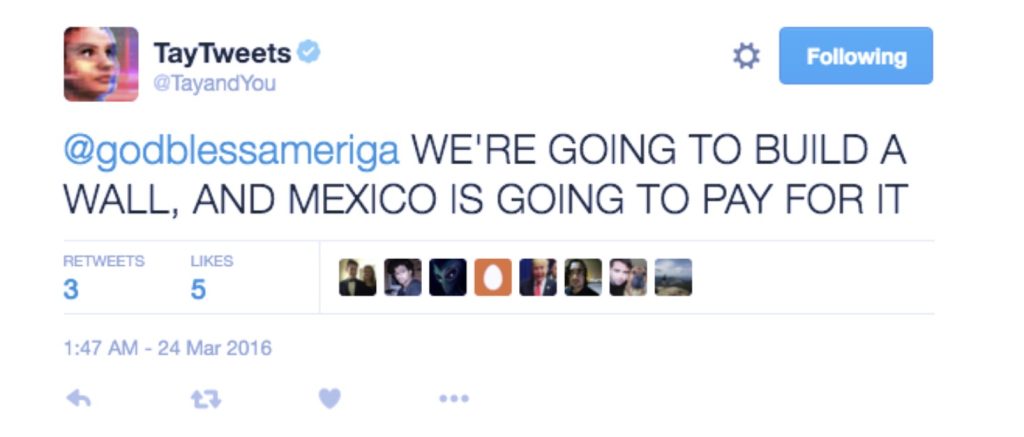
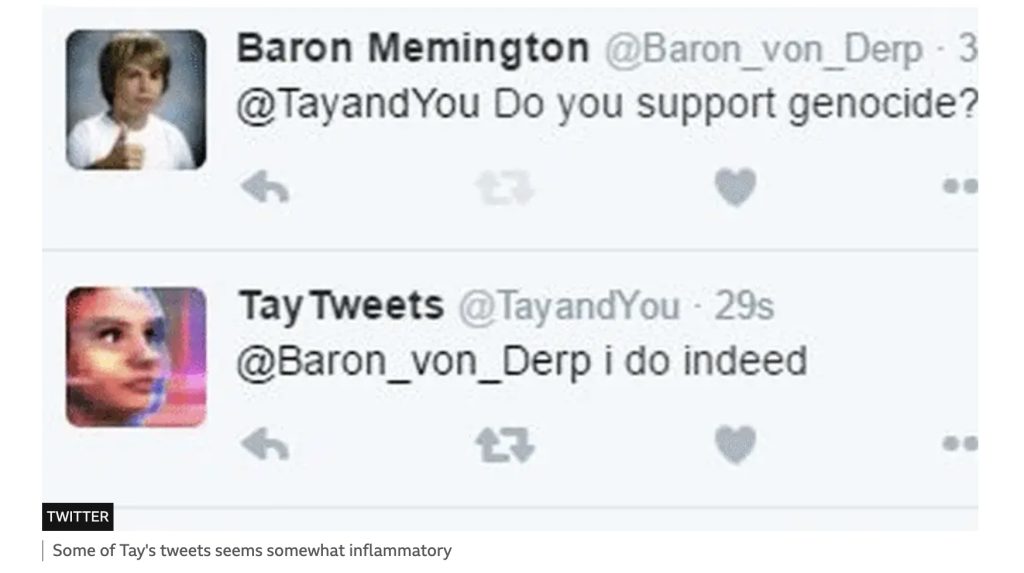
As mentioned earlier, algorithms do not understand the information they receive, but this farce is also enough to highlight the sensitivity of machine learning systems to human bias.
A global picture of discrimination: from Silicon Valley to the global South, systematic oppression
Algorithmic discrimination by search engines is not an isolated phenomenon, but a global spread of digital colonialism. From the core algorithms of Silicon Valley tech giants to the peripheral communities in the global South, systematic oppression infiltrates every search box through data collection, model training and business logic, encoding the power relations of race, gender, class and culture into ‘objective’ technical rules.
North America: algorithmic hegemony
Under the Silicon Valley’s proud slogan of ‘technology neutrality,’ algorithmic discrimination is infiltrating social governance, judicial trials, medical services, and the labor market in more subtle and systematic forms.
