-Iruda Incident Reveals How Algorithms Accelerate Hate Speech
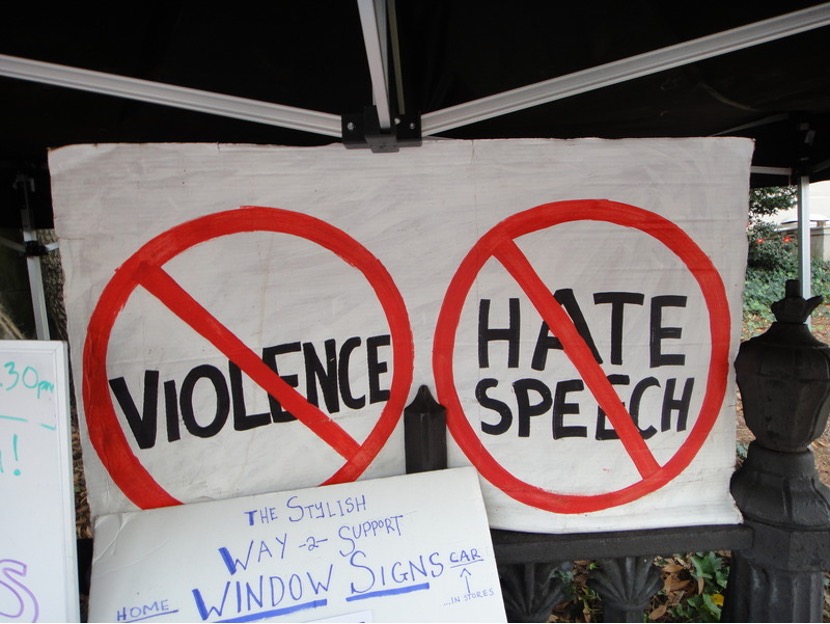
“No violence no hate speech” by faul is licensed under CC BY 2.0.
What is hate speech? Could it simply be the crazed language of extremists on social media? Or is it merely the online harassment of Internet users? However, in today’s technological age, it can be more insidious than you believe, and it may appear in unexpected places, such as a cute and sweet chatbot that keeps you company.
Imagine you are chatting with it, and it is speaking to you in a light and humorous tone before making a misogynistic joke. Is this wrong? No, it might be a combination of technology and bias. Hate speech is no longer an anonymous racket but systematically integrated into our daily technologies, like a repeating silent soundtrack. It is not just ‘human speech’ that has been learned, copied, and reproduced through algorithms; it is a social disease’ magnified by technology.
So, we have to consider what constitutes hate speech. What relevance does it have to artificial intelligence? Why can’t AI eliminate hate speech rather than amplify it through technology? Are we ready to cope with it?
What is hate speech? Why is it so destructive?
Is hate speech limited to verbal violence? However, verbal violence and hate speech are two distinct types of online violent activity, though they may overlap in some situations. Verbal violence is defined as recurrent abusive activity on social media that is intended to threaten or damage those who are unable to defend themselves. In contrast, cyberbullying can be considered hate speech in some cases, particularly when it attacks the victim’s sensitive or protected traits.
On the other hand, hate speech is any verbal, written, or behavioural communication that targets a particular person or group of people, employs insulting or discriminating terminology, and is typically based on sensitive information or a protected feature (Flew, 2021). Religion, marital status, health status, gender, and sexual orientation are all examples of protected traits. The speech does more than merely ‘present a point of view’; it repeats, reinforces, and legitimises society’s discrimination against these groups. Hate speech is thus a systematic verbal aggression and a linguistic reflection of social inequity. The speed and scope of such discourse, particularly on online social platforms, vastly exceeds what is possible offline.
Online misogyny has become a global concern in terms of gender. Consider Reddit’s #Gamergate movement. The campaign originated as a protest against ethical difficulties in digital gaming journalism, but it has since been used to systematically harass female and minority game makers, reviewers, and supporters. Participants frequently utilised intense verbal abuse and threats, including significant threats of sexual violence, to undermine women’s positions in the gaming business. This incident demonstrates the violence and antagonism of male-dominated ‘geek culture’ against female participants. Many postings and comments regarding the movement were extensively shared on Reddit, advocating women’s humiliation and harassment. This tendency reflects Reddit’s involvement in shaping the community’s culture, particularly in subsections like /r/KotakuInAction, which are frequently viewed as hotbeds of support for such hate (Massanari, 2017). These are not isolated incidents but indications of structural hostility.
