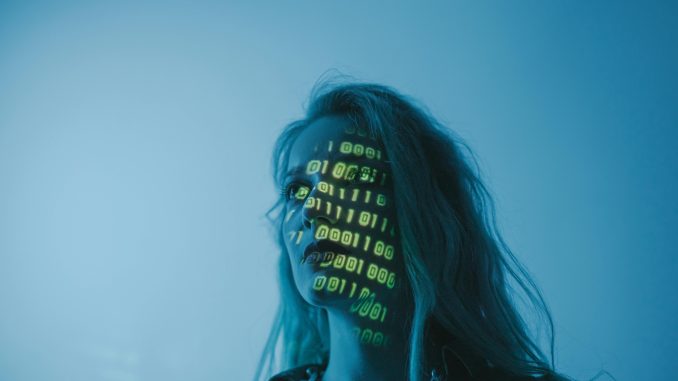
You’re Scrolling for Fun? The Algorithm Has Other Plans
Do you have this experience? You just want to scroll through some funny videos to relax, but you are gradually and unconsciously guided into topics concerning anxiety and uncertainty. The content you see is selected by yourself, or is it arranged?
Nowadays, the recommendation algorithm is a core mechanism of information transmission. It seems to help find the content you are interested in, but it is truly beyond technology, becoming an implicit power structure of reshaping public opinion and manipulating public attention (Suzor, 2019).
Unlike traditional media edited by humans, algorithm-driven content distribution relies on data extraction and predictive models. Your actions give feedback to the system and then determine what you will see. In this case, the content is typically driven by recommendation algorithms because of engagement and monetization needs of the platform’s interest rather than the real needs of users. As a result, the emotional content and fake information will be amplified, and the diverse points and critical thinking are often surpassed.
In this situation, the attention of users becomes a commodity, and recommendation algorithms benefit the platforms (Joy, 2021). Users are increasingly finding it difficult to distinguish which contents are truly autonomous and which are decided by recommendation algorithms. This article will focus on the operational logic, beneficiary groups, and how to govern the unfair recommendation algorithm, combined with the TikTok content suppression case.
Recommendation Algorithm: Personalized for You, or Programmed to Control You?
Recommendation algorithms are often regarded as tools to promote user experience by offering personalized content. It seems to deliver videos, news, and commodities based on users’ interests. However, behind this, it is a complex network of predictive models, behavioral tracking, and optimization mechanisms. The fundamental aim is not to truly serve users but to maximize their engagement.
Platforms like YouTube, TikTok, and Facebook collect vast amounts of users’ data from clicks, watches, scrolling action, even time of pausing. Based on these behavioral data, machine learning models, collaborative filtering techniques, and interest graphs work together to construct a user profile that seemingly ‘understands’ you (Phuong & Phuong, 2019).
However, according to Crawford (2021), this algorithm is absolutely not neutral. It is deeply connected with commercial logic, data-driven biases, and political aims. The algorithm not only reflects the content you are interested in but also constructs a system based on your interests. It continuously increases the recommendation frequency of the content you have seen, the viewpoint you support, and the emotions you tend to respond to, while marginalizing other multiple perspectives. This algorithm leads platforms to offer appropriate content for every user. However, it serves a clear goal: you stay longer and watch more advertisements, and platforms are more easily to maximize interests. In other words, the time your attention is attracted defines how successful the algorithm is.
Online Visibility: Who Gets Seen, and Who Gets Silenced?
On digital platforms, visibility is not only about what you can see but also about who can be seen and who is hidden. The recommendation algorithm determines what can be delivered based on the popularity, keywords, and tags of content. It means some voices will be amplified, while others like those from marginalized communities, are quietly excluded. This process is highly covert, specifically, these voices are not explicitly banned, but they stop being delivered to others. This process is called “shadow banning” (Leerssen, 2023).
As Suzor (2019) points out in Lawless, platforms have created their own systems of governance that operate beyond traditional accountability mechanisms. This system is totally invisible; platforms can individually decide what and who can say and the range of this content. In this case, the platform performs the creator, overseer, and enforcer of the reward-and-punishment system, but without being subject to any public supervision. As a result, users have no way to appeal, while they can not understand why their content is limited or promoted.
Overall, the platform’s invisible governance of recommendation algorithms results in content visibility being arranged, calculated, and unequally distributed.
