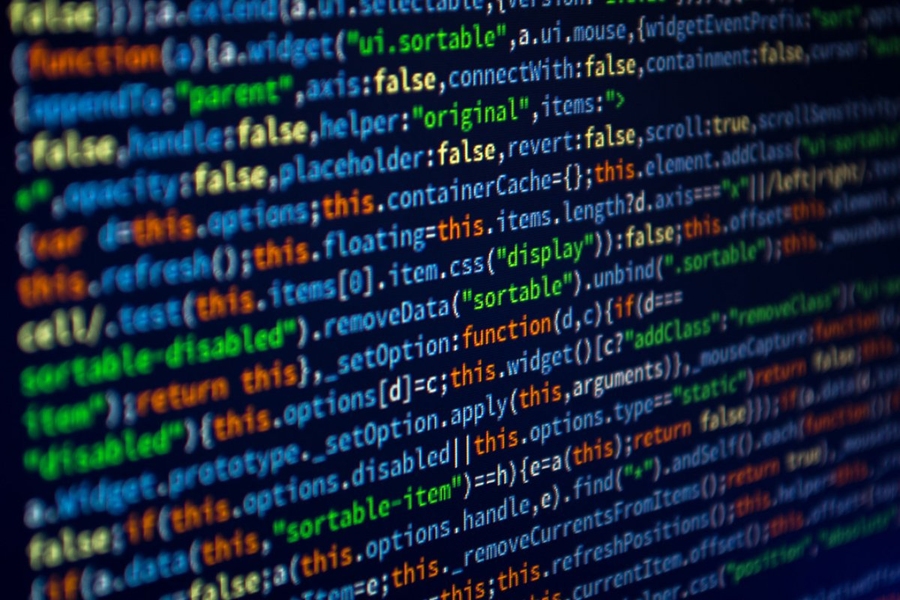
Algorithms and big data in the current highly dynamic context affect industries and shape society in the contemporary world. These technologies have drastically changed how business is conducted, people’s interaction with various forms of media and how global decisions are made (Siles & Hartmann, 2023). Platforms with recommendation systems that rely on algorithms are changing workplaces and are more and more involved in areas as diverse as entertainment and finance. Big data refers to the development of systems based on specific codes that analyse all human activities as data. However, they may generate new opportunities and new questions about privacy, power, and fairness (Siles & Hartmann, 2023).
Case Study 1: Netflix and the Power of Algorithms
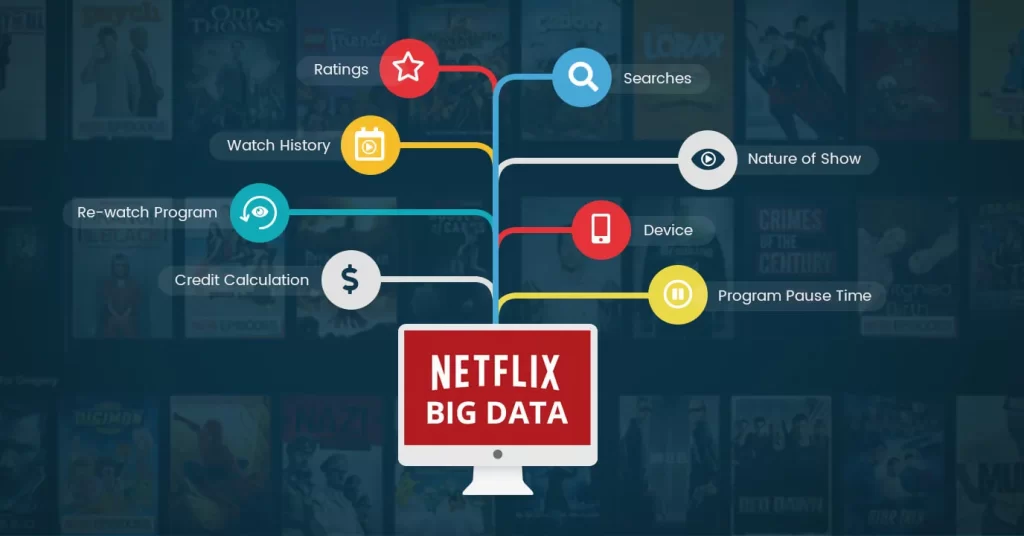
AI and algorithms have been talked about a lot in recent years. Just (2017) points out that AI is a system or machine that is capable of doing activities that may be done by intelligent humans. Different from AI, Algorithms are the rules or steps that guide how AI and automation work (Just, 2017). They tell the system how to handle data and how to deal with the information it receives.