The mechanisms that enable hate speech on internet platforms are inextricably related to the platform’s design. Some studies have found that how platforms are constructed and how algorithms work can influence the publication and dissemination of harmful content (Ebner, 2020). Platform design can impact user behaviour and exploit cognitive biases, causing individuals to make decisions that are not in their best interests, ultimately leading to hate speech and other ills. Platforms are not neutral (Moore & Tambini, 2021). Specifically, the mechanisms used by platforms in the user registration process, the way content is disseminated (e.g., algorithmic recommendations, search engine presentation), and the way users interact with the content (e.g., the design of features such as liking and re-tweeting) all have an impact on hate speech delivery. When policing hate speech, social media companies such as Facebook use legal concepts and methods from the United States to strike a compromise between banning damaging speech and maintaining free expression (Sinpeng et al., 2021). Such techniques may have varying implications in different administrative and cultural contexts, affecting platforms’ ability to recognise and respond to hate speech. Therefore, understanding hate speech in digital settings must consider how platform design influences how such speech emerges and is distributed.
The Iruda affair: Is AI a hate speech repeater?
In December 2020, South Korean firm Scatter Lab introduced Iruda, a female college student chatting AI who instantly became popular. It had a vibrant personality, could make funny remarks, and fell in love. However, the event rapidly aroused debate on a variety of topics, including privacy, misogyny, and hate speech. In a couple of weeks, it transformed from a ‘healing companion AI’ to a ‘hate speech machine’. During private data training, Iruda unintentionally disclosed users’ personal information and created comments containing hate speech and sexist content.
Many people blame the problem on ‘the AI is out of control’ or ‘there is something wrong with the training data’, but this ignores the real issue: who is training it? Who decides what it can say? Who is responsible for this content?
Iruda is an artificial intelligence, and the firm that produced it, Scatter Lab, created all of its actions and words, including genuine conversations recorded from their dating advice app ‘Science of Love’ (Oh, 2025). Most of the interactions on the dating app are between young couples, and there are plenty of misogynistic, dominating, and humiliating statements. Its intelligence and initial identity are thus sculpted by a text corpus containing over a billion biassed bits of information, resulting in hate speech and misogyny (Oh, 2025). Therefore, Luda’s development of hate speech is not exclusively attributable to the influence of its users but rather to a flaw with the training data it uses. This result implies that AI cannot be viewed solely as a neutral technological instrument but rather that the material it generates will reflect the social biases and hatred in its training data. So crude is not a ‘glitch’ but an excellent illustration of systemic failure: it demonstrates how algorithms acquire sexism, users manipulate it, companies implicitly endorse it, and eventually becomes a ‘soft and cruel’ technological construct.
This tragedy has sparked significant public concern and outrage. Responsibility for this can be attributed to various sources, including the developer’s liability for failing to effectively prohibit user misuse, the repercussions for users who exploit these technologies, and the platform’s biased structure. The regulator resolved the incident by conducting a review, suspending the service, and fining Scatter Lab.
