Asian students post daily vlogs on TikTok, while foreign users send various squint-eyed emoji combinations in the comments section with the caption “Your eyes are so cute.” The platform system judged it as a “neutral rating.” Still, this emoji combination is often used in Western contexts as an appearance discrimination against Asian people (mimicking the squinting eye stereotype) (Kim, 2017).
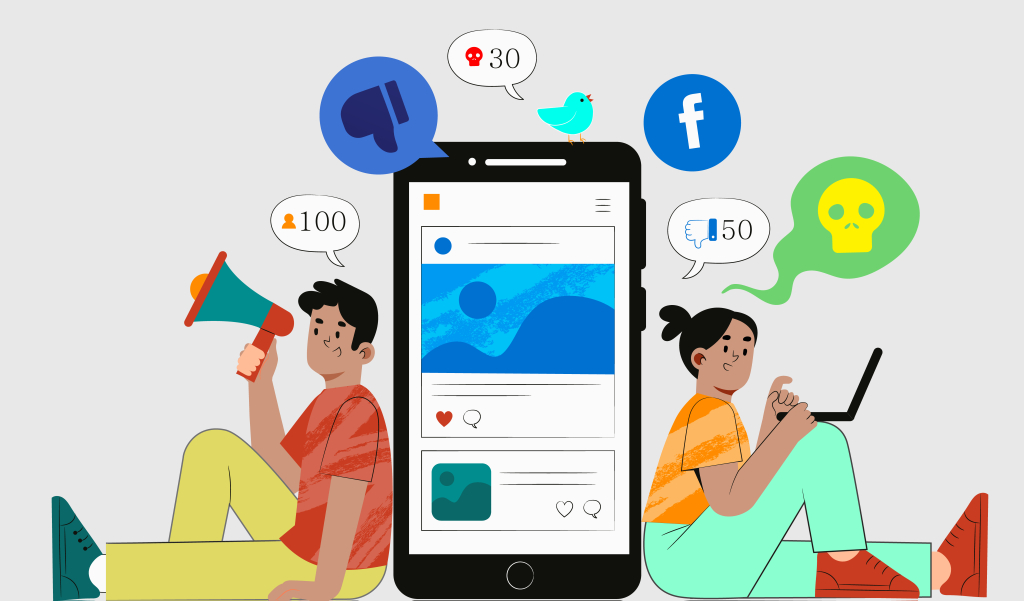
These sentences and characters are like an invisible knife cutting people’s living spaces. This is not alone—a two-decade-long experiment in “digital translation” is exposing a profound crisis of governance as algorithms in Silicon Valley try to understand complex cultural contexts in regions such as the Asia-Pacific (Gelber, 2019).
- Cultural metaphors cannot be captured by algorithmic technology
There are more than 2,300 languages in the Asia-Pacific region, but Facebook’s machine-learning classifier covers only 40 in 2019 (p.6). It cannot identify sub-national dialects, lingua franca and culture-specific terms. Due to the diversity of languages and insufficient localization of algorithms, it misses a serious judgment in the identification of dialects and culture-specific discrimination in the Asia-Pacific region, and cross-discrimination involving religion, caste, and region often becomes a regulatory blind spot (Sinpeng, 2021).
Meanwhile, how does the untransliterability of the symbol system exacerbate the prevalence of hate speech? According to a comparative experiment between English and Bengali, in low-resource languages such as Bengali, the use of emoji is deeply bound to cultural context (such as specific religious or regional symbols), while the pre-training data of models such as Multilingual BERT are mainly English.
Resulting in poor semantic understanding of non-English symbols. This reveals the core mechanism of platform recognition failure: when the contextual meaning of symbolic systems (such as emoji) cannot be accurately analyzed across languages and cultures, the detection system relying on the common semantic model will miss the implicit hate speech, and ultimately lead to toxic content rampant in multilingual scenes (Saikh et al., 2005).
