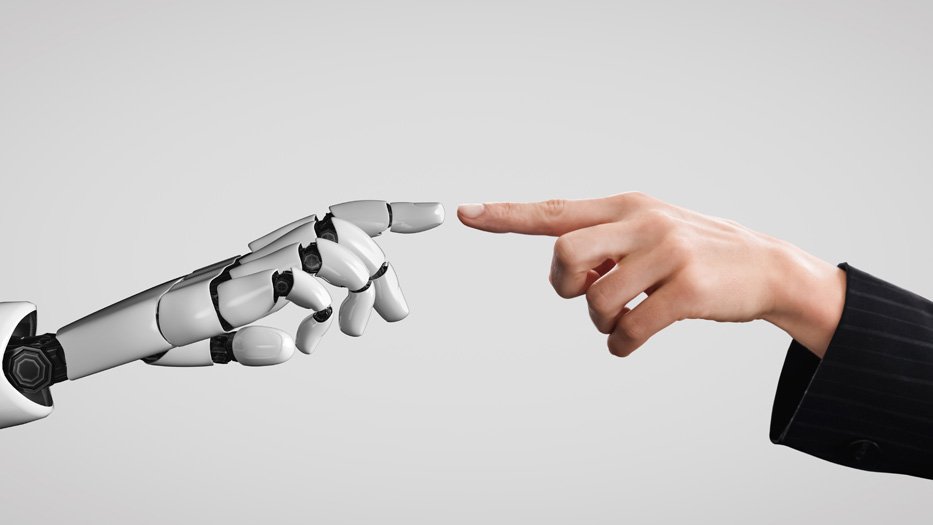
Artificial intelligence (AI) has inevitably entered our everyday lives. I still remember how shocked I was by its capabilities and potential when I first used it. Over time, AI has expanded its use into diverse fields, including travelling, law enforcement, policing, welfare, job recruitment, and even the military. Witnessing its rapid growth fills me with amazement but concerns at the same time—is AI magical enough to solve all our problems?
The answer is NO.
Artificial intelligence systems are inherently biased and shaped by existing human values and power structures, which can lead to harmful decisions and advice. Therefore, AI governance needs to adopt a people-centred approach to prioritise individual needs and create a more transparent and trustworthy framework for the use of AI.
For real, what even is AI?
AI is designed to learn and evolve from data to think, solve problems and make decisions similarly to humans. Many people rely on AI as their personal assistants to release their workload, find answers, interpret information, etc. However, Crawford (2021) argues in her book that “AI is neither artificial nor intelligent” (p.8). What she means is that AI systems run on natural resources, human labour, historical context and infrastructure, yet they cannot reason, feel or understand any meaning. Instead, they are merely detecting patterns and providing responses based on human-trained data (Crawford, 2021). So don’t be fooled, AI can not give us the best possible solution.
Algorithm: the secret hands that control AI
We might think that AI is able to make responses on its own, but they are actually powered by a web of invisible instructions – algorithms. This could be the hidden manipulating hands behind each system to control what we see, read, and believe, a process called algorithmic selection (Flew, 2021). You might find that platforms like Google, YouTube and Facebook always recommend content that matches your interests and thoughts; this is because one of the main goals of algorithmic selection is to generate personalised content. These platforms access to your social media, searching history, likes, and even locations to select content shown on your screen. You will then continue receiving these filtered contents that the system deems matters, popular, and relevant, blocking you from opposing news and information (Flew, 2021). Eventually, your views of the world, beliefs, and opinions start to shift and be shaped simply through the showing content without you knowing it.
That’s why algorithms are not neutral tools. They are built and controlled by people with specific goals, assumptions, judgements, and blind spots, making them unreliable and unaccountable. When algorithms have the capacity to decide what’s valued and visible based on the number of clicks and views, they are likely to reflect and reinforce the social injustice and inequalities rather than challenge them (Nobel, 2018). AI operated through algorithm is then not fair, but politics in disguise.
