On the night of April 3, in a sea of cheering footy fans at Melbourne’s iconic MCG, two men slipped through security with guns—right under the nose of an AI scanning system (Doran, 2025). After reading this report on ABC News, it’s hard not to wonder: are these so-called advanced AI security technologies really reliable?
Before diving into the main discussion, let’s take a moment to understand the security dilemma faced by the MCG. As Australia’s largest stadium, the MCG makes the challenges of event security even more visible. On one hand, sports stadiums are considered potential terrorist targets; on the other, fans are often frustrated by the inefficiency of manual screening.
Imagine this: you’re a die-hard fan, and today is the big final. You arrive ten minutes before the game starts—only to see a massive queue stretching out ahead. Every fan has to place their bag on a conveyor belt, hand over their phone and keys, and go through a full-body scan by staff. So, even though you’re standing just outside the stadium, you end up watching the first pitch on your phone in a livestream.
With the introduction of Evolv Technology’s AI screening system, fans now have a much better chance of getting to their seats in time. But the problems haven’t disappeared entirely. Reports of missed detections have triggered a crisis of trust in the technology—and exposed deeper flaws that today’s governance systems are struggling to address. AI algorithms are not neutral tools; they are data-driven, unauditable, and operate as black boxes with blurry lines of accountability. This blog post explores the black-box nature of AI in security settings, and the broader governance challenges brought about by automation.
Who’s really to blame—AI or humans?
Evolv Technology is a company headquartered in Massachusetts, USA. Its security products are typically installed in public places such as schools and stadiums and are capable of detecting both metallic and non-metallic threats. The company has also developed facial recognition technology to identify and handle suspicious individuals in various situations.
“Today’s visitors expect a touchless experience, but for decades, the industry has been developing technologies that meet outdated detection standards.” said Mike Ellenbogen, the founder of Evolv Technology. He stated that their team introduced artificial intelligence. AI processes data from many sensors on the physical system to help distinguish between potential threats and many common personal items, which ensures high detection accuracy while eliminating the need for physical contact required by traditional metal detectors (Evolv Technology, n.d.).
Evolv Technology is widely used across the United States, including at the Super Bowl and Taylor Swift concerts. It has even been recognized by the U.S. Department of Homeland Security (DHS) as an advancement in anti-terrorism technology. However, in recent years, doubts and accusations about Evolv Technology have not been limited to the incident in Melbourne.
In October last year, a student at the Utica City School District in New York secretly brought a hunting knife into a high school and attacked another student, stabbing him multiple times. The district had invested 3.7 million dollars in the system, yet it failed to detect guns and multiple knives.
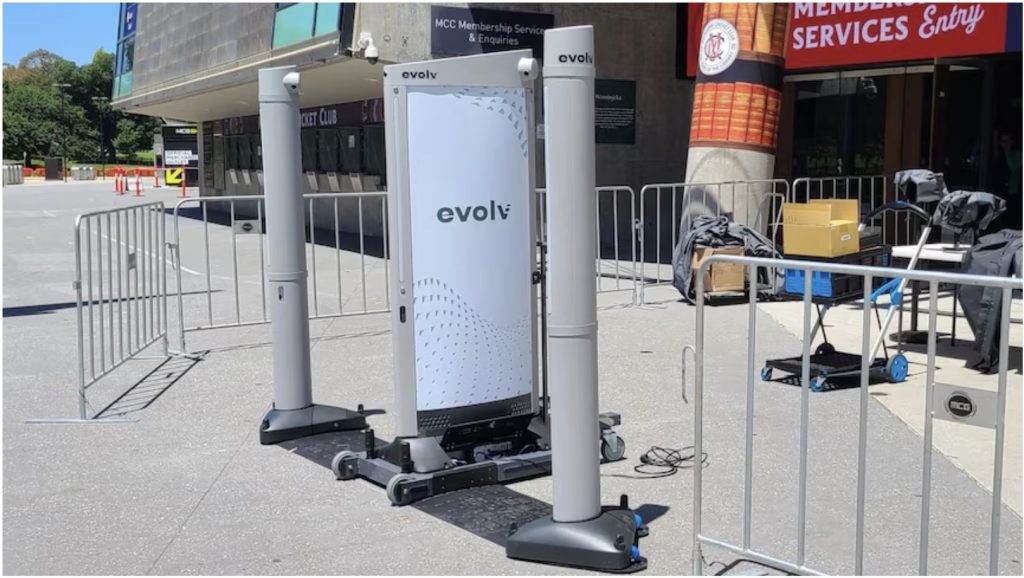
After that, Evolv Technology commissioned a study by the National Center for Spectator Sports Safety and Security, which found that the system failed to detect 42% of knives and a small number of guns. Evolv is continuously working to improve its system, but it can never achieve 100% accuracy. In addition to the system itself, extra security measures are needed to ensure public safety.