Twitter’s “sensitive media” filter is used by users to mask racist content. For example, when users post images or text containing discriminatory metaphors, they use the platform’s “sensitive content” tag to circumvent censorship, and the algorithm is only based on user-initiated tagging or keyword matching, and cannot identify the racist intent behind the content. This technical design reduces language to marketable symbols (such as whether to check “sensitive media”),
but ignores the power game of language in specific contexts – for example, insulting expressions against indigenous people may be disguised through humor, metaphor, etc., and the algorithm lacks a library of cultural context. It cannot identify its discriminatory nature (Matamoros-Fernandez, 2017).
- The difficulty in eradicating hate speech
The vagueness of the definition of hate speech leads to the regulatory dilemma, the core of which lies in the unclear conceptual boundaries and excessive generalization, resulting in the dilution of “systematic discriminatory speech” that needs to be regulated (Gelber, 2019).
In public discourse, the category of “hate speech” has been arbitrarily expanded to include extreme abuse of ordinary offensive speech, causing regulatory targets to lose focus. For example, Australia’s regulation of “racial insults”, Indonesia’s “dissemination of profane videos”, and the UK’s proposed classification of “hostility towards men” as hate crimes blur the essential distinction between “systematically discriminatory speech” (institutional harm based on structural discrimination) and individual conflict speech.
The technological governance of social media platforms exposes the definition bias of mechanical division. The Facebook algorithm prioritizes the protection of broad groups such as “white people” while ignoring targeted discrimination in subgroups such as “black children,” showing that a standardized classification of “protected categories” fails to capture the complex context of systemic discrimination (Gelber, 2019, p.5,17).
At the same time, the opacity and data deviation of the platform audit has intensified the contradiction: the “third nature” content is mistakenly deleted, and the audit standards are not uniform, which is essentially the conflict between the industrial standardization model of “headquarters decision-making – regional implementation” and the multicultural context. For example, Meta set up the Singapore headquarters in the Asia-Pacific region but retained the decision-making power of the California team, resulting in Filipino users’ complaints of “regional discrimination” having to rely on the review of American cultural consultants, and the governance logic separate from local culture exacerbated the dislocation of systematic discrimination identification (Sinpeng, 2021).
- Under the economic orientation, algorithms promote cultural conflicts
There is “implicit bias” in Google’s search algorithm, which exposes the hypocrisy of technology neutrality. This is not a technical error, but the inevitable business model – the ACCC 2019 report pointed out that the release of personalized advertising is the main revenue of the platform, and hate speech is always more likely to attract the public to click to read and feedback, and the time spent on these messages is always more, so the platform from its own interests, The algorithm chose “user retention” over “content security.” (Australian Competition and Consumer Commission, 2019).
Silicon Valley sees “free minds and free markets” as the essence of the Internet, arguing that government should give way to market and technological autonomy. For example, the multistakeholder governance model promoted by the United States (such as the establishment of ICANN) emphasizes that non-government entities (enterprises and civil organizations) dominate Internet rules, essentially imposing the American concept of “small government, big market” on the world. At the same time, Silicon Valley technology giants often refuse to take responsibility for the platform content on the grounds of “technology neutrality” and “freedom of speech”,
but in fact, their algorithm design and content review standards (such as the treatment of hate speech and fake news) imply American-style liberal values and ignore the differences in the definition of “harmful content” in other societies. It is also the arrogance of cultural perception – the idea that Silicon Valley values can be used as the norm to define every inch of global culture (Flew, 2021).
- The problem that platform governance needs to face is the game between profit and responsibility
The lack of governance responsibility of the platform is mainly reflected in the disregard of “profit first” and “social risk”. In the Christchurch, New Zealand, shooting in 2019, Facebook allowed live video to spread, exposing its inertia in reviewing user-generated content – despite the platform’s advanced image recognition technology, it delayed processing violent content for fear of interfering with user engagement. The review team shelved the intervention for fear of hurting advertising revenue. This “regulatory arbitrage” strategy essentially involves platforms taking advantage of differences in governance capacity between countries to shift the social costs of high-risk content to areas with weak regulation (Flew, 2021).