The Black Box Model
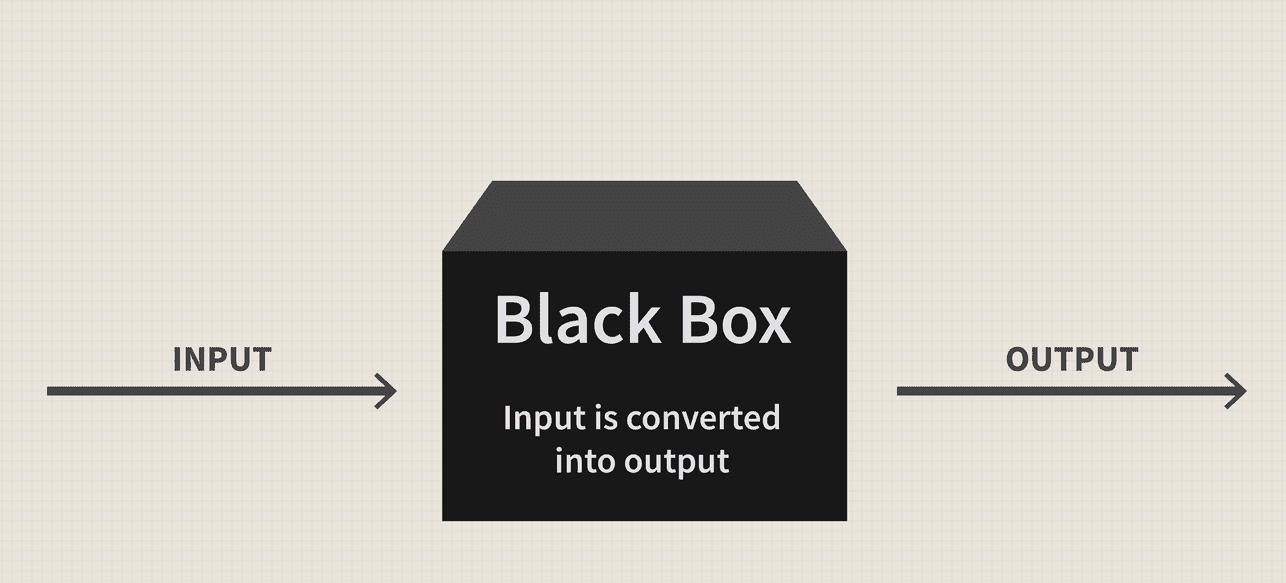
AI systems are concerned with another factor: its black box model. The input, throughput, and output process (refer to data collected, processed by algorithms and the final contents) is non-transparent (Just & Latzer, 2019). Many decisions that happened in this ‘black box’ have no explanation of how and why these results are made; even the developers have no clue. Trusting the black box model means putting trust in the entire database it has built on, which may include incorrect, biased and unverified data (Rudin & Radin, 2019). The lack of transparency makes it impossible to challenge the accuracy of the outputs and may bring serious consequences to society as AI systems are now integrated into many fields (Flew, 2021).
For instance, the COMPAS system is used in American criminal justice systems to predict the likeliness of someone reoffending. Investigations have revealed that black defendants are twice as likely to be falsely labelled as high-risk compared to white defendants, even when they don’t reoffend (Rudin & Radin, 2019). This example reveals the hidden biases behind these algorithms, and confirms that AI is far away from being neutral and accountable. People who have been mistakenly labelled could not appeal the decision due to limited access to the systems’ inner working process. So who should they go to? Who is responsible for these wrong decisions?
If the data is biased, AI will replicate the bias

AI learns and evolves through identifying patterns within data. Both machine learning and deep learning are based on huge datasets that the developers feed to the system (Flew, 2021). For example, suppose you input AI with hundreds of student essays and tell it which ones received high marks. In that case, AI will analyse these examples to discern patterns and learn to predict which ones are likely to get similar marks. This process raised a significant concern: AI has an inherently biased nature; it learns and grows from existing human preferences and biases.
