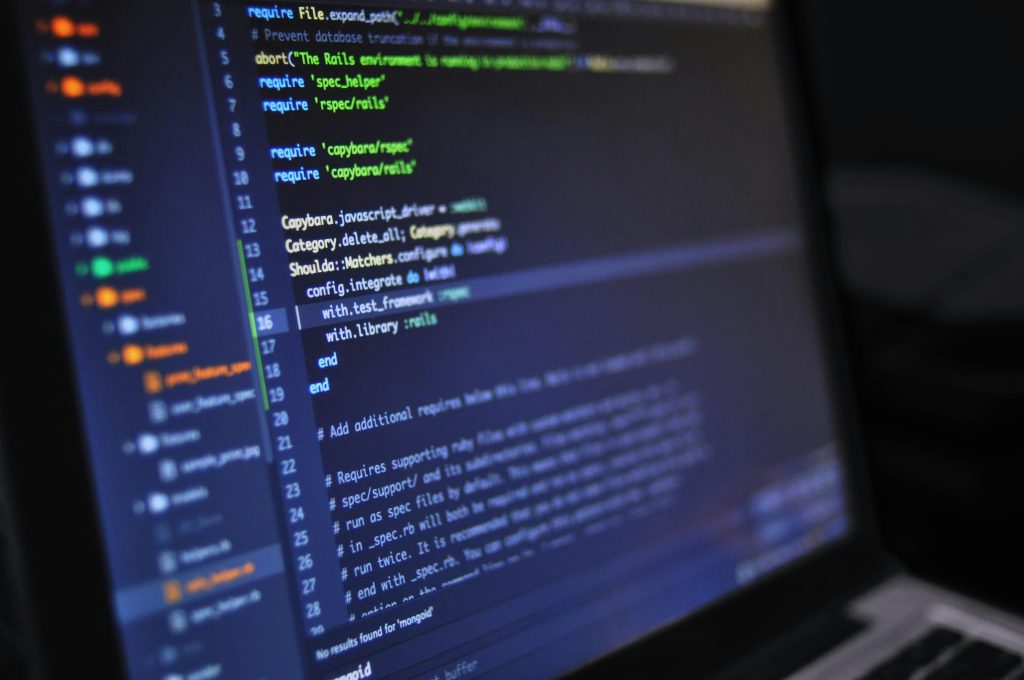
source: https://www.pexels.com/zh-cn/photo/546819/
Do you experience such a moment in your daily life: Scrolling on the TikTok and fall into videos that that feel “made just for you”? It is the “For Your Page”, FYP, a core function design of TikTok, as well as an important upgrade for the recommendation algorithm system to enhance users’ experience and content dissemination. They act like an invisible friend who knows your tastes better than you do. But sometimes, this “friend” feels overbearing: Why am I stuck watching the same type of videos? Does it really know the real me? Let’s unravel the secrets of this digital puppet master.
Algorithms are the rules and processes established for machine-driving activities such as calculation, data processing and automated decision-making. Recommendation systems of TikTok and many other digital platforms are driven by deep algorithms for online services, which recommend and prioritise different content to their users. But how does it support platforms as a powerful tool?
The aSafety Commissioner (2022) indicates the algorithm gathers info and data about users, like their demographic details, or by keeping an eye on how they interact with content, such as locations, likes, comments, or how long they pause on specific stuff before scrolling past. Therefore, users can achieve a sense of community and satisfaction when they spend time on browsing videos online. Even though recommendation algorithms personalise content and improve user experience, they also raise concerns in terms of privacy, filter bubbles, and manipulation of users’ behaviour.
How Recommendation Algorithms Work?
The basic mechanics of recommendation algorithms can be decomposed into many steps, like data collection, machine learning models, engagement-based ranking factors.