In 2013, Netflix started using algorithms and big data to guide both recommendations and production decisions. It may be one of the most familiar examples of which algorithms and big data influence consumption. Big data refers to a trend to turn any aspect of people’s lives into data, including social networks, commerce, or health (Hallinan & Striphas, 2016). It is for this reason that analysis of this data is conducted to forecast behaviours, recognize trends and achieve better solutions. Through datafication, organizations are then able to tailor their products and present users themselves to make decisions. These approaches of recommending programs and films are the drivers of the platform’s effectiveness. Millions of users depend on Netflix to find new content based on their history (Williamson et al., 2023). When users open Netflix and enter their username and password, they are offered a list of recommended series and films of interest. This is made possible by the algorithms that consider not only your interests in your own viewing history but also similar users. On one hand, algorithms can give users a better experience. On the other hand, they may influenced by the business goals of the companies, which may lead to homogenisation and privacy crimes.
Following Hallinan and Striphas (2016), Netflix’s recommendations algorithm not only gives content but remains an active participant in producing algorithmic culture. Algorithms in Netflix may define what people perceive as popular, good, or relevant to their demands or preferences. For instance, it might suggest new releases based on the classes or actors a user usually prefers, which then feeds them more similar content in a cycle. Netflix may prevent users from coming across different types of shows or movies different from the ones they enjoy and thus becoming prone to only watch what they like most. Additionally, Hallinan and Striphas (2016) also outline that users could be re-identified, exposing risks of algorithmic profiling. For example, a lesbian user lawsuit against Netflix because of privacy leakage.
Due to the concern mentioned above, Siles and Hartmann (2023) distinguish between the concept of mutual domestication which refers to a co-creation of user and algorithm. Customers simply staring at the TV screen and scrolling through the series and movies can be shaped by algorithms without their knowledge. For instance, the users may start watching content that is consistent with the Netflix suggestion model, thereby exposing them to more generic content that Netflix often recommends. It also leads to positive feedback because the platform offers more similar choices, which reinforces a limited choice set.
Therefore, Netflix balances itself well between eliminating the middlemen and streamlining the process of finding good content, which leads toward a more homogeneous digital culture (Burroughs, 2019). The core concept of the recommendation system indicates that it is necessary to legalize algorithmic decision-making processes more strictly.
Case Study 2: Tiktok with the Algorithmic Economy
In the same way that repurchase works, the TikTok social network which has recently gained immense popularity has a recommender functionality that adapts content to the actions of its users (Williamson et al., 2023). The “For You” page is composed of short videos driven by one of the most developed recommendation algorithms currently available.
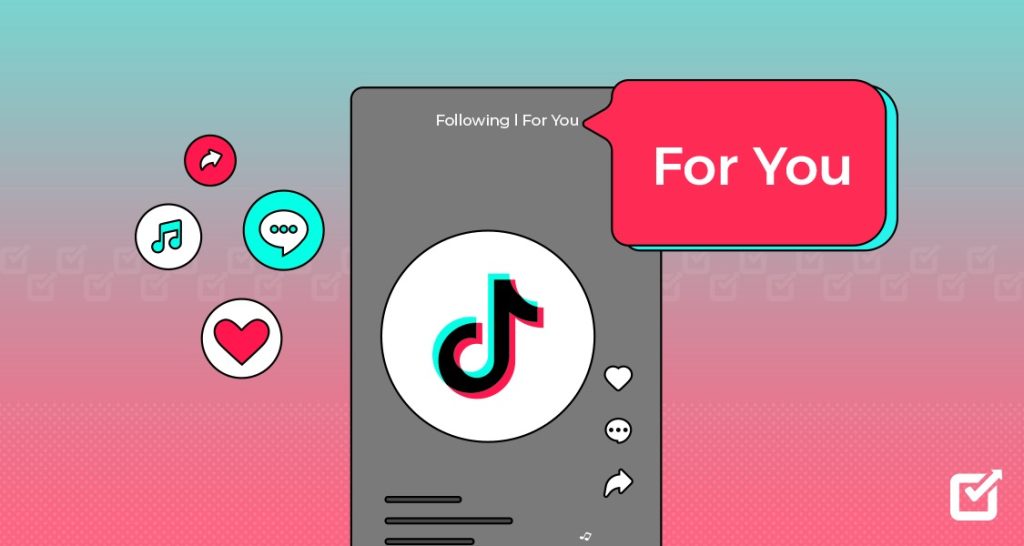
Therefore, TikTok uses its algorithm to retain user engagement for as long as possible upon recommendations based on how the users’ time and engagements are spent within the app, which may include likes, shares, and comments, among other features. This fusion brings several advantages. Firstly, it improves marketing efficiency by allowing businesses to reach potential customers more accurately. In addition, it improves user satisfaction and individual experience, as people are more likely to see content and products that cater to their demands. Lastly, it has real-time feedback. It allows companies to adjust their strategies quickly based on user behavior and trends. As a result, the integration of big data between TikTok’s business and video sections not only boosts commercial value but also strengthens user engagement (Crawford, 2021).