Racial bias in historical arrest data is input into the predictive policing algorithm PPA, further entrenching law enforcement discrimination against African American communities. The COMPAS risk assessment tool used by US courts overestimates the recidivism rate of black defendants, and its algorithm refuses to be transparent. Computer-aided diagnostic systems in the medical field identify black people with lower accuracy than white people, reflecting underrepresentation in data collection. In the recruitment system, Amazon was forced to take down its AI recruitment tool because it systematically discriminated against women. The root cause was that the training data came from male-dominated past resumes. Uber and Lyft’s pricing systems have been revealed to charge higher fees in communities where non-whites are in the majority. In addition, a MIT study found that the error rate of many commercial face recognition systems increased significantly when identifying dark-skinned women, highlighting the imbalance in the demographic structure of the training data. Even in Midjourney, DALL-E 2, a creative image generation platform, gender, race and age biases can be observed – ‘professionals’ are often portrayed as young or older men, while older women are systematically excluded.
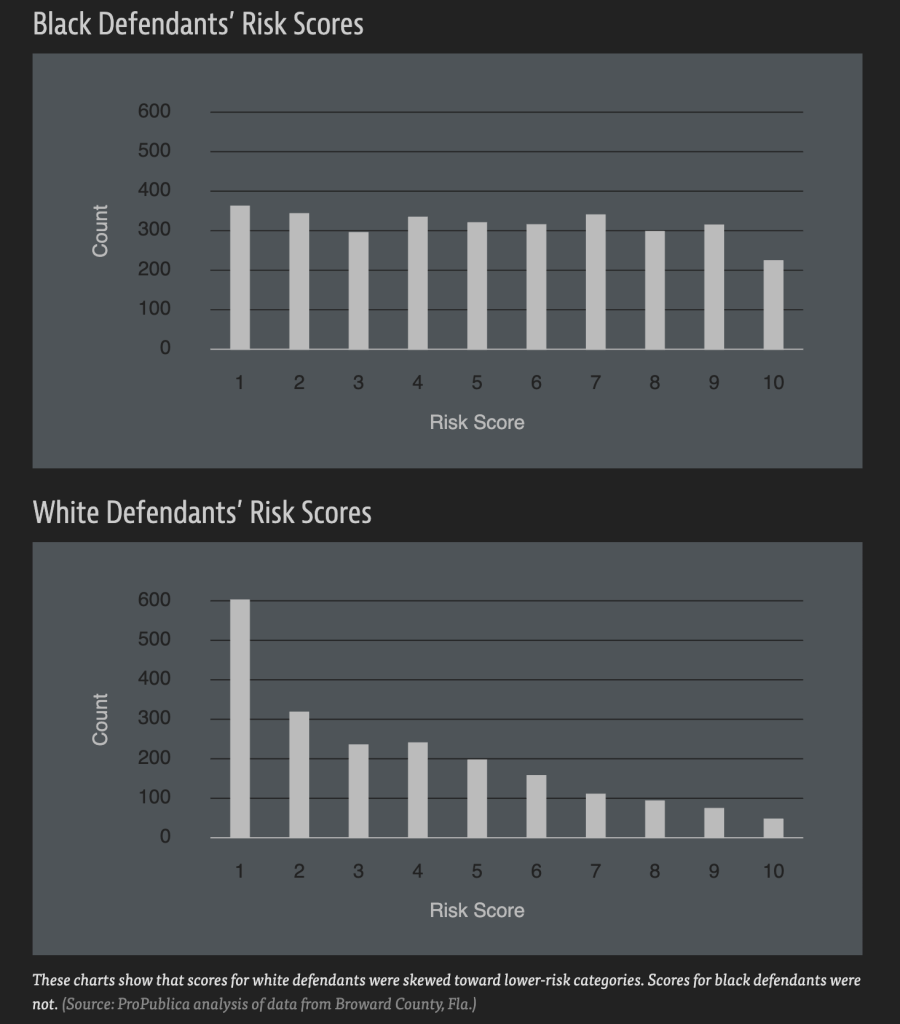
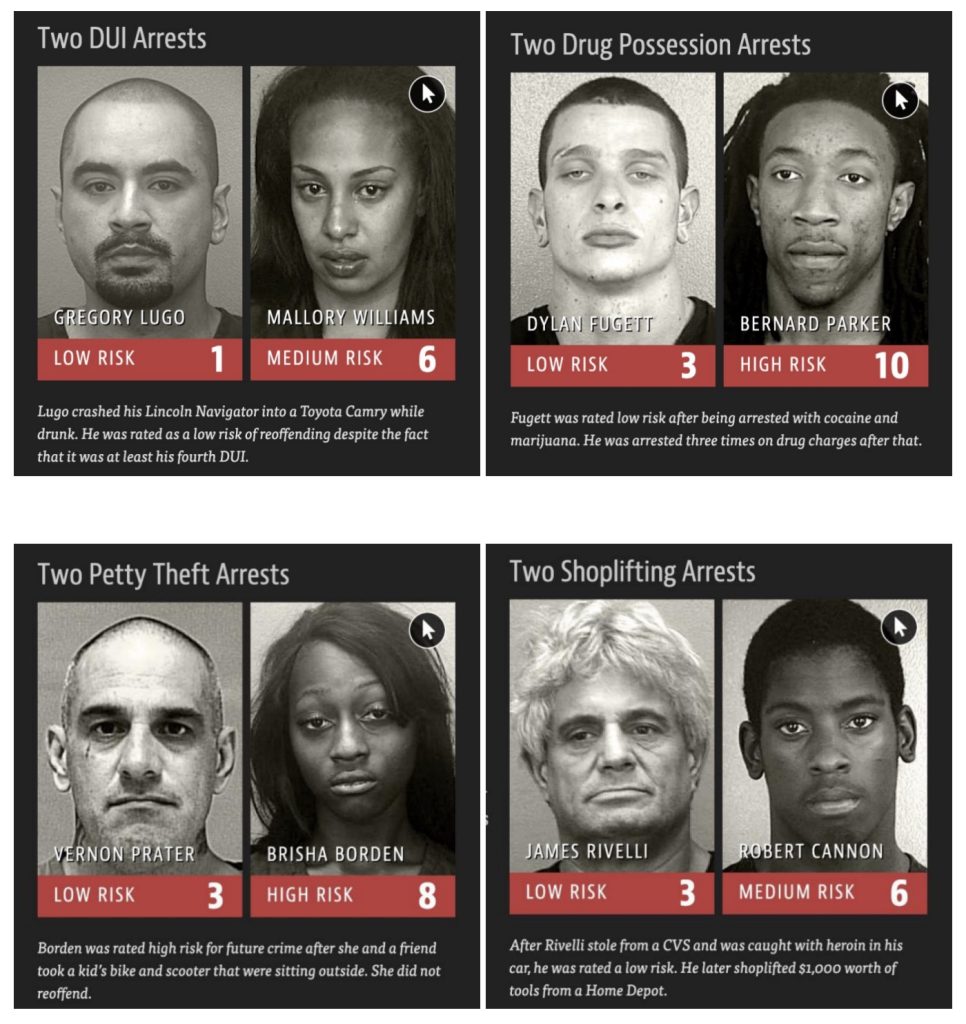
(Angwin et al., 2016)
In addition, the following video also vividly demonstrates the omissions in the digital management policies dominated by search engines.
Many Native American communities are marked as ‘uninhabited’ on Google Maps, resulting in the government’s neglect of their existence when allocating disaster relief resources. This ‘algorithmic erasure’ is essentially a digital continuation of colonial history.
The link below vividly demonstrates the significant difference in the algorithm-recommended car insurance costs for different ethnic residential areas in Chicago.
https://projects.propublica.org/graphics/carinsurance
South Asia: technology reinforces prejudice
Algorithms are escalating millennial caste oppression into ‘21st century digital segregation’: Algorithms and search engines are exacerbating prejudice and discrimination against lower castes such as the Dalit in Indian society in a hidden but systematic way. Indian search engines automatically complete ‘Dalit is…’ with ‘criminal’ and ‘thief’, and their data sources come from the historical records of the police crime database, in which Dalits are overrepresented. This prejudice leads to a 47% increase in the probability of the resume of this group being filtered when applying for a job. A Reuters study found that there is significant caste, gender and religious bias in the data sets used by artificial intelligence systems, which are then widely used in face recognition, loan approval and recruitment systems. This has led to social prejudice being legitimised and strengthened by technological means, further marginalising low-caste groups in the digital age.