In the review of social media content, AI technology assistance has limitations, and the review of complex content is still highly dependent on manual labor. Globalization makes the content of the platform present multi-language and cross-cultural characteristics, which increases the work burden of auditors. According to Roberts (2019), Meta’s outsourced auditors handle 1,000 reports per day for only $2.30 per hour. At work, they often rely on Google Translate to process content, and can only rely on limited cultural knowledge to guess specific words, which makes the misjudgment rate of “culture-specific discrimination” as high as 55 percent.
In addition, the auditor labor rights protection is lacking. Most auditors are employed by third-party contractors, lack social security and psychological support, and find it difficult to protect their rights. Microsoft and Facebook auditor lawsuits are typical. What’s more, they face a mental health crisis. Meta’s Kenyan outsourcing company, Samasource, requires auditors to sign waiver agreements to avoid liability. According to a 2025 lawsuit, 81% of 144 moderators who underwent psychological evaluations were diagnosed with “severe PTSD,” dealing with 600 pieces of extreme content per day and making decisions within 30 seconds (Roberts, 2019). This shift of governance costs to lower-class workers in developing countries is essentially a new form of “digital colonization.”
The technical design of digital platforms often reduces cultural symbols to binary data (e.g., labels, keywords), and its logic is centered on business efficiency rather than cultural sensitivity, which is essentially a digital projection of the real power structure (Couldry & Mejias, 2018). In South Korea’s “Free the Body” movement, topless protest photos of women were frequently deleted due to the platform’s decision to “violate the body privacy policy” (Roberts, 2019), but the naked content of men’s fitness was not restricted, this algorithmic double logo is a digital reproduction of the gender power structure. (Gelber, 2019). These cases show that platform rules are tacitly based on Western culture, such as Facebook’s removal of images of naked women from Australian Aboriginal ceremonies for “sexual innuendo” (Matamoros-Fernandez, 2017), ignoring its cultural sanctity, and exposing the systematic misreading of marginalized groups’ expressions by technological design.
- When algorithms encounter power structures
The platform’s “global governance” relies on the myth of “technology neutrality,” which imposes Western legal and ethical standards on the world. For example, the document mentions that Facebook and Google’s Terms of Service require users to unconditionally accept their rules, but ignore local cultural needs. Emphasizing that data colonialism eliminates cultural diversity through “technological rationality,” such as “algorithms that reduce social life to computable binary data and refuse to acknowledge the incommensurability of multiculturalism” (Couldry & Mejias, 2018).
Digital platforms use algorithms to filter user activity through “election” to influence content online visibility. The platform will prioritize the display of so-called “relevant” content based on user interaction data, and similar controversial or negative information is often judged by the algorithm as “user interest matching” because it is more likely to trigger clicks and comments, to obtain higher transmission weight. This mechanism makes the natural transmission of negative information or hate speech potentially higher than that of ordinary content, just as the platform algorithmically enhances the visibility of such content on the grounds of “user interest” (Keskin, 2018).
Data colonialism reinforces systemic bias through “standardized categorization” platforms that reduce complex social behaviors to quantifiable labels (such as “race” and “religion”) through algorithms, ignoring the unique dimensions of non-Western cultures. For example, data classification systems are often based on Western rationality, resulting in “non-Western cultural traditions being treated as’ anomalous data ‘in algorithms.” The essence of data double standards is a hegemonic projection of Western values: The platform’s nuanced classification of issues of Western concern (e.g., LGBTQ+) and lack of understanding of other cultural issues (e.g., monarchies in Southeast Asia, indigenous cultures) is essentially a “digital continuation of colonial-era cultural hegemony” (Couldry & Mejias, 2018).
- Suggestions and measures
Building regional decision centers, Facebook has strengthened its moderation capabilities in Burmese and minority languages in the wake of the Rohingya crisis, hired more local moderators, and worked with international organizations to increase cultural sensitivity.
1. Meta’s Asia Pacific Culture Lab in Singapore has been in operation for three years, and its core innovation is the “dual-track review system” : common content is handled by the local team, and culturally sensitive cases are “tripartite review” (platform, NGO, community representatives) (Sinpeng, 2021).