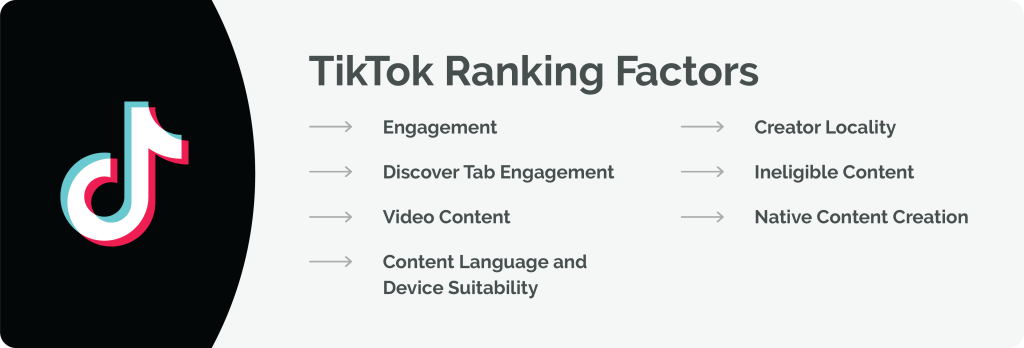
source: https://images.app.goo.gl/w8BoyD9Rz7a99HAXA
Apart from some explicit feedback given by users, like reviews and the number of stars after watching a movie. Recommendation algorithms of Platforms can track users’ watch time, clicks and interactions – implicit feedback – to collect more data. Then they can train volumes of data by AI & machine learning, identify content patterns and adjust recommendations timely. Therefore, highly engaged users can be always attracted by recommended content. For example, the operation of TikTok’s algorithm for new users can be roughly summarized into following steps:
First impressions: When users signs up for TikTok, they provide some basic information, such as their age and location. Upon opening their newly created TikTok account for the first time, their For You page will display some of the platform’s most popular videos, especially those favored by users with similar demographic profiles.
Silent observations: As users scroll through the video, they might like a particular clip, follow a creator, or even navigate to that creator’s profile to explore more content. Each of these small actions carries a specific weight within the algorithm and indicates the level of engagement the user has with the content – if you pause for 3 seconds vs. 10, the algorithm logs it as a “micro-preference.”
Tailored feed: The algorithm of TikTok prioritizes several key elements, known as ranking factors. The more actively the user engages with TikTok, the more data the algorithm collects about their preferences, enabling it to better predict what content they are likely to enjoy. The algorithm maps your interests through every click. It’s like a chef who tweaks a recipe based on your facial expressions while eating.
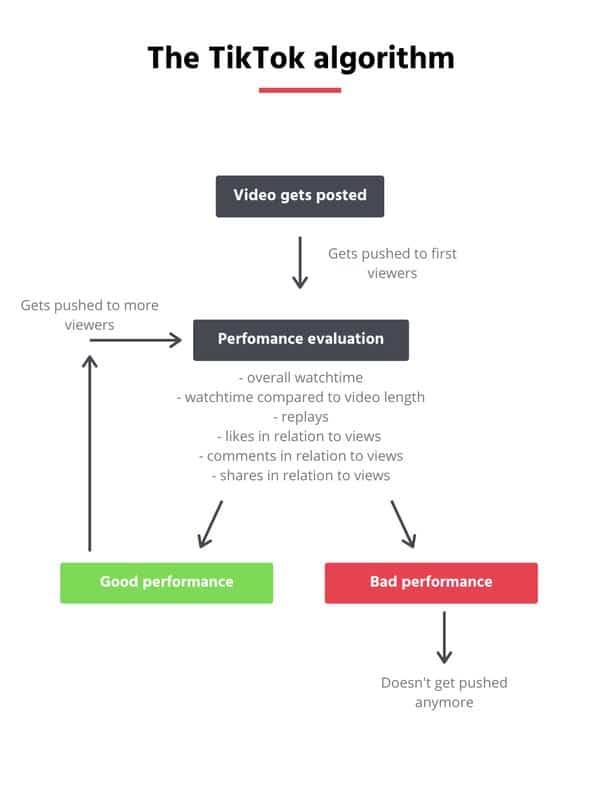
source: https://socialbee.com/blog/tiktok-algorithm/
The superpower of recommendation algorithms
Recommendation algorithms have transformed into an effective tool that bring benefits to the development of economy and society. Users enjoy content that platforms deliver to them accurately. Even though users do not actively search for something, recommendation algorithms can still enable users to discover information or products that they have never known about but are interested in. Furthermore, the recommendation algorithm can effectively filter out those irrelevant contents and services, thereby saving users’ time to a certain extent. Imagine walking into a store where every shelf displays exactly what you need – that’s algorithm – powered shopping.
