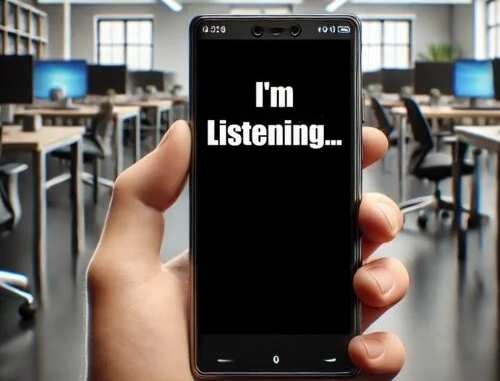
Figure 1: Image depicting how AI is listening. From AI Privacy: 6 Ways To Secure Your Organization from AI Data Leaks, 2024, LMG Security Blog. (https://www.lmgsecurity.com/ai-privacy-6-ways-to-secure-your-organization-from-ai-data-leaks/)
The Silent Observer or an Active Listener?
Imaging that you are scrolling TikTok or any other algorithm-fed digital media, and coming across a video that suggests a skincare routine tailored to your current skin condition. It’s as if someone is spying on you because you didn’t ask for it, but it’s actually there waiting for you. The elusive strength of AI-powered algorithm is apparent. These systems do not merely sit idle anymore; they actively record what we do and how we interact with online contents. Their predictive power is able to accurately speculate about our preferences and current moods, subtly guiding us in desired directions.
While it all seems to be driven by some unseen force, reality paints a more complex picture involving data, computation, and, to some extent, manipulation. When these AI-powered systems become the arbiters of political debates, cultural movements, or even issues related to mental well-being, who is exactly in control of everything under the surface? This is precisely where the governance of AI comes into play. There is no more fitting example to illustrate this issue than the controversial incident of TikTok Congressional hearings in the United States. TikTok CEO Shou Zi Chew underwent intense questioning regarding content moderation, connections to the Chinese government, and data strategies. The focus of the scrutiny is much broader than just TikTok; the fight is over the control of AI and the mechanisms for enforcing technological compliance.
Do We Understand AI Governance?
Before we go deep into the case, it is necessary to clarify one concept: AI governance. Simply put, the concept of AI governance is like parenting a genius but rebellious child. Much like disciplining the reckless behavior of gifted children, AI governance means limiting and guiding the robust systems created by humans, which are consistently evolving. Actually, it is one of the most prominent issues requiring political and ethical intervention today, which contains the efforts to control the development and use of artificial intelligence to ensure they are ethical, safe, and responsible to society (De Almeida, dos Santos, & Farias, 2021). Easier said than done but still a very important aspect.
From a policy standpoint, AI governance raises four critical issues:
- What to govern? This encompasses more than just the algorithms in question; it also includes the data that train them, the platforms that run them, and the participants that gain their benefits. It considers every decision in the AI, from data collection to model deployment.
- Who governs? Governments, enterprises, international organizations, and users themselves all have an impact on governance. Different stakeholders have different interests and perspectives, and the distribution of governance rights is a process full of competition (Gorwa, 2019).
- How to govern? A combination of government regulations, industry benchmarks, ethical frameworks, technological measures, and sometimes public pressure.
- Govern towards what? Ideally, AI governance intends to build a digital society that is more equitable and transparent, with AI that promotes well-being rather than reduces it. The vision aims to protect privacy, maintain inclusiveness, and foster trust.
According to Flew (2021), platform governance has eroded government authority by transferring regulatory power to technology corporations, creating a gap that requires urgent governments’ attention. This is magnified in the context of artificial intelligence, as the decision logic is obscure and the risk of escalating harm. Besides, Crawford (2021) further explains deeper dimensions of the influence of AI, noting that the implications not only lie in inadequate datasets and false narratives, but in the economic and material exploitation that sustain its infrastructure. Both the exploitation of labour and the environmental decay resulting from enormous data centers are directly related to who bears the cost and the extent of damage caused in the course of technology development.