However, what Crawford (2021) says is that TikTok is also using an algorithm whereby users are manipulated through constant interaction. It provides preference to those videos that elicit strong feelings such as humorous, inflammatory, or emotional ones. This is because platforms can increase the amount of time people spend online by attracting users in this way which leads to more advertisement views and clicks. Although this approach can enhance the user interaction experience, it also raises concerns about its potential impact on users’ mental health (Lindgren, 2023). In particular, such design strategies may create addictive behaviors. Users—especially young people—can easily fall into a cycle of endless scrolling, constantly seeking the next piece of content that gives them a quick sense of pleasure or excitement, often linked to a dopamine response (Lindgren, 2023).
Another key process involved in the application is that of big data, TikTok’s business sector makes extensive use of big data to optimise marketing strategies and improve user engagement. TikTok has successfully integrated its business and video sections through the use of big data, creating a powerful and efficient digital ecosystem (Crawford, 2021). The platform can match advertisements and promotional content with the right audiences in a highly targeted way by analysing user data such as viewing habits, interaction patterns and content preferences. Additionally, in TikTok, content creators can attach links to the products they used in their videos below the video. This allows users to click the links and purchase the same items easily. In addition, when users click on a product, the platform will recommend similar types of products and help users compare prices and choose higher-quality options. This provides a convenient and personalized shopping experience.
However, these developments raise several important questions about privacy and surveillance. Platforms like TikTok and Netflix with billions of active users have become powerful authorities in shaping people’s consumption such as the choices they make and even their emotions. This highlights the issue of information concentration, which calls for proper regulation to protect the personal data collected by digital platforms.
Privacy and the Algorithmic Gaze
Because of algorism and datafication, the digital space becomes personalized and feels unique for each user. However, this kind of personalization also brings some negative effects. On both platforms, the power of algorithms is very strong. More limits may generated if more people’s experiences are analyzed by the algorithm. This means the person may only see certain types of content or ideas, leading to a biased experience (Crawford, 2021). As a result, users can become trapped in a closed space that the algorithm creates. This is a significant problem because it may be harder for people to access different views or types of information.
The platforms that collect and use people’s personal information have raised serious privacy concerns (Lavazza & Farina, 2023). Most users don’t know what data is being collected or how it is used. For example, TikTok may track how long you watch a video and what you like or share. This information helps the platform improve its algorithm so it can suggest more content that keeps you watching. However, these platforms could become a big threat to personal privacy unless stronger rules are put in place and users are given more control over their data. Users have the right to see what data is being collected . This problem becomes even worse because there is often a lack of clear laws or strong government regulations around data collection. Without this, companies can gather and use a lot of information without telling the user. This makes it easy to combine, share, or even misuse personal data in ways that users do not expect or approve of.
We also need to focus on fairness in algorithms. Machine learning algorithms have bias. Since these algorithms learn from data, they also absorb the biases within that data. Therefore, they may regenerate these biases, which can lead to unfair treatment of people (Andrejevic, 2019). The problem of algorithmic bias also involves how these systems can reinforce stereotypes or exclude important voices and identities. This creates limitations in how fairly and accurately the digital world represents different communities.