Take the e-commerce on social media platforms for example:
Recommendation algorithms in e-commerce are aimed at providing customised recommendations of products to customers of websites. They learn from customers from their browsing habits, even search history from other searching engine, then recommend relevant products to users. These make the shopping experience more personal and cater to each user’s preferences. According to Fayyaz, Z et al (2020), there are three main ways a product gets recommended, by showing the top-selling items on a site, by considering the customer’s demographic info, or by looking at what the customer has bought before. When you use a recommendation system, it makes the site feel more tailored because it adjusts to fit each individual customer.
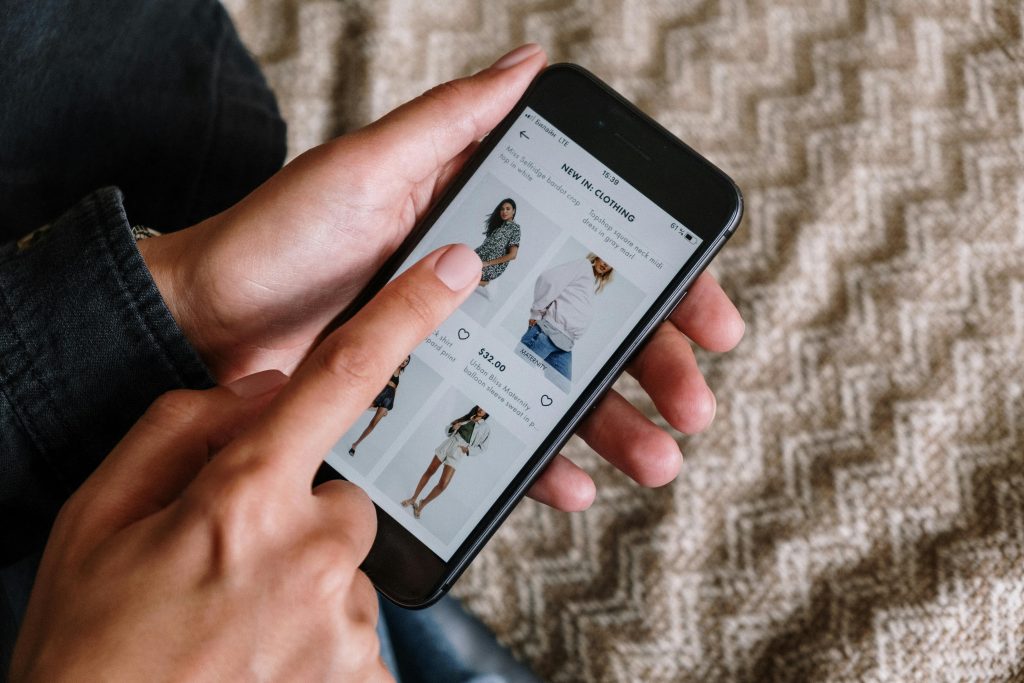
source: https://www.pexels.com/zh-cn/photo/iphone-5076511/
Recommendation algorithms can boost sales on e-commerce sites in three ways:
Turning browsers into buyers: People visiting an e-commerce site often just browse without making a purchase. But if the site shows them relevant recommendations, they’re more likely to be attracted and buy something.
Cross-selling: Besides the product a user is already interested in, recommendation systems suggest other items that might interest them. This way, the average order size tends to grow over time.
Loyalty building: In today’s world, it only takes a couple of clicks to visit a competitor’s site. So, keeping customers loyal is really important. By personalising the shopping experience for each user, these systems help build a strong relationship between the user and the site. The more a customer uses the system, the better it gets at understanding their preferences, which makes recommendations better over time.
Issues and concerns about recommendation algorithms
Although recommendation algorithm systems have brought more customized entertainment experiences to personal life and many benefits to the economy and society, we should also consider problems and concerns that may arise behind them.
Users are likely to get trapped in their own viewpoints as they always browse preferred content that recommended by algorithms. As a result, they would fall into filter bubbles. The filter bubble stems from personalised online content and is regarded as intellectually isolating Internet users and reducing the diversity of information they are exposed to (Fondation Descartes).
For example, on TikTok, a person who has a strong interest in dogs will come across many videos related to dogs in their news feeds. If personalized algorithms make our information horizons impoverished, it means that the diversity of information we encounter on digital social networks is less than offline, and this impoverishment occurs without our awareness. Algorithms show you more of what you like—but less of everything else. It’s like living in a room of mirrors, reflecting only your own interests.