In the final analysis, AI systems are embedded in biases from historical data, as well as subjective choices and values of their designers. The absence of systematic accountability would probably enable AI to evolve into complex tools that exacerbate social inequality and intensify social and political conflicts. A relevant case would then be analyzed to further clarify these problems.
A Case Study in AI Governance: the TikTok Hearings
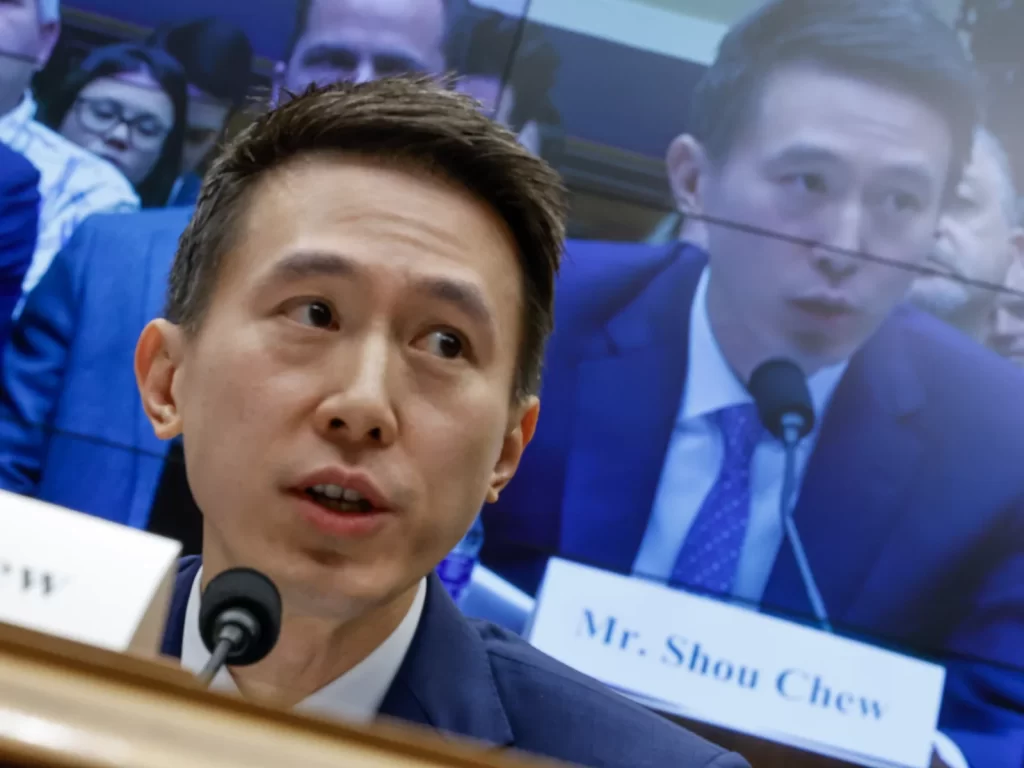
Hearing in the U.S. Congress in March 2023 is like a carefully choreographed political drama. The atmosphere was tense and there were several fierce debates, which was different from the usual legislative process. TikTok has been accused of being a weapon for Chinese intelligence services to monitor American teenagers, and has also been criticized for failing to effectively prevent platforms from spreading dangerous contents. This hearing not only focuses on the operational issues of TikTok itself, but also places it in the broader context of AI governance and geopolitical competition, highlighting the complexity and urgency of pertinent issues.
This case vividly exhibits many deep-seated problems currently confronted with AI governance:
- Data territoriality: One of the core disputes at the hearing is the issue of data ownership and security –– who can access the data, and where are they kept? Congress was worried about the possibility of ByteDance, TikTok’s parent company, getting access to American users’ data and having to share them with Chinese government, which has pushed TikTok in the teeth of the storm of the global debate on data privacy and national security. In response, TikTok launched “Project Texas”, promising to store and manage data of American users in the United States by a local company.
