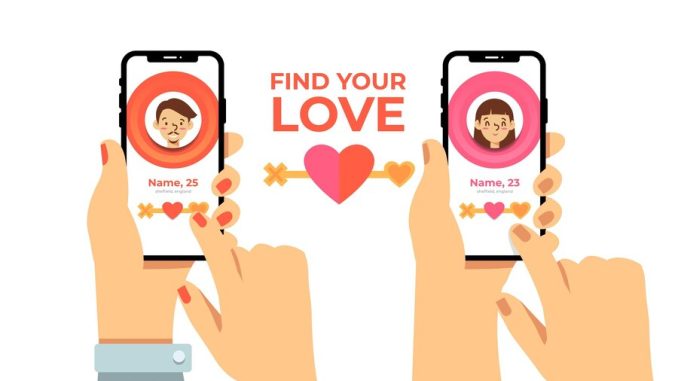
Imagine this: You’re sitting in a coffee shop, absentmindedly swiping through profiles on your favorite dating app. Left, right, left, left, right. Within minutes, you get a match. You smile, start a conversation, and maybe, just maybe, this will lead to something real. But did you really make that choice? Or did an algorithm make it for you?
Artificial Intelligence (AI) is transforming modern dating in ways most people don’t even realize. Every swipe, match, and message is influenced by complex machine learning models designed to keep you engaged, predict your preferences, and shape your love life. Dating apps like Tinder, Bumble, and Hinge process billions of interactions daily, constantly refining their algorithms to offer you the perfect match.
But is AI actually making dating easier and more effective? Or is it just keeping us hooked on an endless cycle of swiping? From deciding who we see to subtly shaping what we find attractive, AI is quietly rewriting the rules of modern romance. Time to zoom in on Bumble—one of the most talked-about AI dating platforms: are users really in control of who we match with, or Should we entrust the experience of true love to algorithms?
How AI Decides Who You Match With
If you think you’re the one choosing your matches, think again. AI is making those decisions long before you even see a profile.
Modern dating apps rely on advanced AI algorithms to determine who is most compatible with you. While the exact methods vary, most platforms use a combination of three core systems: collaborative filtering, content-based filtering, and hybrid models(Thorat et al., 2015, p. 31).
- Collaborative Filtering: Algorithm Recommendation
Think of how Netflix recommends movies. It analyzes your past viewing habits, compares them with users who have similar tastes, and suggests content accordingly. Dating apps use the same approach: if you tend to swipe right on certain types of people, and others with similar preferences do the same, the AI assumes you’ll be interested in similar profiles. The impact of governance algorithms mentioned by Just and Latzer (2017) shows that Internet algorithms are not only tools, but also governance mechanisms used to build and maintain social order (p. 253). This concept is similar to the operation of matching systems, Thorat et al. (2015) found that algorithms analyze user data to determine which matches are “relevant”, which affects the interaction and relationship building between users (p. 32-33).
