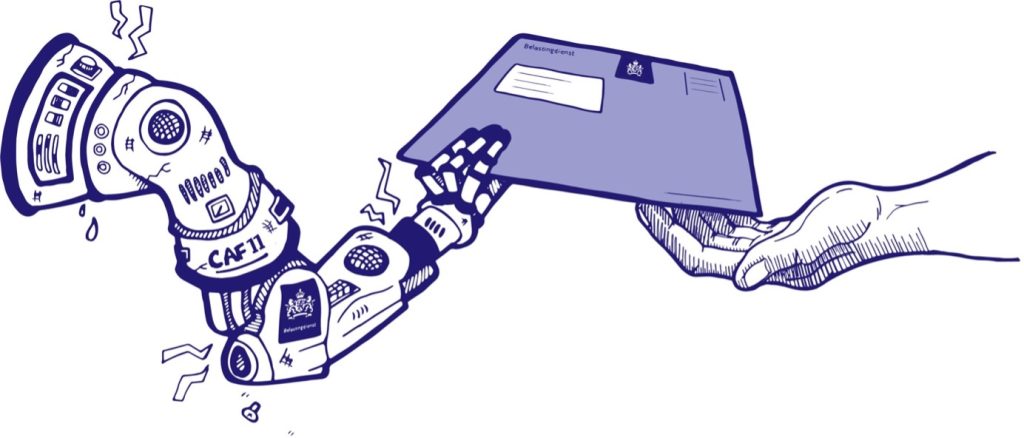
The Dutch childcare benefit scandal
A classic blue envelope characterising the Dutch Tax Authority (DTA) was delivered to the homes of parent in The Netherlands. Though wrapped in bureaucratic jargon, the message inside was clear: “You are a fraud.” This is the beginning of what would be one of Europe’s biggest government scandals in recent decades, the Dutch childcare benefits scandal, or “toeslagenaffaire.”
Between 2013 and 2021, thousands of parents would receive this crushing verdict. In a letter, they were informed that they needed to repay years’ worth of childcare benefit—ranging from tens to hundreds of thousands of euros— plunging families into financial ruin.
But the damage was not just financial. Under the stress of this sudden crushing debt, victims lost their jobs and homes, marriages crumbled, and government statistics later revealing that over 11.000 children were evicted from their homes.
What these parents couldn’t know was that many had been flagged by an algorithm programmed to hunt for fraud based on risk factors that included having a non-Dutch nationality. They had no way to understand why they’d been targeted, no meaningful way to appeal, and nobody believing in their innocence.
And now, 7 years since the start, victims continue to struggle to find answers about why they were targeted. The Tax Authority’s recovery unit (UHT) — A new government branch that was created in response to the scandal to aid victims— has recently stopped providing victims with their complete personal files, making it nearly impossible for many to understand exactly why they were wrongfully flagged as fraudsters.
So, what caused these victims to be seen as frauds and what was the government’s role in the scandal? These questions will be explored in this blog post where we take a deeper dive into the scandal and the surrounding context.
Turning prejudice into policy
At the centre of the scandal lies an algorithmic decision-making programme that was responsible for flagging potential frauds. This programme—installed in 2013— was the result of the intensifying political pressure crack down on welfare fraud in the early 2000s after it became apparent that Bulgarian criminals were commiting fraud by receiving Dutch welfare. In an attempt to prevent something like that to happen again, the government put into place new strict measures—amoung which was the deployment of the automated fraud detection model.
Blindfolded by design
The model that was created is a so-called risk classification model. These types of models are trained to sort information into designated classes. For this case, this could be ‘fraud’ and ‘not fraud’ for example. The model also included self-learning elements, meaning that it learns to draw conclusions not based on human guidance, but by laying connections throughout the information themselves (Belcic, 2024). The key advantage of such a system is that they continue to learn from experience over time and making it much harder to trick the system. However, self-learning models often become ‘black box’ models. And this is exactly what happened in The Netherlands as well.
The combination of self-learning and bias training data essentially created a discriminatory feedback loop within the algorithm. The tax authorities had used information on nationalities, race, criminal record, and other discriminatory data points as part of their training set. As a result, people with matching information received higher risk scores and were designated as frauds.
The Dutch policy against fraud was strict, extremely strict. A single missing signature or incorrect date would be seen as fraud and cause parents to lose access to their benefits. But in typical government fashion, this would only be communicated to the person moths or even years after the fact, causing the repayment debts to stack up hidden from view. To add insult to injury, when victims asked to see the reasoning why they were flagged as frauds, they were presented with redacted reports.