Therefore, as users, we should be aware that digital social networks and internet search engines have provided us with opportunities, enabling us to access information sources that we have never voluntarily sought out. Occasionally search for unfamiliar topics. Think of it as stretching your digital muscles. Or just turn off personalized feeds in TikTok or Instagram settings. Your feed will feel random—but randomness can spark curiosity. Try to make use of recommendation algorithms to explore the knowledge that we desire to gain a deeper insight of.
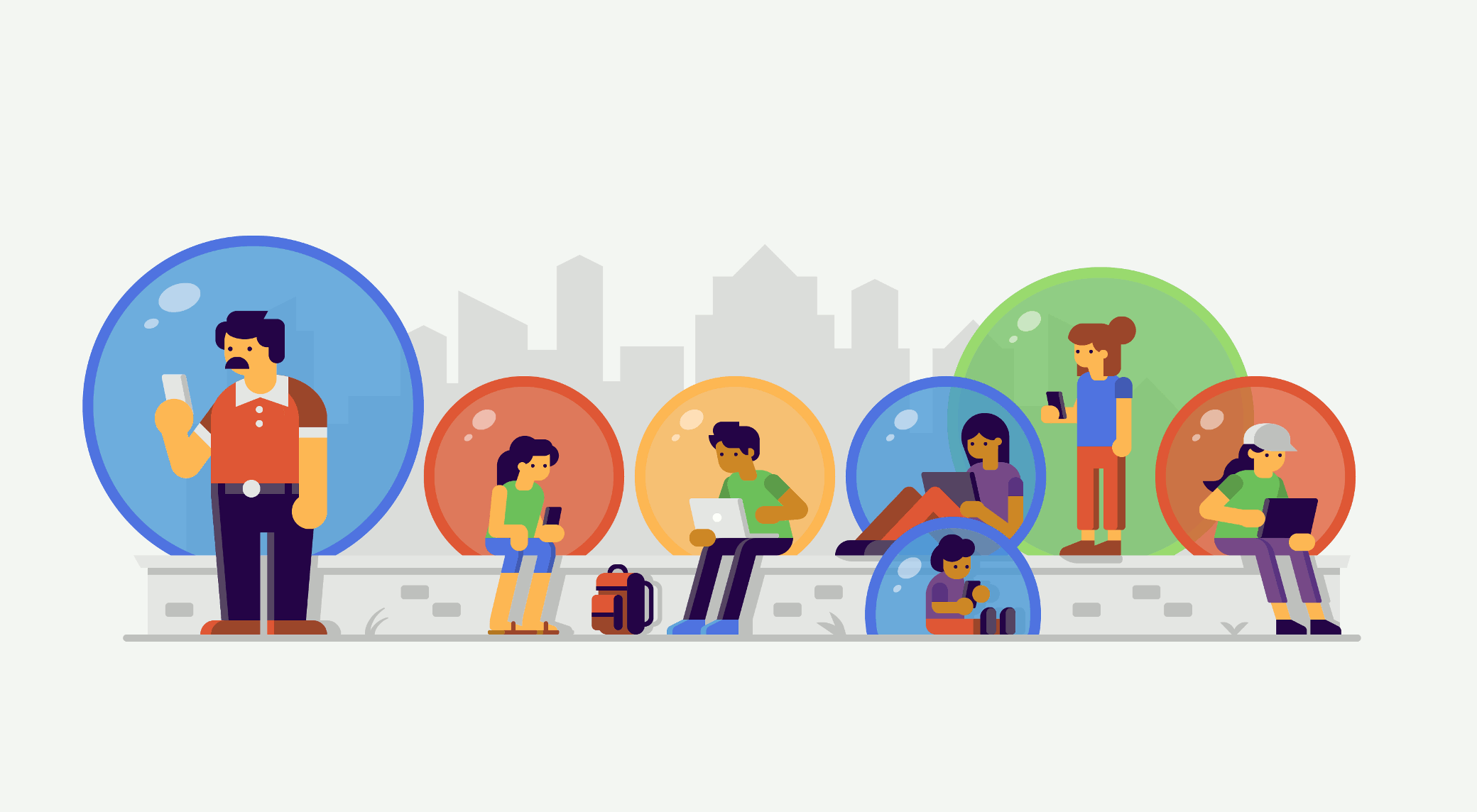
source: https://images.app.goo.gl/8NFmr1iZDYe4WWgL7
Algorithms unintentionally promote extreme or misleading content from time to time. Sometimes, algorithms can be wrong, or content creators have incorrect perspectives lead to misinformation to users, which is false or inaccurate information without intent to deceive (Benkler et al, 2018). By contrast, Benkler et al (2018) explain that misleading information is designed to manipulate target demographics by affecting their beliefs, attitudes or preferences can be defined as disinformation. These misleading information on digital platforms can be hardly distinguished as platforms have more power to disseminate whatever they want you to see.