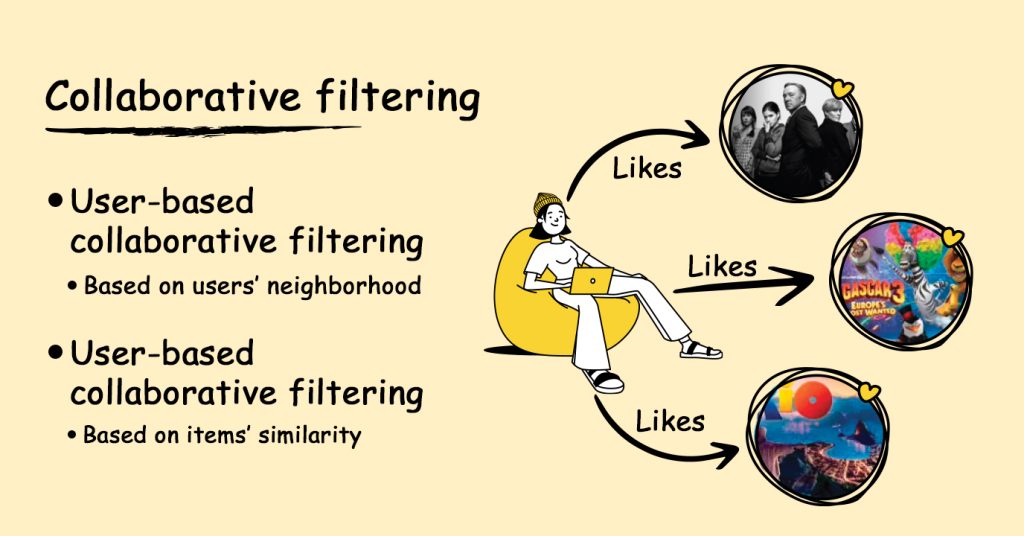
One major example is Tinder, which originally used an Elo score system to rank users based on attractiveness and engagement levels. However, Lokanan (2023) explained that it later abandoned Elo scoring in favor of behavior-based AI models(p. 2). Today, Tinder’s algorithm prioritizes user activity and interaction patterns, analyzing how often you swipe, how long you linger on profiles, and who responds to your messages (Lokanan, 2023, p. 16). This means that your match suggestions are influenced not only by your preferences but also by how active you are on the app.
- Content-Based Filtering: Your Personalized Dating Experience
Unlike collaborative filtering, which relies on group behavior, content-based filtering focuses purely on your personal history. It examines factors like age preferences, location, interests, and even the types of conversations you engage in (Jena, 2022).
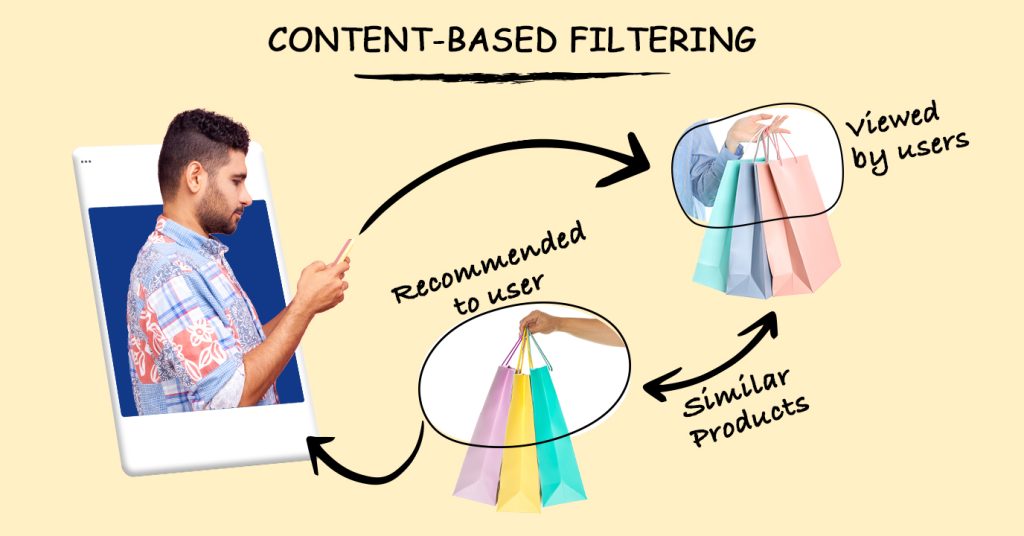
A prime example of this approach is eHarmony, which differs from Tinder by focusing on long-term compatibility rather than quick matches. The platform’s AI analyzes responses from a 150-question personality test, refining match suggestions based on psychological compatibility and communication styles (eharmony, n.d.). Over time, the algorithm adjusts its recommendations as it learns more about your dating behavior.
Analyzing user data for personalized reality construction is an important aspect of algorithmic governance. The selection of automated algorithms not only affects individuals’ matching results, but also shapes their perception of reality and social behavior. In this process, users are not only passive recipients of information, but also act as the “secondary gatekeepers” (Just & Latzer, 2017, p. 248), providing important data inputs in the algorithm selection process. This role highlights that algorithmic governance is a result of digital technology and an important component of social structure and cultural cognition.