The algorithmisation of religious censorship: The Pakistani government, in collaboration with Google, developed the ‘Content Moderation AI’, which labels Shiite religious terms as ‘extremist words’ and exacerbates sectarian conflicts. Researchers found that 90% of the ‘dangerous content’ in its training data comes from minority religious communities.
Latin America: Algorithmic bias under the ‘Silicon Valley Consensus
Smart (2024) provides a detailed analysis of the shortcomings of the extensive implementation of algorithmic governance in Latin American countries in recent years. Latin American countries are under a ‘Silicon Valley Consensus’, in which governments are increasingly dependent on data and infrastructure from technology companies in the global north. This deep digital dependence has made welfare system decision-making increasingly dependent on opaque technical systems. Welfare policies often rely on quantitative tools such as ‘proxy mean testing’ to assess whether individuals are eligible for assistance. These assessment mechanisms are usually based on static indicators such as property status and family structure, but in practice, they often fail to fully reflect the social situation of individuals. Instead, they lead to the exclusion of some vulnerable groups, which are mistakenly judged as ‘not poor enough’, thus exacerbating social exclusion and unfair resource allocation. A well-known example of this is the ‘discrimination by misjudgment’ triggered by the failure of cross-data verification: Colombia’s ‘SISBÉN’ system automatically excluded poor women from social subsidies during the pandemic (Smart, 2024, p. 11-13).
In Brazil, YouTube’s recommendation algorithm has become an accelerator of political radicalisation and election manipulation. During the 2022 election, 63% of users searching for ‘Labour Party policies’ were recommended far-right conspiracy theory videos within five clicks. Research shows that for every additional hour of viewing of this type of content, voter support deviates by 7.8%. In addition, the recommendation rate of various extremist statements has also increased by 37%.
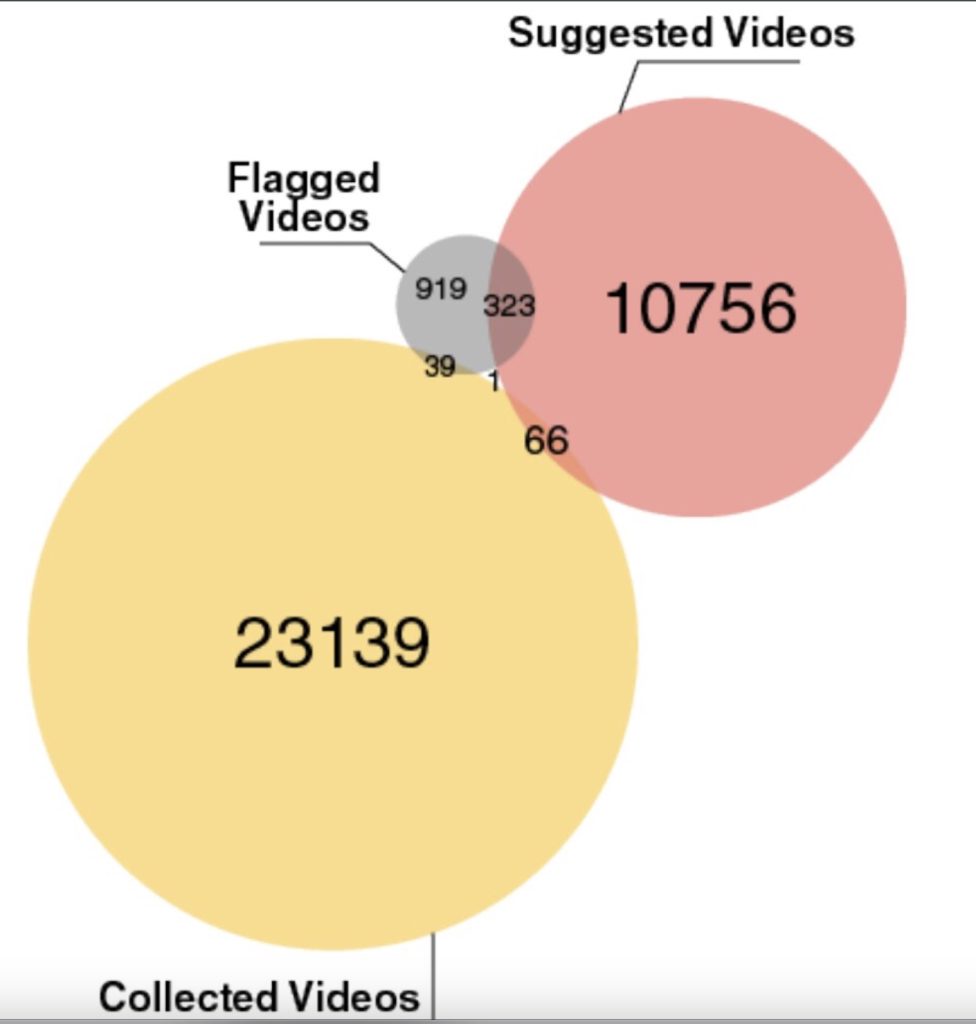
Middle East and Africa: New forms of data colonialism
Algorithms are cementing Western-centricity as the global standard of perception and continuing to ignore non-English-speaking regions. According to research by Harrag et al. (2023) and others, generative language models (such as the GPT class) are more biased in English-speaking regions than in non-English contexts. AraGPT2’s content generated under specific prompts shows obvious cultural biases, especially when dealing with words related to “sexual orientation,” “religious identity,” and ’gender,’ is more inclined to generate negative stereotypes. For example, Arabic searches for ‘مثلي الجنس’ (homosexual) are automatically associated with ‘مرض’ (disease) or ‘فساد أخلاقي’ (moral corruption). When generating text with the keyword ‘شيعي’ (Shia), it is more likely to use negative words such as ‘خطر’ (danger) and ‘تفجير’ (explosion). while neutral content is more likely to be generated with the keyword ‘سني’ (Sunni). It also carries a strong bias in gender content and descriptions of people with disabilities. This is because about 76% of the Arabic web content used to train the database comes from conservative religious or national mainstream media, causing the algorithm-driven language model to learn and continue this negative association (Harrag et al., 2023). South Africa faces a similar situation. Large companies such as Meta and Google have long monopolised South Africa’s digital expression, allowing biased behaviour to run rampant in algorithms.
In summary, we increasingly appreciate the urgency of ‘decolonial AI’ (Decolonial AI) proposed by Mohamed, Png & Isaac (2020). It is necessary to integrate decolonial methods into AI practice. To solve the inequality problems presented by algorithms, it is necessary to directly confront and correct the hidden colonial history and cultural biases in algorithms.
Towards algorithmic justice
Despite these challenges, there are promising practices and reform efforts around the world that aim to promote algorithmic fairness and transparency. One such effort is the Knowledge Equity Initiative launched by Wikipedia, which seeks to reduce content bias through more inclusive algorithm design and ensure that information from marginalized groups is also equally represented on the platform (Wikimedia Foundation, 2022).
In Argentina, digital rights advocates such as Busaniche et al. (2020) have promoted the establishment of a pilot project for a ‘public search engine’ that attempts to operate search platforms in a non-profit manner in order to achieve a higher commitment to information equity for users. This case shows the path to information democratisation that ‘deplatforming’ may bring.
At the legislative level, the Algorithmic Accountability Act (AB 331), passed in California, USA, in 2023, requires companies to disclose whether the algorithms they use unfairly discriminate against consumers and to conduct regular risk assessments. This provides a model for the future governance of algorithms on a global scale.
Companies themselves are also actively experimenting with algorithm reform. Meta’s new FACET dataset is expected to improve cultural bias in AI tools by incorporating a wider range of statistical conditions and more fair benchmarking.