- Algorithmic opacity: The recommendation system on TikTok might be one of the most advanced content engines globally, but only insiders understand how it works. It’s perilous because when the decision logic is kept away from the public, the potential social impact –– from shaping users’ cognition to influencing their mental health, and even interfering with their political leanings –– may imperceptibly run out of control. Although lawmakers kept requiring TikTok to disclose more details about its algorithm, TikTok has repeatedly defended itself by using reasons like commercial secrets. The blurriness of its responses failed to meet the demands of legislators.
- Platform responsibility: Who is at fault for the viral spread of unhealthy trends or disinformation? The standard answer of TikTok is commitment to enhancing the content review and improving the technology for detection. However, this approach of relying on technology and strengthening internal management has been described by some observes as “platform paternalism” –– as pointed by Register, Qin, Baughan, and Spiro (2023), this is more of appeasement rather than structural change. Critics hold the belief that this precisely reflects the limitations of self-regulation by platform and calls for more powerful external intervention.
Although TikTok wasn’t subject to immediate ban or major policy shift after the intense inquiries by Congress, this hearing undoubtedly warned the existing regulation mode: relying solely on corporate self-discipline or government supervision seems insufficient to deal with the implications brought by global technology platforms with effect. It has successfully exposed the profound political and moral issues behind the tech giants to the public, sparking continuous attention and debates.
Additionally, this disturbance also indicates that such problems require multi-faced approaches involving international cooperation, dialogue from different sectors, and inclusive policy-making, as no single nation or organization can manage the risks alone. TikTok incident actually reveals the common dilemma in managing emerging technologies: how to achieve a balance among national security, commercial freedom, freedom of speech, and user protection. Understanding this contradiction is to grasp the key to why we must attach great significance to and make in-depth explorations on the governance of AI.
Beyond TikTok Hearings: Why AI Governance Matters?
TikTok hearings is just the tip of the iceberg. The problems it has exposed show the importance of AI governance and how it impacts people’s lives in multiple aspects:
- Economic control: Nowadays, automated decision-making systems are infiltrating every aspect of our economic life, deciding what services we can obtain, what products we can purchase, and what kind of jobs we can find. More alarmingly, the number of companies mastering these powerful AI technologies is decreasing, and the power is increasingly concentrated in a few tech giants. As Crawford (2021) argues, these companies profit not only from the software of AI but also from the control over infrastructure and labour. This highlights the significance of AI governance: we need effective rules to ensure fair competitive environment and prevent the control of key digital technologies from changing to new economic monopolies, thus safeguarding everyone’s opportunities to participate in economy.
- Social impact: AI systems influence the public’s agenda. Facebook has been accused of having politically divisive algorithms. YouTube’s algorithm is charged with radicalization. If go into the reasons, this often stems from the profit-oriented strategies of platforms: AI models are designed to deliver contents that can best capture users’ attention first, while anger, disputes, and false information tend to be the most eye-catching. The final product is an environment where society’s divisions can be worse made by algorithms, and has the power to shape powerful social and political movements. Therefore, there is an urgent need for establishing relevant rules to regulate the operation of content recommendation systems, guaranteeing that they can realize commercial benefits while also taking into account social responsibility and public welfare.
- Technical complexity: Most AI is perceived as a black box. Even the developers may not know the reason behind an algorithm’s decision. This creates countless challenges for providing accountability due to a lack of investment in transparency and interpretability (Von Eschenbach, 2021). Consider the example of an AI-powered hiring system. Who can be held liable if it biases and discriminates against a particular group of candidates? Without proactive oversight, systems can perpetually operate with ingrained biases and make harmful decisions unchecked.
- Environmental impact: AI governance should not only consider the social and ethical hazards, but also take into account the ecological damage in the advancement. Actually, the development of AI itself is resource-intensive. Crawford (2023) describes it as the “extracting” of natural resources: training complicated AI models like GPT-3 or DALL· E would cost mountains of electricity and computing power. At the same time, the colossal data centers that support the operation of these systems have left massive carbon footprint. However, environmental costs are often selectively forgotten in the heated discussions on the potential of AI, while funds keep pouring in. It’s obliged to value environmental sustainability, which is absolutely a critical task of AI governance.