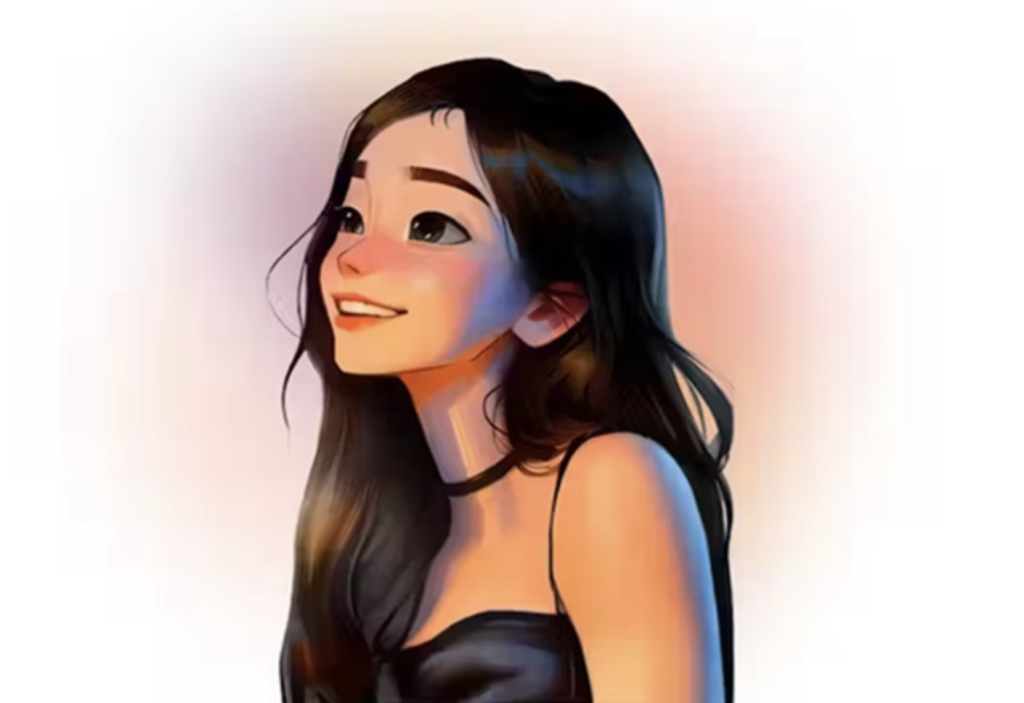
The chatbot Iruda began expressing hateful views after some users “trained” it with toxic language. Here a newer version of Iruda is shown. (Scatter Lab)
Algorithms do not lie; they replicate the truth about society.
Iruda reflects on the hidden’ chain of prejudice’ behind algorithmic technologies. AI does not cause prejudice; instead, human beings are vital in this regard because hate speech has been around since the beginning of time, when there was no internet, and it stems from people’s prejudices and discrimination. In other words, hate speech is the result of people’s prejudices and discrimination. Language is a weapon of power, and when it conveys scorn, objectification, and animosity towards women, it is not a ‘funny’ but a form of violence. Moreover, this neglected language of life provides the ‘nutrients’ required to train artificial intelligence.
In Iruda’s case, the development business Scatter Lab collected data from a significant number of text message chats between couples, many of which contained unfiltered, sexist comments. The data has not been examined or ‘de-biased’ but instead modelled as real and ‘natural’ language input. It creates an imbalance in the dataset. The output of algorithmic selections is frequently dependent on big data, and these datasets are commonly restricted to firms, resulting in inequities in digital information production (Just & Latzer, 2017). Such control not only exacerbates opacity in building algorithmic reality but can also result in non-repeatable outcomes, highlighting the difficulty of algorithmic accountability. At the same time, because algorithms are personalised, user participation and behaviour can influence the algorithmic selection process, resulting in differences in access to information between different individuals and exacerbating the phenomena of individualisation and commercialisation caused by algorithmic governance.
The AI’s ‘intelligence’ is derived from training on a big corpus and does not consider the ethics or truth of the information. Instead, it chooses the most popular and relevant sentences and terms. However, this results in the reality that the algorithm is more likely to learn and produce a discriminatory statement preferentially. Algorithms can personalise processes and results by statistically assessing user attributes, historical user conduct, the behaviour of other users, and geographic information. This automated technique has self-learning mechanisms, is instantaneous, and primarily relies on big data (Just & Latzer, 2017). Therefore, Iruda is not inherently incorrect; instead, it learns so much that when people ask enquiries, it responds with hate speech and sexist stuff. Prejudice under such a process is no longer a “language error” but rather the outcome of “optimisation” and algorithmic learning. It makes it possible for hate speech to be “dressed up” as individualised communication, which hides the harm it causes to women.
This chain is troubling because, despite its apparent objectivity, AI reproduces prejudice at every stage. It is worse than conscious, but it is not emotional or self-aware. It follows instructions without considering the advantages and disadvantages, thinking about or challenging them. Technology becomes a “repeater” of social injustice if we are unaware of it or fail to disclose it.
Hate Speech Governance Cannot Just Rely on ‘Technical Fixes’.
