While the modern world is moving towards an automated understanding of almost everything, the important question that is nagging almost every person using AI is, “Is AI neutral?” In a study from MIT and Stanford University, it was found that software using AI for facial recognition shows an error rate of 0.8 percent for light-skinned men, while the error rate skyrocketed to 35.7 percent for women who have dark skin. The experiment depicts not just the growing concerns about the biases in the model software, but also shows how using AI in daily life can be harmful to a certain group, in this case, the dark-skinned females.
The idea that AI leads to the creation and maintenance of a biased approach about humans and events is not unknown. There are numerous examples throughout the internet which depict how using AI can lead to bias in the model in terms of hiring, policing of minorities, and so on. These examples not only show the inherent flaw of AI-biased models but also raise the concern of whether these flaws can be fixed and is AI neutral? As such, this blog claims that even though AI is considered objective, it reflects and increases the biases held by human beings. Moreover, the blog asserts that these biases held by AI are not just a flaw or bug that can be fixed, but rather, they are a part of the system. It uses topics such as policing, hiring, and facial recognition to justify this position.
AI in Policing: A way of understanding crime or increasing systematic discrimination?
The idea of using AI in policing by governmental agencies is widespread, and numerous agencies have used AI for monitoring. The GAO (2023) report shows that there are nearly 1,200 current and planned use cases of AI in the federal system.
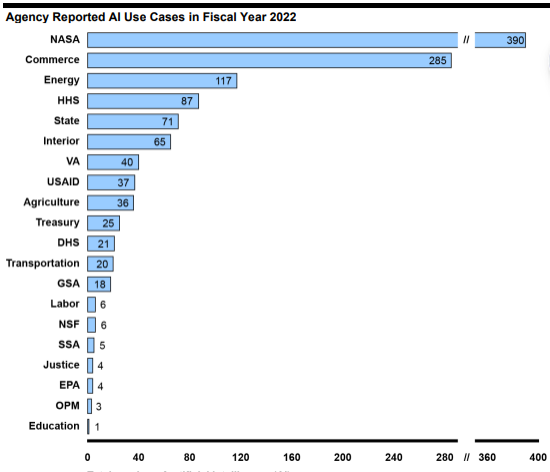
Total number of AI use cases (GAO, 2023)
These efforts by the agencies highlight that the use of AI as a means of federal forecasts can help understand crime hotspots, police the routes where crime is likely to take place, and understand the risks associated.
The main idea behind this understanding is that AI can help process huge chunks of information and make police work easier and more effective. However, AI models use existing data to train themselves and base their predictions and analyses. The use of this form of analysis highlights the concern that existing data can be flawed and affect the policing efforts undertaken by the agencies.
According to Couldry and Meijer (2018), data used by AI is extracted through numerous sources, and the users are more often than not, unaware of this extraction. This is very commonly used in policing based on facial recognition. The researchers define this method as data colonialism and state that it is collected without the conscious input of the individual. This highlights that the digital landscape creates an atmosphere for biases in the model to penetrate and creates an unsafe environment for certain individuals, for example, individuals from different races than the dominant one in a region.
This likelihood of a biased understanding of race was expressed by ProPublica (2016). The forum brought forward a case where Brisha Borden, a black teenager, was arrested for attempting to steal a bicycle in 2013. On the other hand, the White offender, Vernon Prater, who was arrested at a similar time, had previous theft convictions during his arrest. The AI system COMPAS evaluated the two subjects using its assessment capabilities. It labelled Borden as high-risk, although she did not have any history of arrests or convictions, while it indicated low risk for Prater, although he had previous criminal convictions.In the following two years, Borden displayed no criminal activity, while Prater received another arrest for theft. ProPublica conducted an investigation which determined that the COMPAS algorithm classified defendants twice as frequently to commit a crime when they were Black subjects, thereby increasing racial prejudice within historical arrest records.
The problem? Artificial Intelligence systems do not eliminate bias in the model but rather make it a self-perpetuating function.
The investigation by ProPublica also highlights another problem that was discussed earlier: AI systems are based on past data. This means that if the past data is biased, the predictions made by AI and the policing efforts it undertakes will also depict the bias held in the past. This is a major concern, as if certain community members were arrested in past for a specific crime, the AI will assume and predict that those communities are inherently criminal and they are more likely to commit crimes irrespective of the situational factors. Moreover, the case by ProPublica also highlights that even if the algorithm is tweaked, the biases held by AI software will persist as they are based on an understanding of already skewed data.
AI in hiring: A means of Hiring or Automating Discrimination?