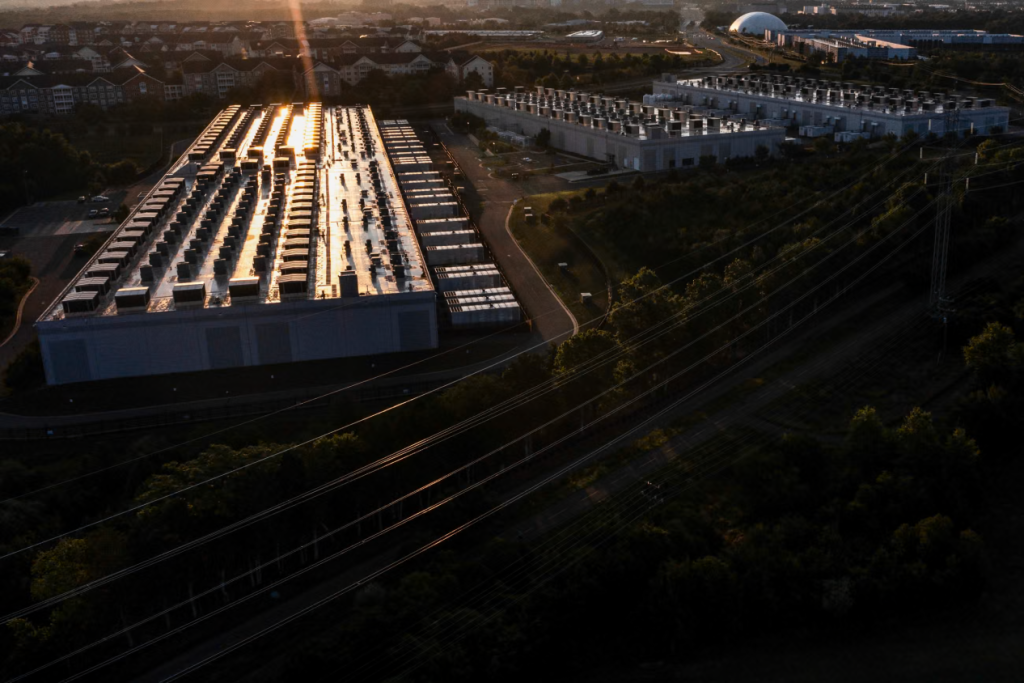
Is Governing the Beast Possible?
There is no one-size-fits-all solution, but here are some proposals to consider:
- Stricter regulation: The European Union is working to implement the AI Act; these places risk classification on AI systems and more severe restrictions on the highest-risk applications. In the U.S., there is no overarching structure, but the Federal Trade Commission and other entities are beginning to act. Proposed federal privacy legislation seeks to empower users with greater control over their own data.
- Transparency requirement: External review of algorithms should be allowed, and explanations on decision logic should also be required, along with the disclosure of the source of training data. Researchers are supposed to have access rights, which is able to validly assess the potential social harms that AI may cause. Similar to environmental impact assessment, this can also be viewed as algorithmic impact assessments. The more transparent AI systems are, the more the public and regulators can hold companies accountable.
- Technological restrictions: It’s important for the users and the firms to strive towards explanatory AI and bias mitigation as well as decentralized variants of data governance systems. Trust and safety can be advanced through open-source models and independent evaluations (Li et al., 2023).
- Public empowerment: It’s essential to inform users about the nature of algorithms and give them choices like allowing them to turn off the function of “you may also like”, choose a browsing mode of non-algorithmic sorting, or adopt stricter privacy protection. To this, Sharma and Singh (2024) deduce that digital literacy ought to be a fundamental component of education curricula in the 21st century.
- Global cooperation: AI governance should not be limited to a country’s borders. Considering that data circulation patterns, the global reach of platforms, and their environmental consequences are all universal concerns, international collaboration through organizations like OECD and UN matters. Without clear international standards, enterprises would leverage gaps where regulations are more lenient, escaping stricter rules imposed elsewhere.
