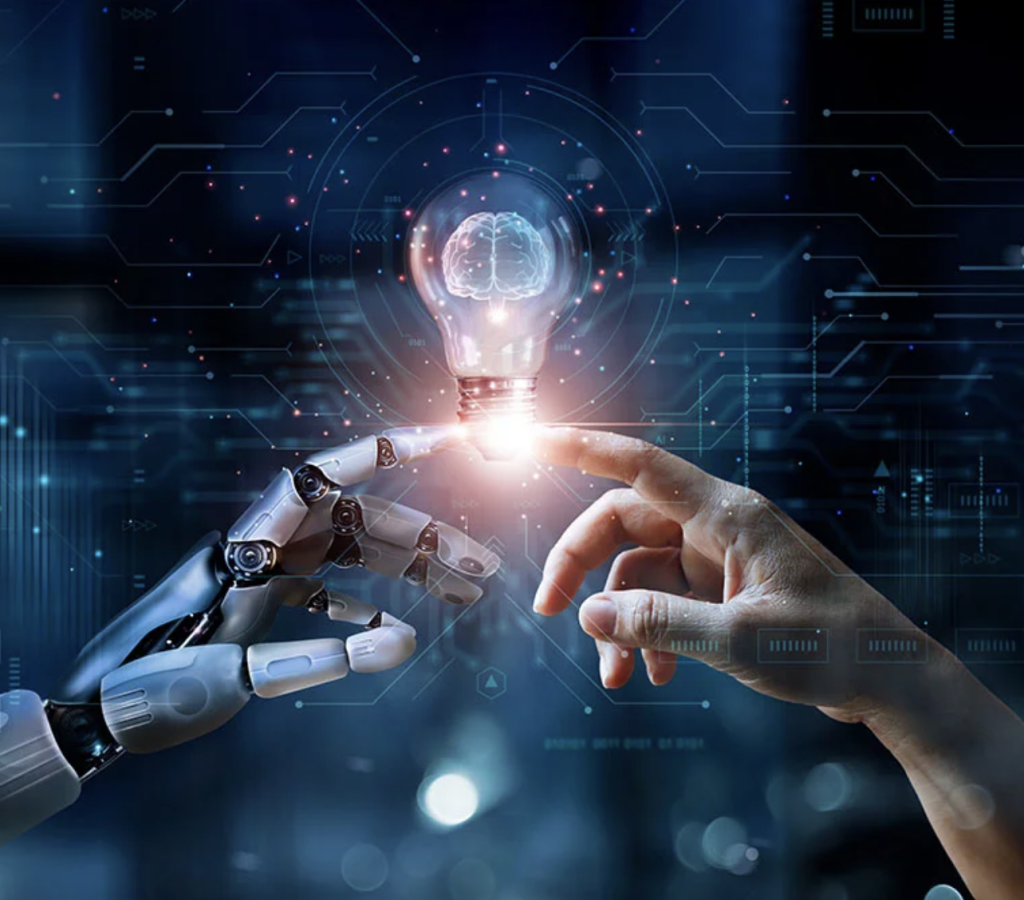
Algorithms Behind the Scenes: How is artificial intelligence quietly changing everything we do on social media? Have you ever had this experience? Post a comment online, only to have it inexplicably deleted or even have your account banned? At this point you may be wondering, “Who the hell decided I crossed the line?” Behind these seemingly magical things, the answer may not be a real person but in fact hides a ubiquitous but easily overlooked protagonist – artificial intelligence (AI).
From system recommendation, content review, and user behavior prediction, AI is undoubtedly behind social media and is increasingly becoming an important part of governing the digital society. Although the emergence of AI brings convenience to everyone, it also inspires a series of complex issues about bias, manipulation, and privacy invasion. In this article, we will explore how AI can participate in “governance” in social media, and what problems have been solved, or what new problems have been created? How do we respond to this invisible form of governance?
What is AI governance? What is the connection with you?
When we talk about “AI governance,” we might be thinking about government regulation, ethical codes, or technical regulations. However, the truth is that AI governance may also manifest itself in the moment we swipe our screens every day.