AI nowadays has access to an immense amount of information online, including videos, books, personal information, comments, and search history. However, not all the information is neutral, and AI will inevitably absorb and remember the biases in it. The more people engage and agree with certain contents, the more the algorithm promotes and reinforces it, creating a vicious feedback loop that perpetuating this unfairness (Just & Latzer, 2019).
Chen’s research (2021) clearly demonstrated that AI recruitment tools contain biases related to race, gender and colour. The results points out that primary sources of these algorithmic biases are rooted in the historical dataset and personal preferences of algorithm engineers (Chen, 2021). This study further emphasises how AI can amplify systemic discrimination under the illusion of being an ‘efficient tool’. This leaves us questioning whether these systems made problems unsolved or even more challenging to solve due to their lack of transparency and complexity.
When Efficiency Turns Harmful
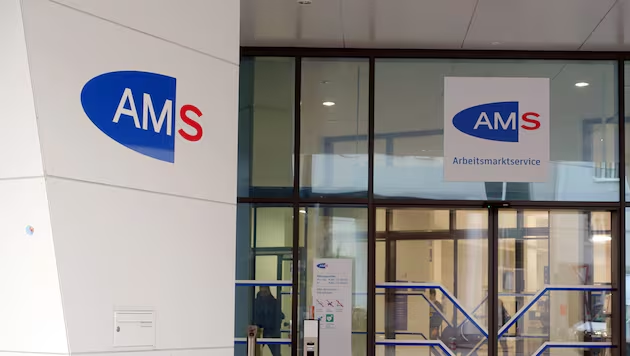
Let’s have a look at a real-life case study that reveals AI’s hidden discriminatory nature: the Austrian Public Employment Service (AMS) algorithm. This system was designed to predict an individual’s likelihood of finding jobs and to provide differing support. The system classifies job seekers into three categories: high, medium, and low employment prospects, to offer according support for re-entering the labour market. You may think that sounds quite useful in terms of helping the job seekers. However, in 2020, the AMS algorithm sparked widespread public debate over its discriminatory outcomes, misinterpretations, and potential to amplify existing inequalities in the labour market (Allhutter et al., 2020).
Like all algorithmic systems, the AMS algorithm relies heavily on data. Yet, no data were specifically collected to train the system. Instead, it uses profiling information such as a person’s age, gender, obligation of care, and employment history to assess their employability. Importantly, Allhutter et al. (2020) highlight that while the system has access to valuable data—like employer hiring behaviour and job openings—these are not included in the model, which means the algorithm focuses only on individual traits rather than broader market dynamics.
As a result, pre-existing inequalities in the job market are embedded into the system and passed forward. The study found that women (especially mothers), older individuals, those with lower education, and people with migration backgrounds were more likely to be placed in the “low prospects” group (Group C), receiving the least employment support (Allhutter et al., 2020). This finding raises the question: if those with the most barriers are given the least help, what is the point of the system for marginalized people?
The system defines “employability” as a fixed set of personal characteristics—many of which are difficult or impossible for job seekers to change (Allhutter et al., 2020). What’s even more troubling is that affected individuals have no opportunity to appeal or challenge the score they’ve been assigned due to the lack of transparency. In essence, the AMS algorithm doesn’t just reflect inequality—it helps to automate and justify it.
Towards a people-centred governance approach
After all we have discussed, I wish to express the message that AI must be governed. AI systems must shift from tech solutionism to a more human-centred approach. What Lomborg et al. (2023) suggested in their paper is that AI governance should put people’s needs, rights, and experiences at the heart of AI systems to promote inclusivity and justice in this digital society. The people-centred approach focuses on listening to the voices of the public and individuals, especially groups of minorities, to be actively involved in testing, designing and training AI systems to create a positive feedback loop. In this way, AI, algorithms, and automation decision-making systems will have the potential to challenge or reshape the existing biases and to assist people based on diverse contexts (Sigfrids et al., 2023). They are no longer calculations and numbers but intelligent partners that work alongside humans to build a better future.