The biased thinking model of AI is not just limited to policing efforts; rather, it is also prevalent in the hiring of candidates for jobs. Nearly 82% of companies use AI to analyse resumes, while 40% use AI chatbots to talk with potential candidates. Additionally, 23% of such companies use AI technology for interviewing the candidates, and 64% use the AI models to review the assessments done by candidates. And guess what? These aren’t even current statistics! So, this means that these statistics are only supposed to increase in 2025.
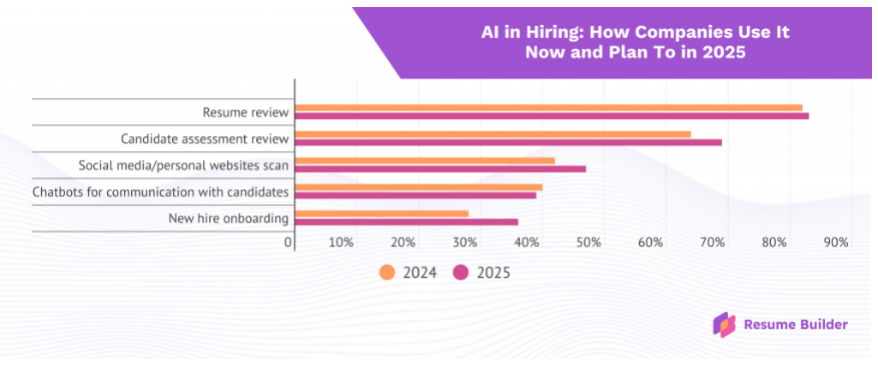
AI planned and current use in hiring (Resume Builder, 2024)
The utilisation of AI in the hiring process is aimed at making the process smoother; however, it highlights the concern of biased understanding and historical data. This was demonstrated in the 2018 Amazon fiasco.
Amazon used an AI system to enhance recruiting processes until this tool started discriminating against female candidates, depicting gender prejudice. The system received training through historical hiring data that revealed male candidates received better treatment, which caused it to lower the scores of applications that had female-centric phrases. The bias exhibited in the hiring tool continued to manifest itself even after performers made attempts to correct it.
Amazon discontinued its AI recruitment tool in 2018, however, it highlighted the main flaw of AI: It learns from human decisions, irrespective of them being flawed.
The example of Amazon shows AI continues to detect underlying patterns from training data even when companies attempt to remove biased words.Thus, AI systems reflect previous discriminatory hiring practices through automated systems, which generate harder to identify forms of discrimination.
The Case of NYC Bias Audit Law
In 2023, New York City implemented a historical law that forced businesses that use Automated Employment Decision Tools (AEDTs) to screen their AI tools for biases before using them. The regulatory standard was built to establish fair hiring practices through automated systems, which must not discriminate against individuals based on their race or gender.The introduction of this law exposed the fundamental fact that bias exists deeply within the core structures responsible for AI decision-making. Many AI tools kept generating biased results in post-audit assessments because they processed hiring data which historically favored specific groups of employees.
The lack of viable methods to achieve compliance made several companies abandon their AI tools completely. The circumstances show that AI regulation measures can succeed, however, biases persist since the corrupted learning basis connects directly to the source data.
In the modern landscape, numerous agencies and companies use AI for facial recognition. Some of the common AI tools used for facial recognition include Amazon Rekognition, Google Cloud Vision API, and Microsoft Azure Face API.
The basic premise for using AI tools for facial recognition is that they will be highly accurate and would recognise individuals irrespective of race and gender. However, the reality as depicted by Larry Hardesty from the MIT News Office is very different. In the study, the researchers proved that AI software’s facial recognition is less accurate for dark-skinned females when compared with white-skinned males.However, the basis of the inaccuracy of AI facial recognition software is not just limited to the study conducted by MIT and Stanford. The case of the wrongful arrest of Williams in 2020 depicts how AI-based facial recognition software reinforces gendered biases.
The AI-based facial recognition system malfunctioned when it pointed to Robert Williams, a Black resident of Detroit, as a shoplifting offender, leading to his wrongful arrest in 2020. Facial recognition technology enabled police to match Williams in surveillance images, although the images were indistinct and there was no supporting evidence. Law enforcement authorities kept him detained for 30 successive hours until his release.Public concerns about facial recognition technology accuracy have escalated because its system tends to mistake people based on their race. Despite their likelihood of creating racial errors in identifying people, the devices keep getting deployed by authorities even though their source data sets are frequently found to contain biases.