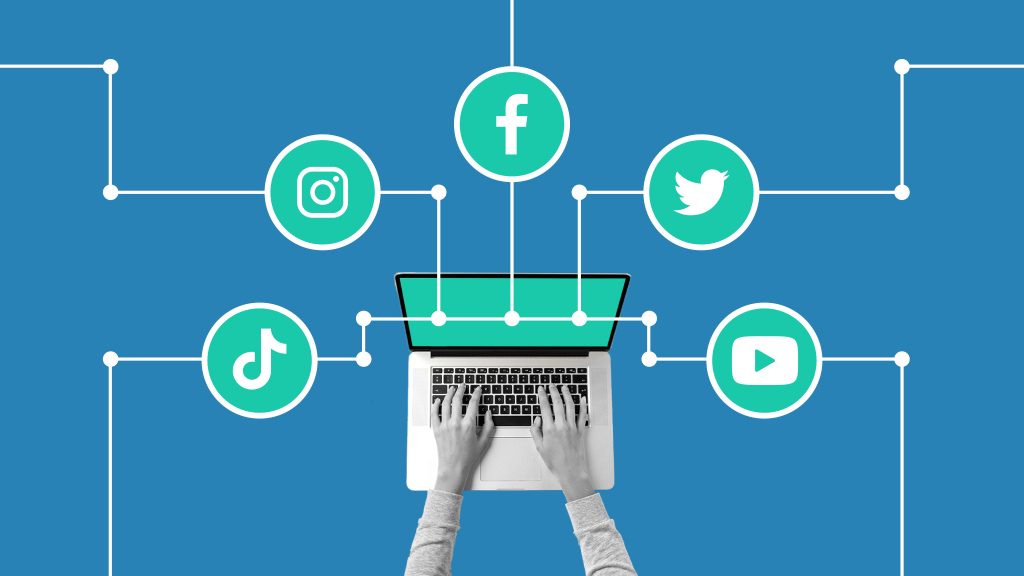
Flew (2021) pointed out that platform governance is no longer just policies and regulations but is quietly carried out through the “technology-governance fusion” such as algorithms and recommendation mechanisms. For example, TikTok’s “recommendation algorithm” is a kind of micro-governance, which determines what can be seen and what will be hidden, directly leading to the trend of public opinion, aesthetic orientation and even social cognition.
According to Crawford (2021), AI is not a neutral “neural network” but rather a “systematic arrangement” embedded with social power relations and technology choices. The governance function of AI exists in the field of huge data sets and training models, but these data are usually derived from users’ own activities on the platform. From this perspective, social media platforms are not just entertainment tools but also governance devices for modern society. In other words, when you think you’re free to watch a video, you’re in a virtual community that’s being “guided” by an algorithm, and you don’t even know it. This makes AI not just a neutral technical tool but an arm of platform power.
Recommendation Algorithms and the “Visible Hand”
Algorithm recommendation should be one of the most recognized AI intervention methods at present. It mainly relies on the target user’s browsing records, likes, interaction frequency and other data to create a “personalized information cocoon” for users. This may sound sweet, but it’s also potentially dangerous.
When algorithms only push what we “want to see”, we may fall into cognitive bias, self-repetition, and lose space for public discussion and multiple perspectives (Pasquale, 2015). This “content bubble” is not the result of our active choice, but the AI quietly “makes decisions” for us behind the scenes. The most typical example is the phenomenon of “information polarization” during the 2020 US presidential election. When AI push mechanisms on social media platforms facilitate the spread of fake news and the amplification and group polarization of conspiracy theories, they directly weaken the rational public space needed for democratic institutions (Livingston & Bennett, 2020).
All in all, the recommendation algorithm is sweet and good at “getting you”, but it’s even better at knowing how to manipulate your attention to affect your mood.
AI in Content Moderation: Fair Judgment or Lost touch with Humanity?
A bigger problem is that AI models themselves are often embedded with biases. Pasquale (2015) refers to this “black-boxing” of algorithms as the “black-box society”: we cannot know how the algorithm makes its decisions, nor can we challenge it. If you are an LGBTQ user, there are times when certain keywords will be triggered on the platform, but they will be “abnormally censored” and even cause the content to not be pushed. Could it be a coincidence? In fact, it is not, but the implicit bias formed by the algorithm in the training based on a particular corpus.