In reports about the armed individuals found at the MCG, Evolv explained that it was not a failure of the system itself, but rather a lapse during the secondary manual screening. The high false alarm rate of the AI system leads to operator fatigue. A security system that was intended to increase efficiency has, in fact, not reduced the burden on people.
Technology is not omnipotent. In The Atlas of AI: Power, Politics, and the Planetary Costs of Artificial Intelligence, Crawford (2021) puts forward the idea that AI is neither artificial nor intelligent—it has no consciousness of its own.
To put it simply, a human child, even if abandoned by their parents, will instinctively learn from their environment and develop diverse values. In contrast, AI cannot recognize anything at all unless humans invest in it and train it using vast amounts of natural resources, fuel, and human labor.
If we trace the issue back to its root, the reason AI receives such immense support is because it aligns with existing dominant interests. Since AI capabilities have rapidly advanced and more generative AIs like ChatGPT have entered daily life, the world has been swept by an AI frenzy. From the perspective of businesses, AI can help reduce labor costs and boost productivity. From the perspective of governments and states, AI development is tied to a range of economic and technological indicators.
AI cannot decide for itself which direction to develop in; instead, it evolves alongside social practices, institutions and infrastructures, politics, and culture. Therefore, AI is not merely a technical matter—it is, at its core, a reflection of human power systems.
Public trust in AI security systems largely stems from the mythologization of technology. Built on the systematic extraction of Earth’s resources, human labor, and vast amounts of user data, AI often seems to “know” what humans don’t. For those who don’t understand the technical principles behind it, AI becomes an object of excessive trust—almost a modern-day god. However, the data used to train AI often comes from people who have no technical awareness at all. AI is not god. It is made, fed, and used by ordinary humans—and the problems of human society will always be more complex than any existing technology can solve.
When incidents like the one at the beginning of this blog happen, we can’t simply blame the AI itself. The responsibility lies with the forces behind the technology, the institutions and actors who control its development, deployment, and oversight.
The AI security system is a “black box.”
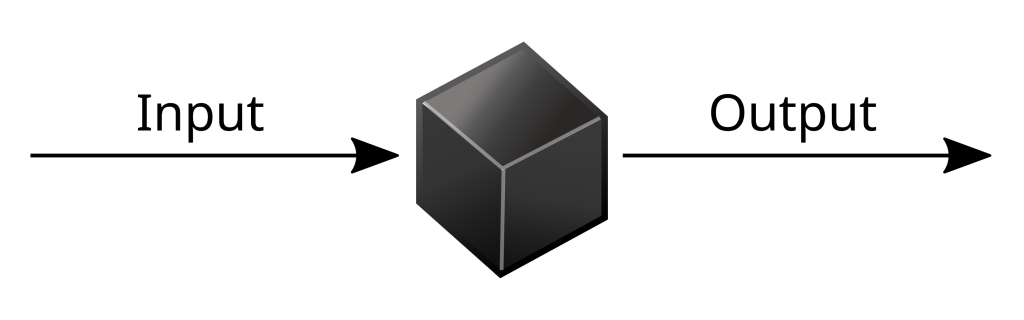
The term “Black Box” originally referred to flight data recorders—after an aviation accident, people would rely on the black box to find out the real cause of the malfunction. Later, the concept was abstracted and applied to various fields. A black box system is one where we can observe its inputs and outputs, but have no idea how it operates internally.