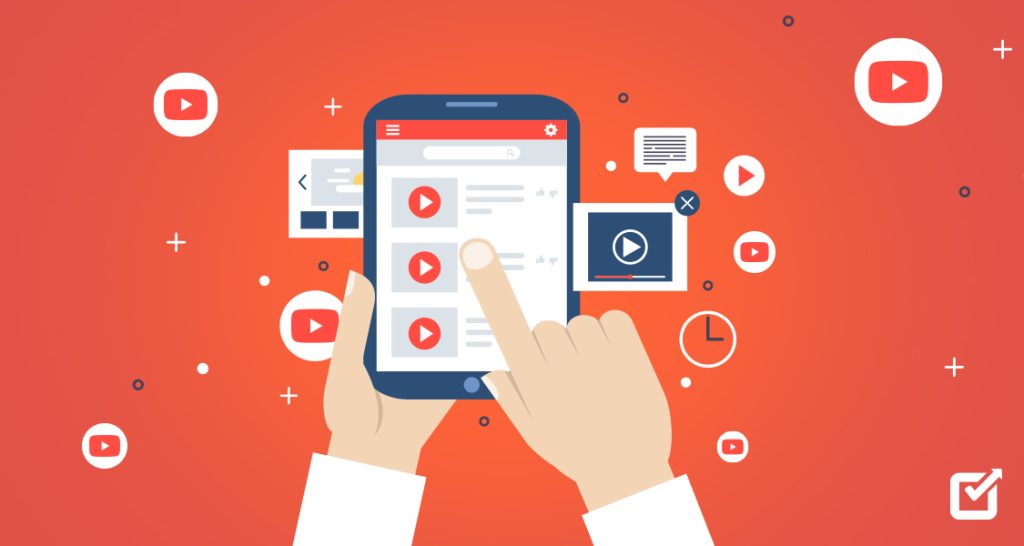
Policy Implications: The Need for Digital Governance
Considering the increasing use of algorithms and datafication as guides for our digital experiences makes digital governance a key necessity. While technology continues to impress world leaders and organizations with innovative products and services that improve living standards in many communities, it also brings both known and unknown risks that may require regulation by governments and other authorities (Hallinan & Striphas, 2016). One of the main areas that should be carefully considered is transparency. Social media platforms should be required to explain how their systems work, what kinds of data they collect from users, and how that data is handled. This would help ensure that users can manage their online activities more effectively and that digital platforms are held accountable for their actions.
There is still a strong need for stricter regulation in the privacy aspects. It is essential to assess new regulations that not only restrict the excessive collection of personal data by companies and digital services but also empower users with greater control and ownership over their own information. Furthermore, this situation emphasizes the urgent need for ethical frameworks to guide the development and application of algorithms. These frameworks should be fairness, transparency, accountability, and protect personal data. We can improve social equality by inserting these values into the design and deployment of Algorithm systems. Digitalization can become a tool to create well-being for all members of society (Calzada, 2024).
Conclusion: The Future of AI and Digital Governance
Looking into the future, such concepts as algorithms and datafication will impact our digital experience even more. Netflix and TikTok represent both the positive and negative uses of these technologies. Although customer reward and brand loyalty can be fun and tailored, such programs can be problematic in terms of power relations, privacy, and equality. For these technologies to be implemented and used appropriately, there is a need to develop sound governance structures. Governance should also give caste on innovativeness to ensure that we form a digital space that is worth owning by everybody without reinforcing powers over others or endangering individual liberties.
References
Andrejevic, M. (2019). Automated Media. Routledge. https://doi.org/10.4324/9780429242595
Burroughs, B. (2019). House of Netflix: Streaming media and digital lore. Popular Communication, 17(1), 1–17. https://doi.org/10.1080/15405702.2017.1343948
Crawford, K. (2021). The Atlas of AI: Power, Politics, and the Planetary Costs of Artificial Intelligence (1st ed.). Yale University Press. https://ebookcentral.proquest.com/lib/usyd/reader.action?docID=6478659&ppg=1
Calzada, I. (2024). Artificial Intelligence for Social Innovation: Beyond the Noise of Algorithms and Datafication. Sustainability, 16(19), 8638-. https://doi.org/10.3390/su16198638
Just, N., & Latzer, M. (2017). Governance by algorithms: reality construction by algorithmic selection on the Internet. Media, Culture & Society, 39(2), 238–258. https://journals.sagepub.com/doi/full/10.1177/0163443716643157
Hallinan, B., & Striphas, T. (2016). Recommended for you: The Netflix Prize and the production of algorithmic culture. New Media & Society, 18(1), 117–137. https://doi.org/10.1177/1461444814538646
Lavazza, A., & Farina, M. (2023). Infosphere, Datafication, and Decision-Making Processes in the AI Era. Topoi, 42(3), 843–856. https://doi.org/10.1007/s11245-023-09919-0
Lindgren, S. (2023). Introducing critical studies of artificial intelligence. In Handbook of Critical Studies of Artificial Intelligence (pp. 1–19). Edward Elgar Publishing. https://doi.org/10.4337/9781803928562.00005
Siles, I., & Hartmann, M. (2023). The mutual domestication of users and algorithms: The case of Netflix. In The Routledge Handbook of Media and Technology Domestication (1st ed., pp. 235–248). Routledge. https://doi.org/10.4324/9781003265931-23
Williamson, B., Macgilchrist, F., & Potter, J. (2023). Re-examining AI, automation and datafication in education. Learning, Media and Technology, 48(1), 1–5. https://doi.org/10.1080/17439884.2023.2167830
Be the first to comment