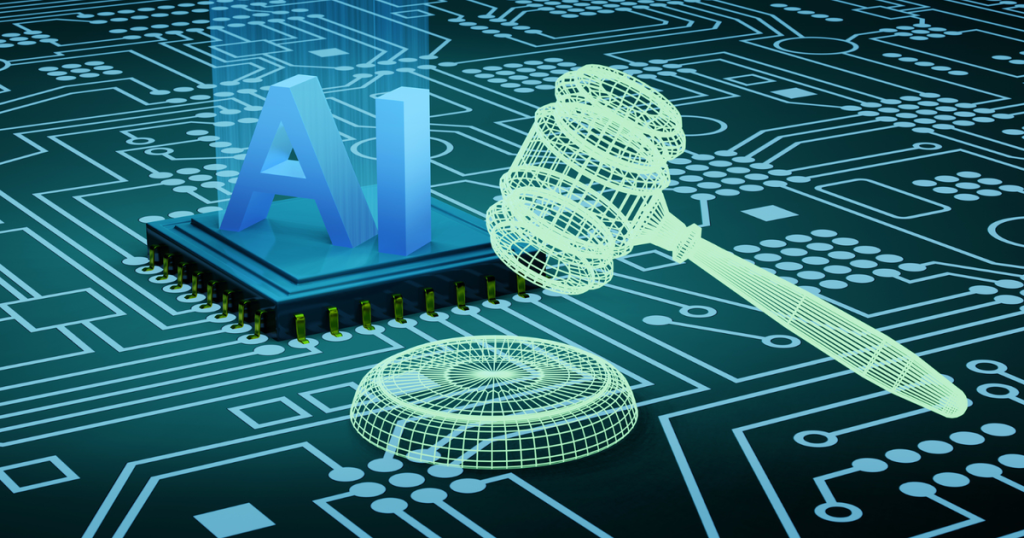
A more important scenario is content moderation. With the current proliferation of social media users, it is becoming more and more difficult for human moderators to process the huge amount of information, and more and more people are introducing AI automatic identification systems to detect hate speech, violent content or false information. That may sound like a good thing. But AI is not always accurate, and it is even easy to make mistakes, making what is right become wrong. Sinpeng et al. (2021) conducted an analysis of Facebook’s hate speech governance in the Asia-Pacific region and found that AI moderation systems often delete statements critical of the government and protest voices of minority groups as “illegal content” due to poor contextual understanding. Noble (2018) goes further and criticizes that many algorithms are inherently biased by race and gender. For example, if you type “Black girls” into a search engine, the AI will give you negative content; But typing “White girls” is a positive image. Is this by chance? Not then, but rather a manifestation of algorithmic bias.
More troubling, however, is that the companies behind the AI models, such as Google, never disclose the data they use, the vetting rules of their platforms, or even the “error rate” of their AI. Flew (2021) noted that the lack of transparency and accountability makes it difficult for platforms to be truly regulated. At the same time, Roberts (2019) pointed out that although “AI moderation” has become a popular word of mouth, there are still many human content reviewers behind it. They face a lot of shocking content every day, and some even suffer from PTSD. But these people are in the “system shadow” and are not seen by the platform or users.
All in all, AI audit is not a disconnect from human nature, because it is not entirely the decision of intelligent machines themselves, but the mode of collaboration between people and AI. It’s just that people often overlook the suffering reviewers behind the scenes. So, when we give censor power to AI, are we masking a deeper injustice with “neutral” technology?
The cost of privacy: Are we the “raw material of algorithms”?
To make AI “smart,” platforms need massive amounts of data. This data comes from what we do every day — likes, views, comments, even time spent. But do we really know what we’re “handing over”?
AI requires large amounts of data to train and optimize models, which leads to potential risks to user privacy. For example, Facebook’s advertising algorithms analyze users’ browsing habits to target ads, which has also led to privacy controversies (Flew, 2021). For example, the Cambridge Analytica data scandal in 2018, Cambridge Analytica illegally obtained the data of millions of Facebook users and used it for political advertising. This incident prompted the European Union to adopt the General Data Protection Regulation (Flew, 2021) to strengthen the protection of user data. Through user agreement, default Settings and other ways, the platform quietly deprived users of the right to know and control the data. This “invisible transaction” of data in exchange for services makes us unconsciously the “raw material of algorithms”.
In contrast, Europe’s GDPR (General Data Protection Regulation) seeks to restore users’ right to know and choose, including mechanisms such as “right to be forgotten” and “data portability.” However, Australia’s current privacy laws are relatively backward and lack a strong enforcement mechanism (Flew, 2021). In other words, most of the time, we are being accurately modeled by AI without knowledge or choice.
How can we counter it? Explore the possibility of artificial intelligence with human-oriented and more humanized design
The application of AI on social platforms is almost ubiquitous, from content recommendation to information review. AI has indeed achieved remarkable results in terms of governance efficiency. It can quickly identify and filter disinformation as well as hate speech and malicious content, creating a safer environment for the public opinion space. But this “efficiency” also brings new worries and challenges.