- Hybrid Models: The Ultimate Matchmakers
Thorat et al. (2015) pointed out that most modern dating apps now combine both collaborative and content-based filtering to improve accuracy (p. 34). One of the most innovative examples of a hybrid model is Hinge, an app that markets itself as being “designed to be deleted” (Hinge, n.d.). Unlike Tinder, which prioritizes engagement and swiping frequency, or eHarmony, which relies on extensive questionnaires, Hinge takes a more dynamic approach to matchmaking (Jena, 2022).
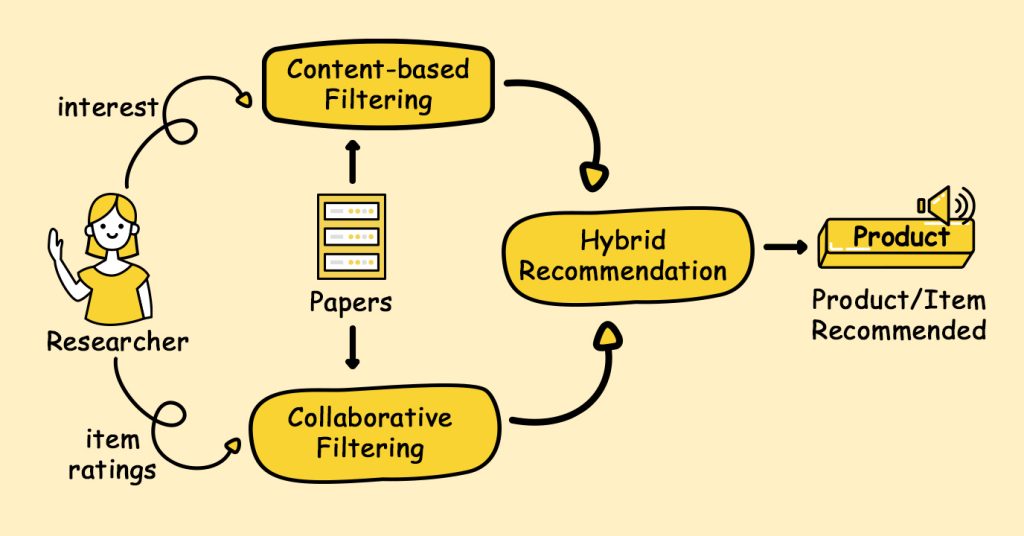
A key feature that sets Hinge apart is its “We Met” feedback system. After going on a date, users are encouraged to report back to the app, answering a simple question: Did the date go well? This feedback provides the AI with valuable insights, helping it to understand which types of matches lead to positive, real-life connections. If two users report that they enjoyed their date, Hinge’s algorithm will look for patterns in their profiles—similar interests, shared values, or even subtle conversational cues—and prioritize recommending similar matches in the future (Hinge, n.d.). On the other hand, if a match does not lead to a second date, the AI will adjust its approach, ensuring that future recommendations are better aligned with the user’s actual dating experience rather than just their initial swiping preferences (Jena, 2022).