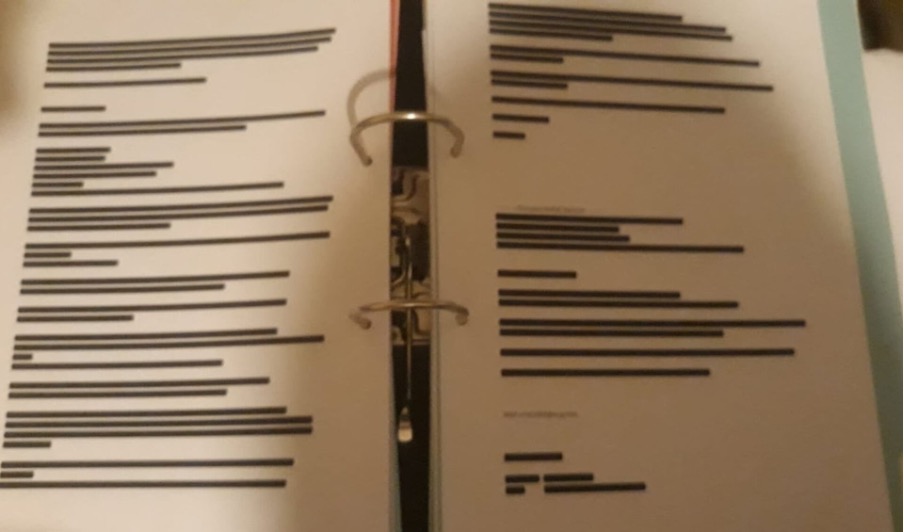
The model was developed to have the same all-or-nothing attitude towards potential fraud; a single improper variable or risk factor was enough for the system to flag you as a potential fraud. Human tax officials were supposed to review each flag manually. However, the sheer number of flags made this security measure doomed from the start. In addition, these officials would receive little to no information as to why the algorithm has flag the person as a fraud. This essentially created the perfect storm of unaccountability where the DTA blindly trusted the algorithm without any proper oversight.
Incentives to Punish
However, blindly accepting the model’s decisions wasn’t just convenient for the DTA, it was incentivised. For you see, developing such models is expensive—and, especially in 2013, novel and untested— The DTA had to prove to the government that this algorithm was effective at reducing costs of fraud. In a perverse twist of fate, the model wasn’t used to mitigate monetary loss from fraud, it became an incentive to seize as much money from the flagged frauds as possible to make the system appear profitable.
This resulted in an environment where people were incorrectly flagged as frauds due to things as their ethnicity or because they had a double nationality, had their childcare benefits seized, and were systematically ignored or sent away when seeking answers.
The breaking point: Silence into scandal
While the systematic injustice remained hidden for many years, the first cracks started to appear in 2014 when the CAF-11 case came to public. This was the 11th round of inspections by the tax agency’s anti-fraud department. However, this time, the victims fought back collectively. A whopping total of 116 court cases ensued between the Dutch government and the victims. As more and more victims spoke out, it became clear that something was very wrong within the governing body.
Various investigations were started, with each one condemning the tax authority on their harsh treatment and unwillingness to help victims. The turning point came when news outlets revealed that the fraud-detection algorithm was essentially engaging in ethnic profiling.
At this point, not just the victims but the entire population was furious, sparking protests and political debates. The final nail in the coffin would come in 2020 when an external committee presented their report “Unprecedented Injustice”. This report concluded that the tax authority’s actions were unlawful on multiple fronts. It was in direct violation of the EU data protection laws and the right of non-discrimination as described both in the constitution as well as the European Convention on Human Rights.