With the rise of internet and financial companies, massive amounts of user data have been collected and used for internal decision-making. This is a classic example of a black box. As ordinary users, we provide data to companies, they analyze it and make decisions that ultimately influence the decisions we make for ourselves. And yet, we don’t know how our data is actually being used (Pasquale, 2015).
Evolv Technology is also a kind of black box. Schools use AI security systems to detect knives. Students walk through the machines, and the system displays a result—but no one really knows how the detection process works. Although this system is frequently used in public settings, it is fundamentally a product of a private company. The specifics of how the system operates are tied to business interests and profit, and there is not enough transparency.
However, from the perspective of society and national safety, it is crucial to understand the limits of what such a system can do. As the security and technology group IPVM has pointed out, the company needs to clearly state what the system can detect—and what it can’t.
As ordinary people, what we need to pay attention to is not only whether the system is effective, but also how it affects social relationships and personal interactions. As Andrejevic (2019) points out, automated systems are not just tools—they are part of the social order, and the way they operate is often opaque to users. While automation can reduce human bias in many cases, the systems themselves often inherit the biases embedded in the databases they were trained on.
At present, there are no complete complaint channels or accountability mechanisms specifically for AI. So when a security system fails to detect a knife or a gun and someone gets hurt, it becomes unclear who is responsible. Evolv claimed that it was entirely the fault of the secondary screening process for failing to detect the knife, while the venue argued that Evolv should be held accountable for the scanner’s false positives.
As automated systems continue to develop rapidly, there is always a risk that we could be harmed by their flaws—and have no way to appeal due to their black-box nature. This is exactly why algorithmic governance matters more than ever.
Algorithms need to be governed—but who gets to do it?
Before we discuss how to govern AI, we should first consider what governance actually means. Just and Latzer (2017) suggest that governance can be broadly understood as a form of institutional steering.
Imagine a traditional, government-centered model. Now picture a diagram with an x-axis and y-axis extending outward from that core—that’s where the complexity of algorithmic governance begins: it involves a wider network of social actors and relationships.
Let’s look at the x-axis first. Horizontally, effective governance requires the involvement of both public actors like governments and private actors like the companies that develop and implement AI. Then on the y-axis, the idea of multilevel governance comes into play—this includes global aspects as well as technical and infrastructural governance strategies.
From the x-axis perspective, we can see that AI governance is currently driven mainly by private companies, but governments are becoming increasingly involved. From the y-axis perspective, effective regulation must begin with a clear classification of AI products available on the market. Without recognizing the different categories and levels of risk, treating all AI systems the same will only lead to superficial or performative governance.
Many scholars have conducted research on the classification of AI programs. For example, Just and Latzer (2017) classify existing algorithm-based applications in their article.