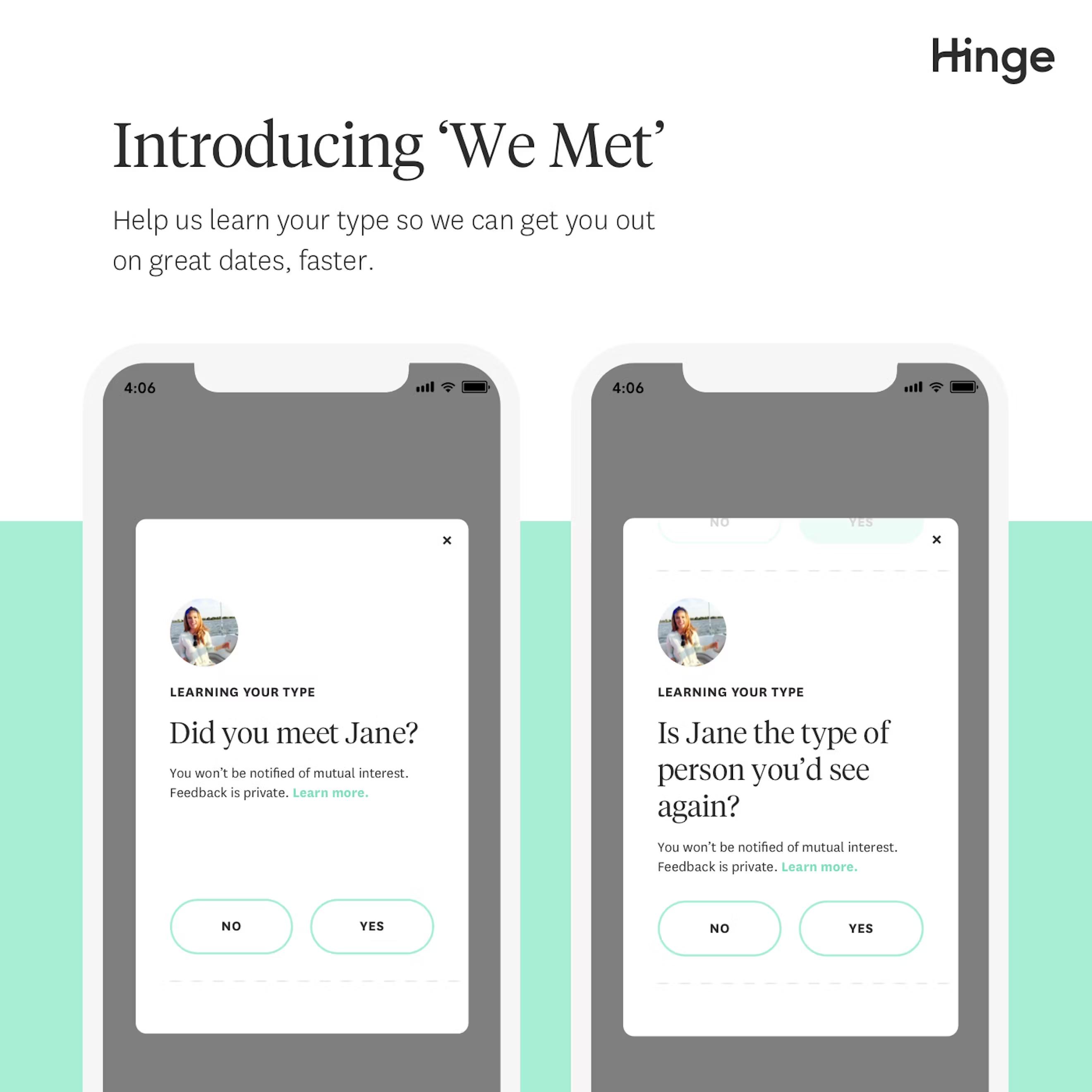
“Every single time we’ve made the app more effective at getting people out on great dates, we spread faster, because more people have good experiences that they then tell their friends about“ by Hinge founder — Justin McLeod (Levesley, 2019).
Bumble-Unique AI dating experience
How Bumble Uses AI to Curate Your Dating Experience
While many dating apps rely on AI to enhance user experience, Bumble stands out for its AI-driven personalization that not only refines matches but also shapes user interactions. Unlike other platforms where both parties can initiate conversations, Bumble’s unique feature—“women make the first move”(Bumble, n.d.). Eddington and Buzzanell (2020) highlighted it is a fundamental part of how its AI operates, by analyzing user behavior, engagement patterns, and even conversational tendencies, Bumble’s algorithm constantly adjusts recommendations to increase the likelihood of a meaningful connection (p. 432-434). But how does this actually work?