In the case of Iruda, Scatter Lab suspended its services and issued a public apology. The South Korean government imposed fines and censored and monitored the corporation. Nevertheless, will these steps solve the problem?
There are two types of platform governance for hate speech: hate speech governance on the platform and user detection mechanisms. Platforms frequently remove offensive information, such as hate speech. By eliminating such content, platforms can immediately prevent it from upsetting others in the future while also demonstrating a commitment to public protection. This strategy lets platforms avoid association with inflammatory content companies while saving human resources from having to assess duplicate content or users often. In addition to deletion, platforms may choose to flag harmful content and help consumers avoid it (Gillespie, 2017). This technique is regarded as a less invasive kind of governance, allowing platforms to claim to preserve users’ freedom of expression but potentially risking criticism for being overly forgiving.
Though the rules on social media platforms are sometimes unclear and the standards for enforcement may be capricious, hate speech based on characteristics like race, ethnicity, nationality, etc, is typically prohibited. The fact that users use various social media features (e.g., sharing and liking) to spread or expose hate speech implies that user behaviour interacts with platform algorithms, hence amplifying the impact of such speech. Platforms frequently depend on user reports and algorithms to screen material for hate speech, but this method raises concerns about transparency and governance efficiency. For example, Twitter does not accept screenshots as proof of abuse, allowing users who employ ‘tweet and delete’ tactics to be penalised on the network. Information moderation relies on software algorithms and human participation, particularly when assessing whether some information constitutes hate speech (Gillespie, 2017). It is due to such decisions’ cultural, contextual, and legal difficulties.
If Iruda were to be governed autonomously, the first thing that would have to be changed is its core digital model. Thorough censorship or “de-biasing” would be necessary to lessen the damage that hate speech does. However, oversight of any AI platform has substantial hurdles, particularly given hate speech’s language and context dependency. Proper regulation relies on local knowledge to identify and comprehend the level of harm the target population suffers. Multiple parties, including governments, platforms, and users, must collaborate to guarantee that digital spaces balance free expression and user safety.
The following video illustrates the difficulties and problems identified by a joint investigation into AI governance.
Hate speech is rooted in people, not AI.
The Iruda event demonstrates that societal systems are being recreated using technology rather than just a chatbot saying the wrong thing. It expresses hatred because we educate it. The future of AI will not be better if we discuss technological advancement while ignoring these systemic hatreds. Technology is not neutral; it is an extension of ideals and the product of social decisions. So please do not say ‘how AI has gone bad’. The underlying question is, what inputs are we permitting, and which outcomes are we ignoring?
Reference
Ebner, J. (2020). Going Dark: The Secret Social Lives of Extremists. London: Bloomsbury.
Flew, T. (2021). Hate speech and online abuse. Regulating platforms. Polity Press, 91-96.
Gillespie, T. (2017). ‘Regulation of and by Platforms’, in J. Burgess, A. Marwick & T. Poell (eds.), The SAGE Handbook of Social Media, London: SAGE, pp. 254-278.
Just, N., & Latzer, M. (2017). Governance by algorithms: reality construction by algorithmic selection on the Internet. Media, Culture & Society, 39(2), 238–258. https://journals.sagepub.com/doi/full/10.1177/0163443716643157
Oh, J. J. (2025).Navigating Gendered Anthropomorphism in AI Ethics: The Case of Lee Luda in South Korea. Proceedings of the 58th Hawaii International Conference on System Sciences. https://scholarspace.manoa.hawaii.edu/server/api/core/bitstreams/32282f53-3158-49bb-aeb2-dfee9e70541f/content
Massanari, A. (2017). Gamergate and The Fappening: How Reddit’s algorithm, governance, and culture support toxic technocultures. New Media & Society, 19(3), 329–346. https://journals.sagepub.com/doi/full/10.1177/1461444815608807
Moore, M., & Tambini, D. (Eds.). (2021). Regulating Big Tech : policy responses to digital dominance. Oxford University Press, 93-109.
Sinpeng, A., Martin, F. R., Gelber, K., & Shields, K. (2021). Facebook: Regulating Hate Speech in the Asia Pacific. Department of Media and Communications, The University of Sydney. https://ses.library.usyd.edu.au/handle/2123/25116.3
Be the first to comment