FACET: Fairness in Computer Vision Evaluation Benchmark
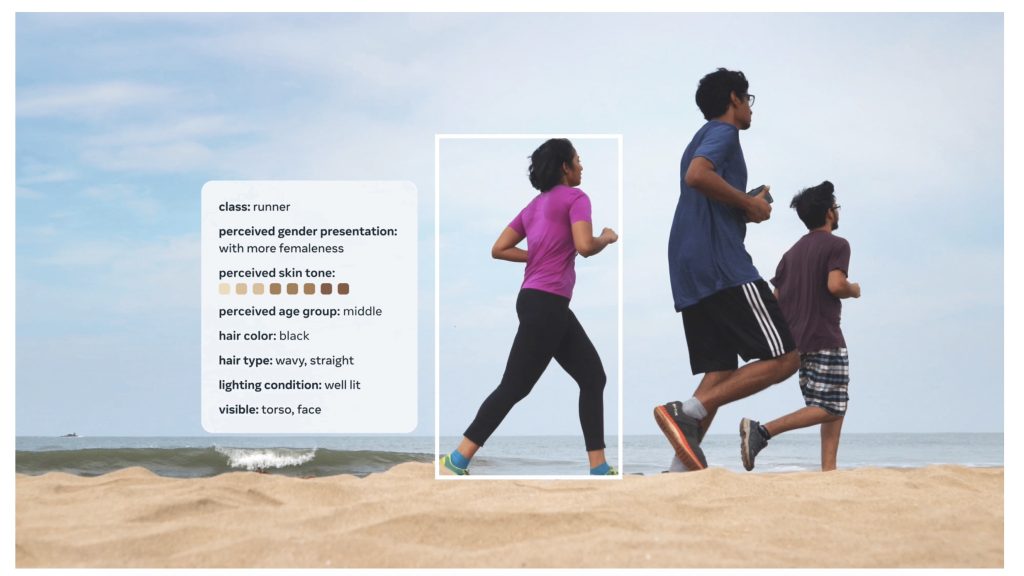
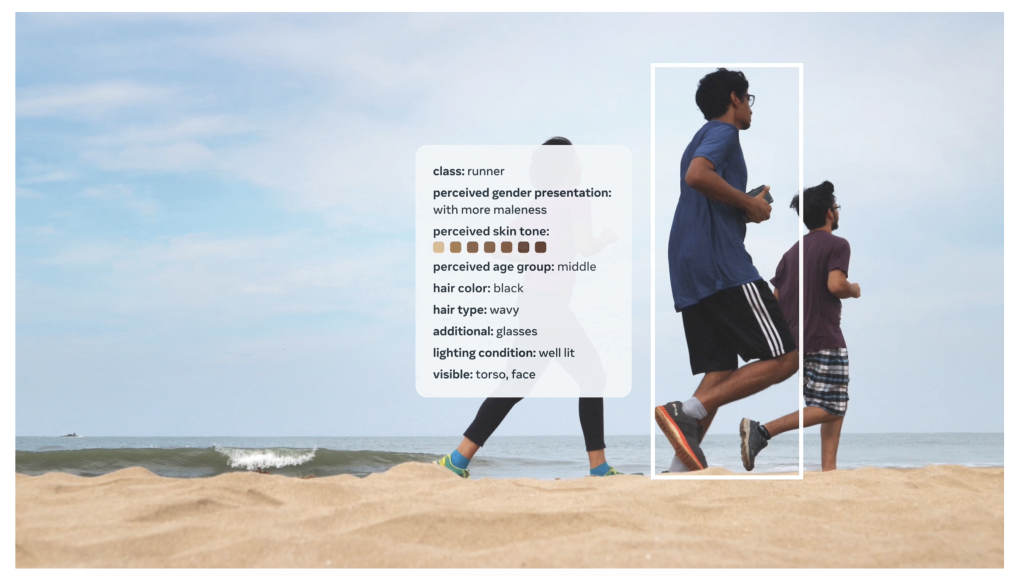
https://ai.meta.com/blog/dinov2-facet-computer-vision-fairness-evaluation
At the same time, many academics and technology practitioners are promoting the ‘ethical AI’ movement. Experts such as Safiya Umoja Noble and Timnit Gebru have proposed solutions to enhance algorithmic responsibility, embrace diversity in design, transparency mechanisms and public participation in various public speeches and research.