Case Study: TikTok Content Suppression
In 2019, the German media Netzpolitik exposed an internal moderation guideline from TikTok, clearly pointing out that the platform requires moderators to limit the content visibility of LGBTQ, disabled, or impoverished communities (Köver & Reuter, 2019). Despite these contents not violating the platform’s rules. Noticeably, most users are unaware of this. A large number of LGBTQ content creators reflect that after using LGBTQ tags like “gay” or “trans,” video views will obviously decrease. However, when switching to the neutral tags, the video views return to normal. As Livingston and Bennett (n.d.) argue, although social media platforms seem to be more decentralized compared with traditional media, recommendation algorithms have greater control over public discourse. The platform decides what is worth seeing, while users are often completely unaware. This example clearly supports this argument. Furthermore, this is a typical example of the aforementioned “shadow banning” and directly results in a “chilling effect,” which is that users are worried about their content being limited by algorithms, and users begin to censor themselves but without knowing for sure whether their judgment is accurate (Penney, 2021).
TikTok claims that this measure is aimed at “preventing cyberbullying,” but this explanation reveals a deeper logic behind the platform’s actions. Sinpeng, Martin, Gelber, and Shields (2021) point out that some platforms tend to use vague notions of “protection” to suppress the expression of minority groups. In this case, although TikTok claims it is for “preventing cyberbullying,” the actual effect is the suppression of marginalized communities. Specifically, by marginalizing unreasonable content, this algorithm constructs a more self-satisfied platform image and makes the platform more aligned with marketing goals. It truly demonstrates that TikTok’s recommendation algorithm not only simply reflects the interests of users but also actively shapes users’ visibility frameworks.
Overall, the case of TikTok content suppression reveals the users’ attention and visibility are not self-managed but consciously allocated, calculated, and monetized by recommendation algorithms, and the distribution mechanism serves a clear commercial logic. Without effective regulation, for further pursue commercial goals and expand users, platforms may misuse moderation to suppress critical voices, especially those from vulnerable communities seeking visibility. In response to this issue, Flew (2021) advocates for a co-regulatory framework involving collaboration between governments, platforms, and the public to ensure platform power is transparently governed.
Fighting Back: Can Platform Governance Make Algorithms Fairer?
The TikTok content suppression suggests the urgency of a more reasonable digital platform governance model. In Regulating Platforms, Flew (2021) introduces the Platform Governance Triangle model, emphasizing that effective regulation depends on negotiation and cooperation of government, platforms, and the public, rather than being dominated by a single actor. In this model, they each play their own roles and keep each other in check, therefore building a fairer and more transparent online environment.
Firstly, governance plays a role as rule maker and public interest protector in many ways due to its right to enforce. The government can set the baseline of content governance, such as combating hate speech and protecting user privacy. In the meanwhile, according to the Digital Services Act (European Commission, 2022), government can establish accountability and appeals mechanisms. In this way, the users’ informational rights and freedom of speech can be protected. Additionally, a more direct measure of DSA is requiring platforms to publicize the recommendation algorithm and explain their moderation standards (European Commission, 2022). It can enhance the transparency and legitimacy of platforms and help build a healthy and fair platform environment.
Secondly, platforms play a mixed role as both subjects and agents in Flew’s (2021) Platform Governance Triangle. Specifically, platforms are subjected to government regulation, while setting their own moderation rules and enforcement systems, giving them ultimate control over the flow of information. As a result, even under government regulation, platforms largely determine what users can see, who gets seen, and ultimately shape the overall discourse environment. Platforms not only control moderation decisions but also hold the appeals process, this is obviously shown in the TikTok content suppression case.
Finally, the engagement of the public is the key element of the co-regulatory framework. As the accountability promoter, they can exert pressure on platform governance through public opinion, protests, media exposure, and research reports, forcing government regulations and platform rules to become more reasonable. In the meanwhile, the public takes the role of policy participant, they offer points from different backgrounds and perspectives from multiple angles so the public can promote the inclusiveness and plurality of the platform governance. Additionally, as a norms shaper, Flew (2021) points out that the public not only provides qualitative content for platforms, but they can also use ways like media exposure, running campaigns, and collective boycotts to influence the core interests of platforms so that ensuring platforms’ democratic nature and social relevance. As Suzor (2019) states, platforms actually create a “rule without law” system, and public intervention is the effective force that breaks this structure.
Flew (2021) points out that the Platform Governance Triangle guarantees the openness, transparency, and publicness of platforms. This model can balance the control among government, platform, and public so that ensuring the recommendation algorithm is no longer to break the diversity, fairness, inclusivity, and openness of platforms and guarantee the visibility of all user groups. However, this model operates greatly relying on a vulnerable balance and still carries some potential risks. According to Flew (2021), although government should represent the public interest, government is probably limited by specific political and economic interests and tends to represent the interests of the elite rather than those of the broader public. Turning to the platforms, like the example of the TikTok content suppression, the recommendation algorithm combined with marketing aims is easily used to marginalize minority groups and influence public discourse and discursive diversity. In this case, if the government or platform excessively controls the regulatory power, the triangle will be broken so that it loses the effectiveness of governance and damages the visibility of some users. Moreover, in the practice of the co-regulatory framework, the public is often in a passive position. The lack of public governance directly leads to an imbalanced governance relationship between platforms and government, further affecting the governance fairness (Lu, Zhou, & Fan, 2023).