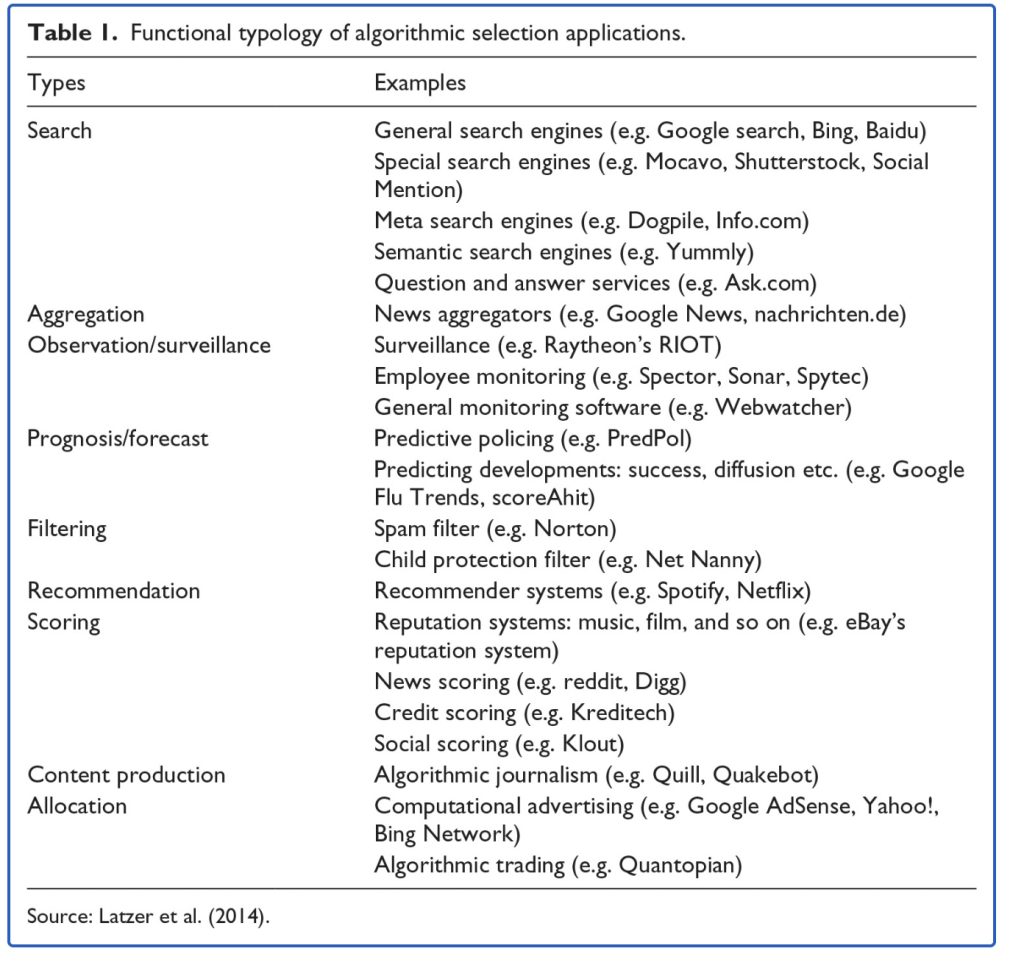
At the practical level of AI governance, one of the most representative examples is the European Union Artificial Intelligence Act, proposed in April 2021. This legislation establishes a risk-based classification system for AI, with different rules for different risk levels. According to an article published by the European Parliament (2023), the risk levels are divided into two main categories: Unacceptable risk and High risk.
The unacceptable risk category typically involves threats to public security or violations of human rights, and such systems are completely banned. Examples include toys that manipulate children’s behavior, AI systems that rank individuals based on personal characteristics, and biometric identification systems used in public spaces. The high risk category includes systems that are not banned but may pose significant risks to safety or fundamental rights.
AI products categorized as high risk must be registered and go through formal assessment procedures. An AI system like Evolv’s, used for public security screening, would fall under the high risk category in this framework. If Evolv were a European company, it would be required to undergo rigorous audits and regulation.
However, such regulations vary significantly across countries, and their actual effectiveness will take time to evaluate. Still, one thing we can be certain of from these studies is this: If we want AI to truly serve the public, governance needs to be transparent, inclusive, and enforceable. Government intervention is essential—we cannot rely solely on corporate self-regulation.
Words in the end
This blog post began with a failure in an AI-powered security system, and gradually shifted toward the much broader and more complex issue of AI governance. Some might wonder:
If governing AI is this difficult and full of hidden risks, why don’t we just choose not to use it at all?