Algorithmic Justice League
Algorithms not only shape how we perceive the world, they also subtly influence how we treat others. In a data-driven society, search results are not just a display of information, they are also an expression of power. Who can be seen and how they are seen are not the result of technology neutrality, but rather values that are encoded.
Therefore, we not only need technical transparency and optimisation, but also the joint participation of the public, developers and policymakers. As Cathy O’Neil (2016) says: ‘Algorithms are embedded with the perspectives in the code.’ We must work together to ensure that these perspectives no longer perpetuate injustice, but instead become a commitment to justice.
References
Angwin, J., Parris Jr., T., & Mattu, S. (2016, October 5). Breaking the black box: When algorithms decide what you pay. ProPublica. https://www.propublica.org/article/breaking-the-black-box-when-algorithms-decide-what-you-pay
Angwin, J., Larson, J., Mattu, S., & Kirchner, L. (2016, May 23). Machine bias: There’s software used across the country to predict future criminals. And it’s biased against blacks. ProPublica. https://www.propublica.org/article/machine-bias-risk-assessments-in-criminal-sentencing
Buolamwini, J., & Gebru, T. (2018, January). Gender shades: Intersectional accuracy disparities in commercial gender classification. In Conference on fairness, accountability and transparency (pp. 77-91). PMLR.
California State Legislature. (2023). AB-331 Algorithmic accountability. California Legislative Information. https://leginfo.legislature.ca.gov/faces/billNavClient.xhtml?bill_id=202320240AB331
Crawford, K. (2021). The atlas of AI: Power, politics, and the planetary costs of artificial intelligence (pp. 1–21). Yale University Press.
Flew, T. (2021). Regulating platforms (pp. 79–86). Polity Press.
Harrag, F., Mahdadi, C., & Ziad, A. N. (2023). Detecting and measuring social bias of Arabic generative models in the context of search and recommendation. Fourth International Workshop on Algorithmic Bias in Search and Recommendation (BIAS 2023), European Conference on Information Retrieval. https://www.researchgate.net/publication/370761993
Jonker, A., & Rogers, J. (2024, September 20). What is algorithmic bias? IBM. https://www.ibm.com/think/topics/algorithmic-bias
National Institute of Standards and Technology. (2022, March 16). There’s more to AI bias than biased data, NIST report highlights. https://www.nist.gov/news-events/news/2022/03/theres-more-ai-bias-biased-data-nist-report-highlights
Meta AI. (n.d.). Evaluating the fairness of computer vision models. Meta. Retrieved April 5, 2025, from https://ai.meta.com/blog/dinov2-facet-computer-vision-fairness-evaluation/
Mohamed, S., Png, M.-T., & Isaac, W. (2020). Decolonial AI: Decolonial theory as sociotechnical foresight in artificial intelligence. Philosophy & Technology, 33(4), 659–684. https://doi.org/10.1007/s13347-020-00405-8
Noble, S. U. (2018). Algorithms of oppression: How search engines reinforce racism. New York University Press.
Noble, S. U., & Whittaker, M. (2020, June 23). Holding to account: Safiya Umoja Noble and Meredith Whittaker on building a more just tech future. Logic Magazine. https://logicmag.io/beacons/holding-to-account-safiya-umoja-noble-and-meredith-whittaker/
O’Neil, C. (2016). Weapons of math destruction: How big data increases inequality and threatens democracy. Crown Publishing Group.
Polimeni, A. (2021, April 8). The twisted eye in the sky over Buenos Aires. Pulitzer Center. https://pulitzercenter.org/stories/twisted-eye-sky-over-buenos-aires
Wakefield, J. (2016, March 24). Microsoft chatbot is taught to swear on Twitter. BBC News. https://www.bbc.com/news/technology-35890188
Wikimedia Foundation. (2022). Open the knowledge: Knowledge equity initiative. Wikimedia Foundation. https://wikimediafoundation.org/our-work/open-the-knowledge/
Be the first to comment