Conclusion:
AI is no longer simply a passive tool; it is shaping the economy, society, and environment. TikTok hearings exhibit more profound difficulties in AI governance: the lack of transparency, corporate centralism, and ecological indifference. Although there is increasing emphasis on policies, there is still fragmentation in the governance architecture that is imposed, passive, and bound to the territory of a nation, as all lag behind the cross-country AI system. A global approach is necessary, and it must be coherent, ethical, and enforceable. Without these guidelines, AI would probably continue to enhance inequality, disinformation, and environmental destruction disguised as innovation.
References
Cabrera, K., & Saldana, S. (2023, March 27). Project Texas: Inside TikTok’s billion-dollar plan to stay in America. Texas Standard. https://www.texasstandard.org/stories/project-texas-tiktok-plan-stay-america-oracle-security/
Crawford, K. (2023). Mining for data: The extractive economy behind AI. Green European Journal. https://www.greeneuropeanjournal.eu/mining-for-data-the-extractive-economy-behind-ai/
Crawford, K. (2021). The atlas of AI: Power, politics, and the planetary costs of artificial intelligence. Yale University Press.
De Almeida, P. G. R., dos Santos, C. D., & Farias, J. S. (2021). Artificial intelligence regulation: A framework for governance. Ethics and Information Technology, 23, 505–525. https://doi.org/10.1007/s10676-021-09593-z
ET CIO.com. (2024, October 3). Data centre emissions are soaring – it’s AI or climate. ET CIO. https://cio.economictimes.indiatimes.com/news/data-center/data-centre-emissions-are-soaring-its-ai-or-climate/113915291
Flew, T. (2021). Issues of concern. In T. Flew, Regulating platforms (pp. 79–86). Polity.
Gorwa, R. (2019). What is platform governance? Information, Communication & Society, 22(6), 854–871. https://doi.org/10.1080/1369118X.2019.1573914
High-level summary of the AI Act. (2024, February 27). EU Artificial Intelligence Act. https://artificialintelligenceact.eu/high-level-summary/
Kerr, D. (2023, March 23). Lawmakers grilled TikTok CEO Chew for 5 hours in a high-stakes hearing about the app. NPR. https://www.npr.org/2023/03/23/1165579717/tiktok-congress-hearing-shou-zi-chew-project-texas
Li, B., Qi, P., Liu, B., Di, S., Liu, J., Pei, J., Yi, J., & Zhou, B. (2023). Trustworthy AI: From principles to practices. ACM Computing Surveys, 55(9), 1–46. https://doi.org/10.1145/3555803
LMG Security. (2024, September 26). AI privacy: 6 ways to secure your organization from AI data leaks. LMG Security Blog. https://www.lmgsecurity.com/ai-privacy-6-ways-to-secure-your-organization-from-ai-data-leaks/
O’Brien, I. (2024, September 16). Data center emissions probably 662% higher than big tech claims. Can it keep up the ruse? The Guardian. https://www.theguardian.com/technology/2024/sep/15/data-center-gas-emissions-tech
Register, Y., Qin, L., Baughan, A., & Spiro, E. S. (2023, April). Attached to “the algorithm”: Making sense of algorithmic precarity on Instagram. In Proceedings of the 2023 CHI Conference on Human Factors in Computing Systems (pp. 1–15). https://doi.org/10.1145/3544548.3581257
Roose, K. (2019, March 29). YouTube’s product chief on online radicalization and algorithmic rabbit holes. The New York Times. https://www.nytimes.com/2019/03/29/technology/youtube-online-extremism.html
Sharma, A., & Singh, A. (2024). Digital literacy: An essential life skill in present era of education. In Transforming learning: The power of educational technology (pp. 118–125). Blue Rose Publishers.
Srivastava, N. (2022, May 30). Facebook and the unconscionability of outrage algorithms. Lens Monash. https://lens.monash.edu/@politics-society/2022/05/30/1384596/facebook-and-the-unconscionability-of-outrage-algorithms
Von Eschenbach, W. J. (2021). Transparency and the black box problem: Why we do not trust AI. Philosophy & Technology, 34(4), 1607–1622. https://doi.org/10.1007/s13347-021-00477-0
Be the first to comment