At its core, Bumble’s AI monitors how users interact with the app. This includes tracking who you swipe right on, how long you spend on certain profiles, what kind of messages lead to responses, and even the success rate of past matches (Eddington & Buzzanell, 2020, p. 427). Over time, this data helps the AI predict not just who you might find attractive, but who you are most likely to engage with in a real conversation. Unlike Tinder, which often prioritizes immediate attraction based on photos and quick swipes, Bumble’s AI focuses on interaction-based learning (Eddington & Buzzanell, 2020, p. 431), refining matches based on deeper engagement rather than just surface-level attraction.
From “Best Bees” to Safe Spaces: Predictive Romance and Personalization in Action
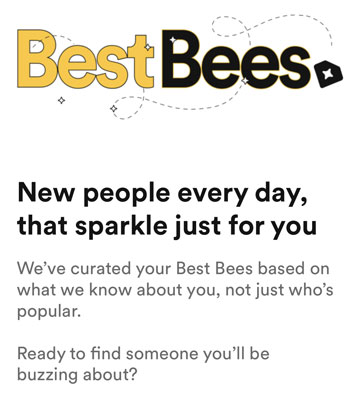
One of Bumble’s most advanced AI features is its “Best Bees” features, which provides a curated daily list of potential matches (Adams, 2025). Instead of randomly suggesting users, the AI selects these profiles based on detailed behavioral insights. If the algorithm notices that you tend to respond more to users with certain interests or communication styles, it prioritizes showing you people with similar traits. For example, if you regularly engage in longer conversations with people who have detailed bios rather than minimal profiles, the AI will adjust accordingly, showing you more users with “well-filled-out” descriptions (Adams, 2025).
Beyond matching, Bumble also integrates AI into safety and moderation, an increasingly important aspect of online dating. Jackson (2024) explained that the platform aims to refocus on the potential of AI and utilize its driven content moderation tools to scan for harassing or inappropriate content in messages in order to improve user experience. If an offensive message is detected, the AI will either flag it for review or warn the sender before they hit “send” (Jackson, 2024). In 2019, Bumble introduced “Private Detector“, an AI feature that automatically blurs explicit images and gives users the option to view or block them (Belloni, 2022). Belloni (2022) pointed out that this AI-driven approach ensures that the app fosters a safer and more respectful dating environment, differentiating it from competitors that rely heavily on user-reported complaints.
One of the most fascinating aspects of Bumble’s AI-driven personalization is its ability to adapt in real-time. According to the video from Forbes (2021) interviewed Whitney Wolfe Herd (the founder of Bumble), she said that the video call function had been launched in the early days of the COVID-19 pandemic. Bumble observed that dating behavior had changed, and more and more users participated in and popularized virtual dating, giving priority to “their own safety and the opportunity for users to meet in advance for validation” (Forbes, 2021). In response, the platform adjusted its algorithm to highlight users who were open to video calls and long-distance connections, making it easier for people to find matches who shared their dating preferences during lockdowns. This level of adaptability showcases how AI can shape not just who we match with, but also how we experience online dating itself.
What Bumble’s Algorithm Says About Us—and When Love Meets the Algorithm
At the end of the day, maybe love and machine learning make a strange pair—but in 2025, they’re already in a committed relationship. Apps like Bumble aren’t just helping people find each other; they’re quietly learning what keeps us coming back, who we think we want, and even how we talk to each other.
We like to believe our romantic choices are entirely our own. That spark when someone’s profile just clicks, the dopamine hit when they reply with the perfect GIF—these moments feel spontaneous, emotional, even magical. But behind the magic is a machine: a carefully trained algorithm that, as Flew (2021) put it, is part of a much larger system where “the market for attention is increasingly being produced and allocated by applications that build on algorithmic selection” (p. 82). In other words, what catches your eye or your heart has already been sorted, filtered, and framed by a logic you never see.