Civil rights groups now use Williams’s case to call for total restrictions or harsher surveillance of facial recognition in law enforcement because they believe these systems strengthen racial discrimination through computer technology.While Williams was only detained for 30 hours, the case depicts the growing concerns of using software such as this in law enforcement. Moreover, this software is deeply flawed and can perpetuate racial profiling of individuals.
The case also shows that AI software learns from test studies that underrepresent people of colour, and the chances of fixing this problem are minute, as these are historical records.
Why is Finding a Quick Fix for the Malfunction in AI Nearly Impossible?
The examples of AI-based software in facial recognition, hiring, and policing show one thing! Biases in the model are not simply a glitch in the tool! Rather, they are a structural flaw, and there is not much that can be done to solve it!
As AI software learn from trainer data and is based on structural inequalities that have penetrated society for eons, they are a depiction of society’s inequality, and the past cannot be rewritten. So, even if the bugs are attempted to be fixed, as seen in Amazon’s hiring AI software example, there is not much that can be done to create a proper representation.
So now the main question is, if AI is so biased, what can be done to solve this issue?
So, What Can be Done to Provide a Solution?
- Fairness audits: To ensure that the data gathered from AI is neutral, the governmental agencies and companies using such software should undertake fairness audits on a regular basis so that it can help not only question the data but also the biases held by people.
- Inclusion of diverse datasets: It is a recognised fact until now that AI are based on test data from the historical incidents. While these historical incidents cannot be rewritten or removed from the internet, it is a good practice to ensure that diverse datasets are fed to AI. This can help limit the bias rather than eliminate it.
- Recognising and accepting the flaws: Rather than believing that AI data can be made fair, human beings should realise that these data reflect the biases held by society, and if the past is any indicator, no form of software adaptation can remove these biases. Hence, it is recommended that AI’s flaws should be accepted and it should not be used in high-stakes decisions.
Conclusion
It can be concluded that AI software is deeply and structurally flawed as it uses human history and data to create predictions and analyses. Implementing such software in policing, hiring, and facial recognition leads to more harm to society and individuals, especially minorities. Moreover, as this software uses past data from society and test studies, there is no way in which these gaps can be fixed. Thus, the biases held by AI are not just a flaw or bug that can be fixed, but rather, they are a part of the system, and society must realise this so that their utilisation can be limited.
References
Allyn, B. (2020, June 24). ‘The Computer Got It Wrong’: How Facial Recognition Led To False Arrest Of Black Man. NPR. https://www.npr.org/2020/06/24/882683463/the-computer-got-it-wrong-how-facial-recognition-led-to-a-false-arrest-in-michig
Angwin, J., Larson, J., Mattu, S. & Kirchner, L. (2016, May 23). Machine Bias. ProPublica. https://www.propublica.org/article/machine-bias-risk-assessments-in-criminal-sentencing
Candelon, F., Carlo, R., Bondt, M., & Evgeniou, T. (2021, September-October). AI Regulation Is Coming. Harvard Business School. https://hbr.org/2021/09/ai-regulation-is-coming
Couldry, N., & Mejias, U. A. (2019). Data colonialism: Rethinking big data’s relation to the contemporary subject. Television & New Media, 20(4), 336-349. https://doi.org/10.4000/questionsdecommunication.29845
Crawford, K. (2021). The atlas of AI: Power, politics, and the planetary costs of artificial intelligence. Yale University Press.
Datson, J. (2018, October 11). Insight – Amazon scraps secret AI recruiting tool that showed bias against women. Reuters. https://www.reuters.com/article/world/insight-amazon-scraps-secret-ai-recruiting-tool-that-showed-bias-against-women-idUSKCN1MK0AG/
Gao. (2023). Artificial Intelligence. Agencies Have Begun Implementation but Need to Complete Key Requirements. https://www.gao.gov/assets/gao-24-105980.pdf
Hardesty, L. (2018, February 11). Study finds gender and skin-type bias in commercial artificial-intelligence systems. MIT News Office. https://news.mit.edu/2018/study-finds-gender-skin-type-bias-artificial-intelligence-systems-0212
Maurer, R. (2024, February 18). New York City AI Law Is a Bust. SHRM. https://www.shrm.org/in/topics-tools/news/technology/new-york-city-ai-law
Resume Builder. (2024, October 22). 7 in 10 Companies Will Use AI in the Hiring Process in 2025, Despite Most Saying It’s Biased. https://www.resumebuilder.com/7-in-10-companies-will-use-ai-in-the-hiring-process-in-2025-despite-most-saying-its-biased/#:~:text=Today%2C%2082%25%20of%20companies%20use,AI%20to%20review%20candidate%20assessments.
Be the first to comment