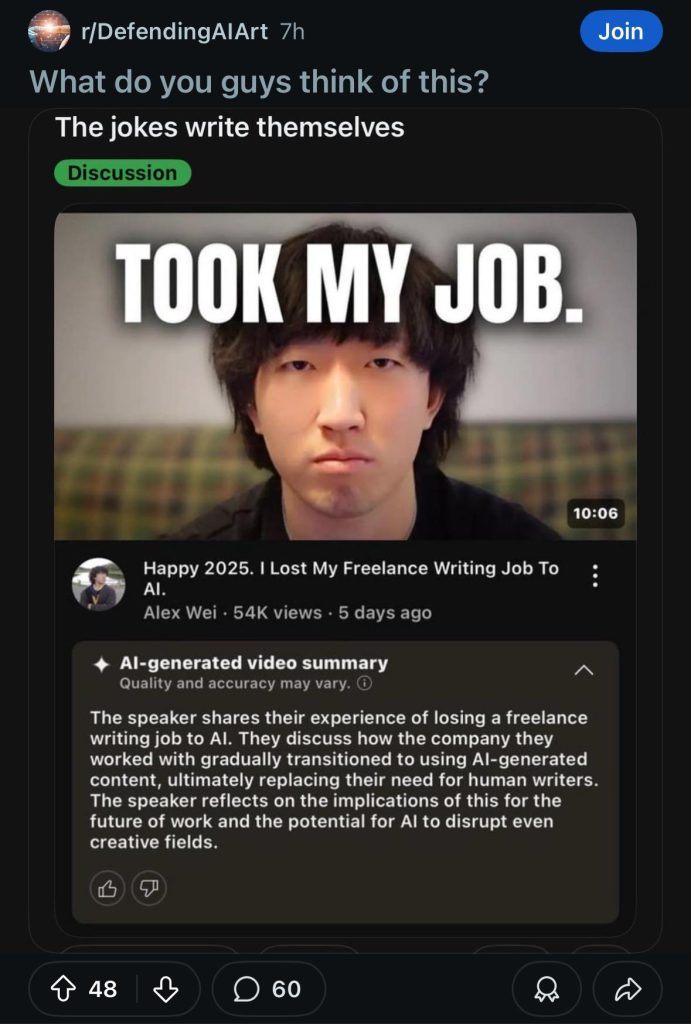
In the school example mentioned earlier, even though an incident occurred where a student was injured due to the system failing to detect a knife, the decision was still made to continue using Evolv Technology’s product. The reason is that this method still saves a significant amount of time and human labor. People simply need to walk through the machine, rather than remove all their clothing and be checked piece by piece.
Examples of how technological advancement disrupts existing systems can be found throughout history. When photography was invented, it greatly impacted the field of realistic painting. When wristwatches were introduced, people even felt a sense of anxiety, as if their time was being controlled.
The advancement of technology always comes with new problems. But once we learn to navigate them, we begin to realize just how profoundly these new tools can transform our lives.
Reference
Andrejevic, M. (2019). Automated media. Routledge.
Crawford, K. (2021). The atlas of AI: Power, politics, and the planetary costs of artificial intelligence. Yale University Press.
Doran, M. (2025, April 5). Evolv AI scanner concerns raised after guns carried into MCG during AFL match. ABC News. https://www.abc.net.au/news/2025-04-05/evolv-concerns-mcg-guns-security-breach/105138072
European Parliament. (2023, June 1). EU AI Act: First regulation on artificial intelligence. European Parliament. https://www.europarl.europa.eu/topics/en/article/20230601STO93804/eu-ai-act-first-regulation-on-artificial-intelligence
Evolv Technology. (n.d.). Evolv Express® – Artificial intelligence-based weapons detection. https://evolv.com/
Just, N., & Latzer, M. (2017). Governance by algorithms: Reality construction by algorithmic selection on the Internet. Media, Culture & Society, 39(2), 238–258. https://doi.org/10.1177/0163443716643157
Pasquale, F. (2015). Introduction: The need to know. In The black box society: The secret algorithms that control money and information (pp. 1–18). Harvard University Press. http://www.jstor.org/stable/j.ctt13x0hch.3
Be the first to comment