AI coexists with potential risks in terms of governance efficiency. The AI’s judgment is not neutral because it is run by the rules extracted from the big data and the training model. This means that if there are biases in the data itself, AI decisions are likely to perpetuate or even amplify those biases. For example, when processing sensitive content, AI may mistakenly block the voices of marginalized groups, deepening the unequal spread of information; Even when content reviewers work with AI, they are often exposed to extreme content, and their physical and mental health are seriously threatened.
The question we need to ask ourselves may not be “should AI audit us?” but “how do we participate in AI audit design?” In the era of information flow dominated by algorithms, the key lies in how to regain the right to understand and participate in technology. The concept of “human-centric AI” has quietly emerged, which advocates putting human values at the core of technological development and promoting the evolution of AI to a more fair, transparent and interpretable direction. For example, the European Union is actively promoting the policy of “explainable AI”, which requires the system to not only provide results, but also explain the basis and process of decision-making, to improve the understanding and trust of users (Pasquale, 2015). We are not powerless in the face of increasingly sophisticated algorithmic control in social media. There are four main ways in which feasibility can be improved:
- Algorithmic transparency:Platforms should disclose the basic logic and key variables of their recommendation mechanisms, allowing users to understand “how the content was selected to show me”.
- Interpretable design: The user should know clearly, “Why am I seeing this?” rather than being led silently by the algorithm.
- Algorithmic choice: The platform provides a variety of ways to sort information, such as allowing users to switch to timeline sorting instead of always default “recommended sorting”.
- Data sovereignty restoration: Legislation to strengthen users’ control over their personal data, including the right to access, modify and delete data.
These measures are not only technical design adjustments, but also a positive response to “algorithmic citizenship”. We should change from passive “users” of algorithms to active “participants” of algorithms and use our collective voice to influence platform policies and technical specifications.
Countering does not mean being the enemy of technology, but it means that we can still insist on human values, ask questions, and participate in decision-making in the face of powerful technological systems. A fairer, more transparent, and more dignified digital world depends not on the computational power of AI, but on our willingness to engage, set boundaries, and guide direction. Human-oriented AI is possible if we stop taking “algorithms” for granted and start thinking of them as social infrastructure that can be co-designed and co-governed.
Conclusions
When AI becomes the governing agent, how will we live? AI is no longer the technology of the future; it has become a structural force in our daily lives. Through algorithmic recommendation, content review, systematic ranking, and future prediction, it has quietly changed the way citizens access information, express opinions, and understand the world. So, what we need to do is not reject AI, but understand its logic and identify its biases, and participate in its governance. This is not only a question of technology ethics, but also a basic proposition of democratic society. So, the next time you see a video that you just want to see, think for a second: Is this my choice, or is it an AI feed?
References:
Bruns, A., Harrington, S. & Hurcombe, E. (2021) ‘‘Corona? 5G? or both?’: the dynamics of COVID-19/5G conspiracy theories on Facebook’, Medi International Australia 177(1), pp. 12-29.
Crawford, Kate (2021). The Atlas of AI: Power, Politics, and the Planetary Costs of Artificial Intelligence. New Haven, CT: Yale University Press, pp. 1-21.
Flew, Terry (2021). Disinformation and Fake News. In Regulating Platforms. Cambridge: Polity, pp. 86-91
Livingston, S. & Bennett, W. L. (2020) A Brief History of the Disinformation Age: Information Wars and the Decline of Institutional Authority. In S. Livingston & W. L Bennett (eds.) The Disinformation Age: Politics, Technology, and Disruptive Communication in the United States. Cambridge: Cambridge University Press, pp. 3-40.
Pasquale, Frank (2015). ‘The Need to Know’, in The Black Box Society: the secret algorithms that control money and information. Cambridge: Harvard University Press, pp.1-18.
Roberts, Sarah T. (2019). Behind the Screen: Content Moderation in the Shadows of Social Media. New Haven, CT: Yale University Press, pp. 33-72.
Sinpeng, A., Martin, F., Gelber, K., & Shields, K. (2021, July 5). Facebook: Regulating hate speech in the Asia Pacific. Final Report to Facebook under the auspices of its Content Policy Research on Social Media Platforms Award. Dept of Media and Communication, University of Sydney and School of Political Science and International Studies, University of Queensland. https://r2pasiapacific.org/files/7099/2021_Facebook_hate_speech_Asia_report.pdfLinks to an external site.
Matamoros-Fernández, A. (2017). Platformed racism: the mediation and circulation of an Australian race-based controversy on Twitter, Facebook and YouTube. Information, Communication & Society, 20(6), 930–946. https://doi.org/10.1080/1369118X.2017.1293130
Be the first to comment