Platforms collect vast amounts of users’ data to refine recommendations that involve privacy and data security. The refinement of algorithms and the growth of access to data are linked, since algorithms with more data perform better and have greater predictive capacity (Flew, 2021). How can platforms gain these data? Before users enjoy online services and products, they should agree with terms of platforms that are grants for companies to use your personal data, include location, sex, age. The reality is we behave totally different to access online content despite we concern our privacy. The power between platforms and individuals is not even.
What can be done to regulate recommendation algorithms?
Recommendation algorithms are powerful, however, will lead to unexpected harms if do not be monitored carefully.
First, digital companies and governments definitely have responsibilities to regulate recommendation systems, because they are not only owners of these ‘digital infrastructure’, but also stakeholders. Before deploying algorithms to the public, big tech companies should conduct tests under the supervision of government experts to ensure that the algorithms design do not harm public interests. TikTok’s algorithm transparency center is a good example, as the industry is rapidly evolving but their systems, policies and practices are not perfect, which is why they are committed to being transparent about practices, which includes allowing invited experts to view TikTok’s content policies and algorithm at the Transparency Center. It offers opportunities for external experts to directly observe and evaluate the TikTok’s review system, processes and policies.
Digital platforms are supposed to adjust algorithms to reduce misleading information. If the main reason for recommending a certain piece of content is that the user has interacted with similar content before, then related similar content should be provided. Providing such information to users will greatly enhance the transparency of the content recommendation algorithm. Because this transparency will reveal the possible improper correlations that may exist in recommendation algorithms: for instance, Mozilla Foundation found in a study that 12.2% of the videos recommended by YouTube’s content moderation system violated YouTube’s own community guidelines. A 10-year-old girl initially started using YouTube by watching dance videos, but later received video recommendations about extreme dieting, which affected her health.
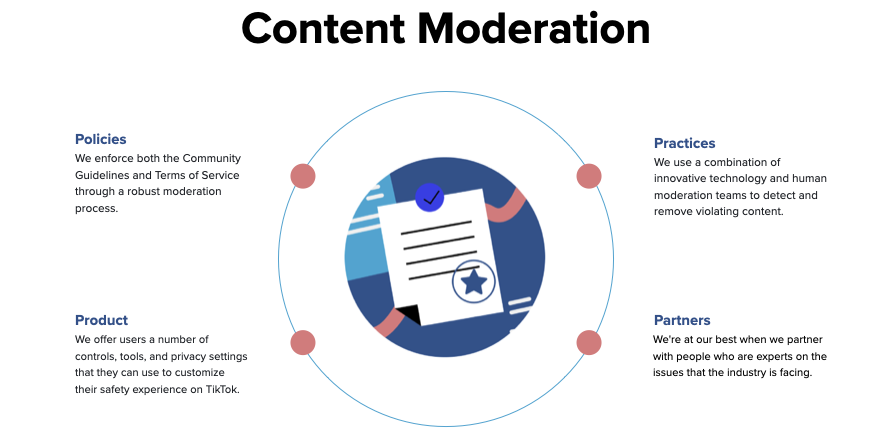
source: https://images.app.goo.gl/PjG1Zp7T3wA99eoQ7
The EU’s Digital Services Act has great impact on recommendation systems. The Digital Services Act requires online platform providers to ensure that content users see in their information streams is more transparent and controllable. This should enable users to understand the criteria on which online platforms rank our content in their information streams and decide whether to opt out of personalized recommendations. Currently, TikTok, Facebook and Instagram all offer options to disable personalized information streams on their respective platforms. Member states and EU commissioners have also been working closely together to monitor and enforce regulations related to the transparency of recommendation systems and advertising, including the implementation of the advertising database (European Union, 2025). This reflects users have more knowledge and control on unique experience online.