The AI Truth About Dating
And it’s not just about what you like—it’s about what you do. Bumble’s algorithm isn’t trying to understand love the way a human would; it’s just trying to predict your next move. Swiped right on a cat-lover last week? Expect more golden retrievers. Paused a little longer on people who write long profiles? You’ll see fewer one-liners tomorrow. As Flew (2021) noted, algorithms evolve through repeated interaction, learning how to deliver what you’ll engage with by drawing from massive datasets of past behavior (p. 80). So the Bumble you use today isn’t static—it’s been tailored to your patterns, your moods, and even your hesitations.
This process turns the dating app into something more than just a digital playground. It becomes what Flew (2021) called a “human–algorithmic interface” that reshapes behavior itself (p. 85-86). Maybe you didn’t plan to swipe right on that profile, but the algorithm had a hunch you might. Maybe you weren’t looking for someone who lives 15 miles away—but your past data says you tend to message them back. This is the subtle power of platforms like Bumble: they don’t just respond to who we are—they guide who we become.
AI Cupid, in that sense, is less of a matchmaker and more of a mirror—reflecting us back to ourselves, processed through thousands of invisible calculations. So next time you get a match and think, “Wow, this person really gets me,” just remember: someone—or something—might’ve known that long before you did. Because in the age of algorithmic romance, love isn’t just what we feel. It’s also what we feed the AI.
References
Adams, G. (2025, March 13). What is Bumble Best Bees? Everything you need to know. Wrizzler. https://wrizzler.app/blog/bumble-best-bees/
Belloni, M. (2022, October 24). Bumble Inc open sources Private DetectorTM and makes another step towards a safer internet for women. Medium. https://medium.com/bumble-tech/bumble-inc-open-sources-private-detector-and-makes-another-step-towards-a-safer-internet-for-women-8e6cdb111d81
Bumble. (n.d.). Make the first move. Bumble. https://bumble.com/en-au/the-buzz/bumble-make-the-first-move
Eddington, S., & Buzzanell, P. M. (2020). Women First: Bumble™ as a model for managing online gendered conflict. In The Routledge Handbook of Gender and Communication (pp. 427-445). Routledge.
Flew, T. (2021). Algorithms. In Regulating Platforms (pp. 79–86). Polity.
Forbes. (2021, February 11). Bumble founder discusses how COVID-19 pandemic affected app usage [Video]. YouTube. https://www.youtube.com/watch?v=ROjPYovQ2LE
Gadgets 360. (2020, December 13). How dating apps work: The algorithm behind Tinder, Bumble, OkCupid explained | Elemental EP 16 [Video]. YouTube. https://www.youtube.com/watch?v=x0gjLdWgHDg
Hinge. (n.d.). Hinge: The dating app designed to be deleted. https://hinge.co/
Hinge. (n.d.). What is We Met? Hinge Help Center. https://help.hinge.co/hc/en-us/articles/360010692913-What-is-We-Met
Jackson, A. (2024, March 24). Bumble: Harnessing AI to Power Human Relationships. AI Magazine. https://aimagazine.com/data-and-analytics/bumble-harnessing-ai-to-power-human-relationships
Jena, A. (2022, April 11). What is a Product Recommendation Engine and How Does it Work? Muvi. https://www.muvi.com/blogs/product-recommendation-engine/
Just, N., & Latzer, M. (2017). Governance by algorithms: reality construction by algorithmic selection on the Internet. Media Culture & Society, 39(2), 238–258. https://doi.org/10.1177/0163443716643157
Levesley, D. (2019, April 19). Hinge app we met: how Hinge makes sure dates turn into something more | British GQ. British GQ. https://www.gq-magazine.co.uk/article/hinge-app-we-met
Lokanan, M. E. (2023). The tinder swindler: Analyzing public sentiments of romance fraud using machine learning and artificial intelligence. Journal of Economic Criminology, 2, 100023.
Thorat, P. B., Goudar, R. M., & Barve, S. (2015). Survey on collaborative filtering, content-based filtering and hybrid recommendation system. International Journal of Computer Applications, 110(4).
Be the first to comment