2. Learn from Australia’s regulatory ideas in the digital advertising market (ACCC, 2019), a “cultural impact special fund” system can be established: Australia’s regulatory practice in the digital advertising market shows that the “proportional provision + effect binding” mechanism can effectively balance the platform’s commercial interests and cultural governance responsibilities. Drawing on the analysis of the market power of digital platforms by the Australian Competition and Consumer Commission (ACCC, 2019), a “special fund for cultural impact” system can be established to tie the economic benefits of platforms to cultural responsibilities:
According to Flew’s “polluter pays” theory, platforms with annual advertising revenue of more than $50 million are required to set aside 1.5 to 3 percent of the funds (referring to Australia’s “Safe Design Principles” resource allocation model) for:
Local cultural data infrastructure: such as the construction of the Australian Aboriginal language corpus and the development of the Southeast Asian dialect audit model, to solve the problem of one-way flow of cultural data caused by “data colonization” (Couldry & Mejias, 2019).
Finally, the governance dilemma of digital platforms is essentially a collision between the standardized thinking of industrial civilization and human cultural diversity. To break the dilemma, it is necessary to reshape the cultural perception of technology and build a “cultural intelligence” system. Tech giants should abandon the arrogance of “global uniformity”, respect the uniqueness of each culture, and create a unique governance solution for it. The value of technology is not to create a homogeneous digital space but to ensure the equal development of cultures in a digital world where different languages and cultural symbols are respected (Flew, 2021).
So when the platform’s algorithms begin to understand that “difference is not a problem, but the nature of the world,” when the pursuit of profit and cultural respect form a symbiotic relationship, we can truly cross the cultural divide in the digital age and achieve digital equality. Let technology be a bridge that connects people, not a wall that divides them.
References
Australian Competition and Consumer Commission. (2019, July 26). Digital platforms inquiry – final report. Www.accc.gov.au. https://www.accc.gov.au/about-us/publications/digital-platforms-inquiry-final-report
Couldry, N., & Mejias, U. (2018). Nick Couldry and Ulises Mejias Data colonialism: Rethinking Big data’s Relation to the Contemporary Subject Article (Accepted version) (Refereed). https://eprints.lse.ac.uk/89511/1/Couldry_Data-colonialism_Accepted.pdf
Flew, T. (2021). Regulating Platforms. John Wiley & Sons.
Gelber, K. (2019). Differentiating hate speech: a systemic discrimination approach. Critical Review of International Social and Political Philosophy, 24(4), 1–22. https://doi.org/10.1080/13698230.2019.1576006
Keskin, B. (2018). Van Dijk, Poell, and de Wall, The Platform Society: Public Values in a Connective World (2018). Markets, Globalization & Development Review, 03(03). https://doi.org/10.23860/mgdr-2018-03-03-08
Kim, J. (2017). UNDERSTANDING ADULT IMMIGRANTS’ LEARNING IN SOUTH KOREA: DETERRENTS TO PARTICIPATION AND ACCULTURATIVE EXPERIENCES. https://getd.libs.uga.edu/pdfs/kim_jihyun_201712_phd.pdf
Matamoros-Fernández, A. (2017). Platformed racism: the mediation and circulation of an Australian race-based controversy on Twitter, Facebook and YouTube. Information, Communication & Society, 20(6), 930–946. https://doi.org/10.1080/1369118x.2017.1293130
Roberts, S. T. (2019). Behind the screen : content moderation in the shadows of social media. Yale University Press.
Saikh, T., Barman, S., Kumar, H., Sahu, S., & Palit, S. (2005). Emojis Trash or Treasure: Utilizing Emoji to Aid Hate Speech Detection. https://aclanthology.org/2024.icon-1.64.pdf
Sinpeng, A. (2021). Facebook: Regulating Hate Speech in the Asia Pacific. https://r2pasiapacific.org/files/7099/2021_Facebook_hate_speech_Asia_report.pdf
Be the first to comment