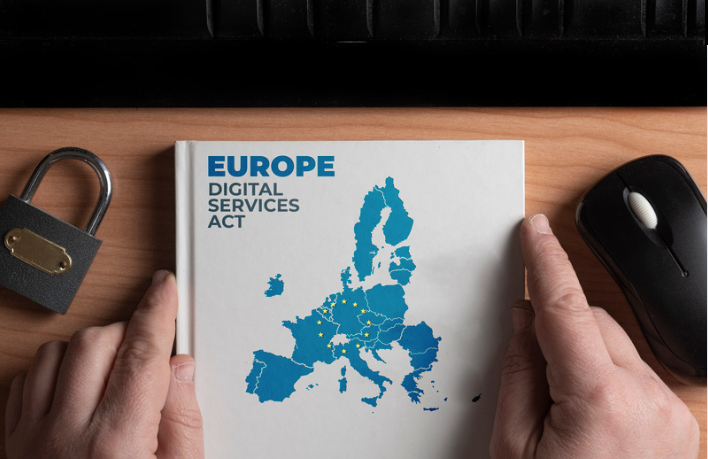
source: https://www.standupforeurope.org/the_eu_digital_services_
act_dsa_and_digital_markets_act_dma
Q: Should we trust algorithms in modern life?
Recommendation algorithms serve as potent tools for both stakeholders and individuals, yet they carry risks that need our attention. They shape what we consume but their accountability remains insufficient. Should we trust contents that platforms decide what we see? Are we truly in control of our choices, or are we subtly manipulated by these algorithms?
On one hand, recommendation algorithms indeed bring convenience to our daily lives. For instance, they guide us to make specific travel plans or select good restaurants with exceptional dining experiences. In these low-risk scenarios, algorithms ca be reliable tools integrate into modern life. On the other hand, AI-based recommendation algorithms can impact everything from minor choices like food or entertainment, to big decisions about economy, healthcare, even ethics. Users should cultivate deeper awareness of how algorithms shape their choices. Therefore, big tech companies and governments are supposed to deliver these information in more clear and simple ways that are relevant to the user’s needs and concerns.
References
Beckerman, M. (2020). An update our virtual Transparency & Accountability Centre experience. https://newsroom.tiktok.com/en-us/an-update-on-our-virtual-transparency-and-accountability-center-experience/
Benkler, Y., Faris, R., and Roberts, H. (2018). Network Propaganda: Manipulation, Disinformation, and Radicalization in American Politics. New York: Oxford University Press.
DuckDuckGo (2018), Measuring the “Filter Bubble”: How Google is influencing What you click. https://spreadprivacy.com/google-filter-bubble-study/
Gomes, L., Code and algorithms. https://www.pexels.com/zh-cn/photo/546819/
European Union (2025), The impact of the Digital Service Act on digital platforms. https://digital-strategy.ec.europa.eu/en/policies/dsa-impact-platforms
Fayyaz, Z., Ebrahimian, M., Nawara, D., Ibrahim, A., & Kashef, R. (2020). Recommendation Systems: Algorithms, Challenges, Metrics, and Business Opportunities. Applied Sciences, 10(21), 7748. https://doi.org/10.3390/app10217748
Flew, T. (2021). Regulating Platforms. Cambridge: Polity, pp. 72.
Fondation Descartes. Filter bubbles and echo chambers. https://www.fondationdescartes.org/en/2020/07/filter-bubbles-and-echo-chambers/
Hamilton, D. (2024). How to Rank on TikTok https://images.app.goo.gl/w8BoyD9Rz7a99HAXA
The aSafety Commissioner (2022). Recommender systems and algorithms. https://www.esafety.gov.au/sites/default/files/202212/Position%20statement%20-%20Recommender%20systems%20and%20algorithms.pdf
Pappas, V. (2020). TikTok launch Transparency Centre for moderation and data practices. https://newsroom.tiktok.com/en-gb/tiktok-to-launch-transparency-center-for-moderation-and-data-practices
Shahzeidi, A. (2024). Decoding the TikTok algorithm for business success. https://socialbee.com/blog/tiktok-algorithm/
Stausholm, Z. (2024). The EU Digital Services Act (DSA) and Digital Markets Act (DMA).https://www.standupforeurope.org/the_eu_digital_services_act_dsa_and_digital_markets_act_dma
YouTube Regrets: A Crowdsourced Investigation into YouTube’s Recommendation Algorithm, MOZILLA FOUNDATION (July 2021), https://assets.mofoprod.net/network/documents/Mozilla_YouTube_Regrets_Report.pdf.
Be the first to comment