Beyond the Scroll: Making the Internet Fair Again
Next time when you are scrolling TikTok and perceive the change of content, remember that it is not totally decided by yourself. Recommendation algorithms not only show the content we are interested in but also actively construct our online experience, further leading us to more emotional, more commercial, and more popular views. The TikTok content suppression case reveals how the recommendation algorithm silences minority voices under the excuse of “protecting users from cyberbullying.” The shadow banning measure damages the interests of content creators, burying their creative work even without realizing it. It also quietly damages the diversity and openness of platforms, leading users into filter bubbles and even making them start to censor themselves (Chen & Zaman, 2024). As the response to this problem, Flew’s (2021) Platform Governance Triangle theory offers a clear method for us. He points out that governments need to set clear rules and make platforms more transparent. Accordingly, platforms should optimize the recommendation algorithm to make it fairer to all user groups and take responsibility for how they manage content instead of only focusing on marketing goals. At the same time, we as users also have a role to play. By protest actions, we can push platforms to be more accountable. When governments, platforms, and the public work together, it creates a healthy balance—one that makes platforms more fair, open, and democratic for everyone. However, this kind of ideal balance isn’t easy to achieve. Governments may be influenced by special interests, platforms are often driven by profit, and public participation doesn’t always have a strong impact. Despite the fact that we should be facing the vulnerable balance and objective challenges, our ongoing attention and involvement are really significant for platforms’ transparency. In other words, a fair and diverse digital space doesn’t just happen on its own—it takes constant effort from all of us.
Reference List
- Chen, Y. S., & Zaman, T. (2024). Shaping opinions in social networks with shadow banning. PLOS ONE, 19(3), e0299977. https://doi.org/10.1371/journal.pone.0299977
- Crawford, K. (2021). The atlas of AI: Power, politics, and the planetary costs of artificial intelligence. Yale University Press. https://doi.org/10.12987/9780300252392
- European Commission. (2022). Digital Services Act. https://digital-strategy.ec.europa.eu/en/policies/digital-services-act
- Flew, T. (2021). Regulating platforms. John Wiley & Sons. https://books.google.com.au/books?hl=en&lr=&id=fI1SEAAAQBAJ&oi=fnd&pg=PA1986&dq=terry+flew+regulating&ots=9IVLSCpoz9&sig=w9df4cXDcOWzrh2vWbNEMv15ssg#v=onepage&q=terry%20flew%20regulating&f=false
- Joy, A. (2021). The attention economy: Where the customer becomes the product. Business Today Online Journal. https://journal.businesstoday.org/bt-online/2021/the-attention-economy-asher-joy
- Köver, C., & Reuter, M. (2019, December 2). Discrimination: TikTok curbed reach for people with disabilities. Netzpolitik. https://netzpolitik.org/2019/discrimination-tiktok-curbed-reach-for-people-with-disabilities/
- Leerssen, P. (2023). An end to shadow banning? Transparency rights in the Digital Services Act between content moderation and curation. Computer Law & Security Review, 48, 105790. https://doi.org/10.1016/j.clsr.2023.105790
- Livingston, S., & Bennett, W. L. (n.d.). A brief history of the disinformation age: Information wars and the decline of institutional authority. In S. Livingston & W. L. Bennett (Eds.), The disinformation age: Politics, technology, and disruptive communication in the United States (pp. 3–40). Cambridge University Press. https://doi.org/10.1017/9781108914628.001
- Lu, P., Zhou, L., & Fan, X. (2023). Platform governance and sociological participation. Journal of Chinese Sociology, 10(3). https://doi.org/10.1186/s40711-023-00181-w
- Penney, J. W. (2021). Understanding chilling effects. Minnesota Law Review, 106, 1451–1490. http://dx.doi.org/10.2139/ssrn.3855619
- Phuong, T. M., & Phuong, N. D. (2019). Graph-based context-aware collaborative filtering. Expert Systems with Applications, 126, 9–19. https://doi.org/10.1016/j.eswa.2019.01.009
- Sinpeng, A., Martin, F. R., Gelber, K., & Shields, K. (2021). Facebook: Regulating hate speech in the Asia Pacific. Department of Media and Communications, The University of Sydney. https://hdl.handle.net/2123/25116.3
- Suzor, N. P. (2019). Lawless: The secret rules that govern our digital lives. Cambridge University Press. https://doi.org/10.1017/9781108666428
Be the first to comment