To shift our current governance to human-centred model, governments and related departments need to set boundaries and regulatory frameworks to foster accountability and transparency of AI use (Flew, 2021). With these regulations, companies will start designing systems that serve human rights, social justice, and public interest instead of being profit-driven (efficient and profitable). Many experts argue in their study that AI governance should not just be technical guidelines, but grounded in legal protection and inclusive participation of users (Flew, 2021; Lomborg et al., 2023).

In fact, many countries have realised the importance of reforming AI governance and started taking action. For instance, Personal Data Protection Commission Singapore has released a new edition of model AI governance framework that focuses on transparency, inclusivity, accountability and fairness of AI use. This framework’s principles allow users to provide feedback and promise to minimise biases and harm to individuals (PDPC, 2020). By focusing on human experiences, we move closer to a better digital future.
A Better Future Must Be a Fairer One
Ten years ago, people were largely unfamiliar with artificial intelligence, and they only existed in movies and fantasy novels. We had no idea how quickly and impressively technology would develop. AI is no longer a futuristic concept, but embedded everywhere in our daily lives. Imagine a future where all self-driving cars on the roads, AI systems handle our jobs, and children learn from everything digital. Would this create a better world?
AI has significant potential to either perpetuate or exacerbate existing inequalities and discrimination across various sectors, including policing, healthcare, banking, recruitment, and government programs. The question remains: are these systems designed to help advance society or to regress it? That’s why we need to start viewing AI as a powerful tool that needs our critical thinking; not everything it says is true, neutral or helpful. It can cause harmful outcomes with no accountabilities. As Flew (2021) mentioned in his book, technology companies can easily avoid their responsibilities by stating that they are just providing the ‘service’.
If we want to build a fairer, more inclusive digital future with AI, we need stronger regulations, increased human participation, and enhanced transparency. A human-centred approach would ensure that the most affected or discriminated users against by AI have their voice in developing these systems (Lomborg et al., 2023). Technology should serve people, not the other way around. We must advocate for change to earn a better future.
References:
Allhutter, D., Cech, F., Fischer, F., Grill, G., & Mager, A. (2020). Algorithmic Profiling of Job Seekers in Austria: How Austerity Politics Are Made Effective. Frontiers in Big Data, 3, 5-. https:// doi.org/10.3389/fdata.2020.00005
Chen, Z. (2023). Ethics and discrimination in artificial intelligence-enabled recruitment practices. Humanities & Social Sciences Communications, 10(1), 567–12. https://doi.org/10.1057/s41599-023-02079-x
Crawford, Kate (2021) The Atlas of AI: Power, Politics, and the Planetary Costs of Artificial Intelligence. New Haven, CT: Yale University Press.
Cynthia Rudin, & Joanna Radin. (2019). Why Are We Using Black Box Models in AI When We Don’t Need To? A Lesson From An Explainable AI Competition. Harvard Data Science Review, 1(2). https://doi.org/10.1162/99608f92.5a8a3a3d
Flew, Terry (2021) Regulating Platforms. Cambridge: Polity.
Just, Natascha & Latzer, Michael (2019) ‘Governance by algorithms: reality construction by algorithmic selection on the Internet’, Media, Culture & Society 39(2), pp. 238-258.
Lomborg, S., Kaun, A., & Scott Hansen, S. (2023). Automated decision-making: Toward a peoplecentred approach. Sociology Compass, 17(8). https://doi.org/10.1111/soc4.13097
Noble, Safiya U. (2018) A society, searching. In Algorithms of Oppression: How search engines reinforce racism. New York: New York University. pp. 15-63.
Sigfrids, A., Leikas, J., Salo-Pöntinen, H., & Koskimies, E. (2023). Human-centricity in AI governance: A systemic approach. Frontiers in Artificial Intelligence, 6, 976887–976887. https://doi.org/10.3389/frai.2023.976887
Be the first to comment