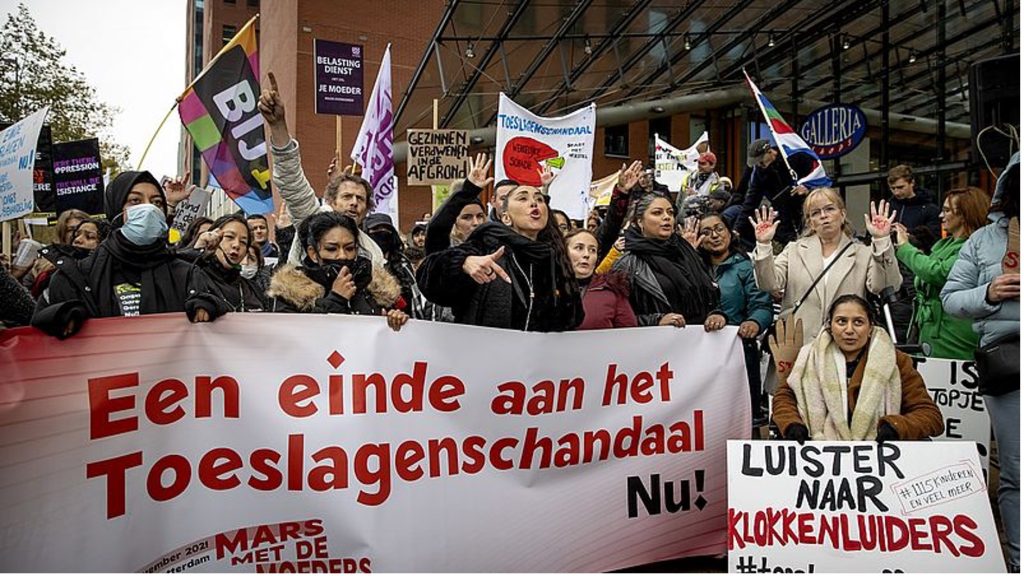
With no other way out, the complete Dutch cabinet resigned in 2021, acknowledging the damage and assuming collective responsibility. The government also committed to compensate each victim. The total compensation package is already costing roughly 9.3 billion euro and is expected to grow with 1 to 5 billion extra.
“The rule of law must protect citizens from an all-powerful government, and that went terribly wrong here”. -Prime minister Mark Rutte announcing the resignation of the Dutch cabinet [Rijksoverheid]
Beyond the financial compensation, the scandal prompted systematic reforms. The tax authority underwent a complete restructuring, and algorithmic-impact assessments became mandatory for implementing algorithmic based tools within the government.
A global pattern of policy failure
What began as an attempt to prevent fraud, transformed into a system that itself defrauded its citizens of an essential part of their lifelihood. And while this scandal at a first glance looks like one born out of technology, it is institutional of governance like wrong choices, incentives, and idleness that allowed the algorithm to have such a profound impact.
Sadly, this path from good intentions to destructive consequences is all too common with more and more social welfare becoming data-driven within many countries. AI’s impact on the right to equality and non-discrimination is one of the most frequently reported incidences pertaining to algorithms. While the Dutch scandal can be seen as especially impactful, it too is not a unique incindent.
Similar cases have occurred, and likely will continue to occur, across countries around the world. Bouwmeester (2023) highlights similarities between the Dutch case and the Nav scandal in Norway, the MiDAS scandal in the USA, and the Robodebt scandal in Australia. These “enforecement fiascos”, as Bouwmeester calles them, follow a similar pattern. He explains that the increasingly penalising and conditional nature of many policy enforcements create the perfect conditions for policy failure and algorithmic harm.
A biased algorithm may be the bomb, but it’s the lack of digital governance that lights the fuse. Closing up the pocliy-making process and concentrating authority, and limiting the posibility to think about decisions are a recipe for large-scale policy failure of which the Dutch fiasco is a perfect example.
More broadly, this case highlights the growing issue of automation bias within many ciritcal organisations such as governments. The algorithms decisions are taken at face-value typically without any further evaluation.
“AI is neither artificial nor intelligent” -Kate Crawford [Atlas of AI]
As Kate Crawford—a renowned AI researcher—puts it, “AI is neither artificial nor intelligent”. In essence, AI is just a series of calculations to measure a probability. There is no conscious or reasoning that considers the context around the decision-making process. Even self-learning models are inherently an extension of human decisions and biases that seep through training data and rules imposed by the developers.
The blind trust in these systems simultaneously concentrate authority while diffusing accountability.
Towards responsible algorithmic governance
The path forward requires a reimagining of algorithmic governance—one that places human wellfare at its center rather than robotic efficiency. As it seems now, technology informs governance, not the other way around. This inversion of priorities has shown to time and time again to undermine democratic accountability and threatens the rights of its most vulnerable citizens.
The lesson is clear; we need to get back to human-focused governance policies where decision-making tools are just that—tools, not judge, jury, and executioner.
A key aspect of this will be transparency. Transparency already serves as one of the cornerstones of good governance; but is especially important when implementing decision-making algorithms within the public sector. As we have seen in the case, a lack of transparency makes it near impossible to verify algorithmic decisions and allows potential biases to remain undetected.
Once models are transparent, it will be posible to establish the proper monitoring and oversight mechanisms. It should be the responsibility of the model developers to identfy any potential impact their system could have on human wellfare. But that does not mean they should face this task alone. Collaboration with regulatory bodies, vulnerable groups, and even the general public is required to ensure a level of equality between those who create the system and those who are subject to it.
Does data-driven welfare have a future?
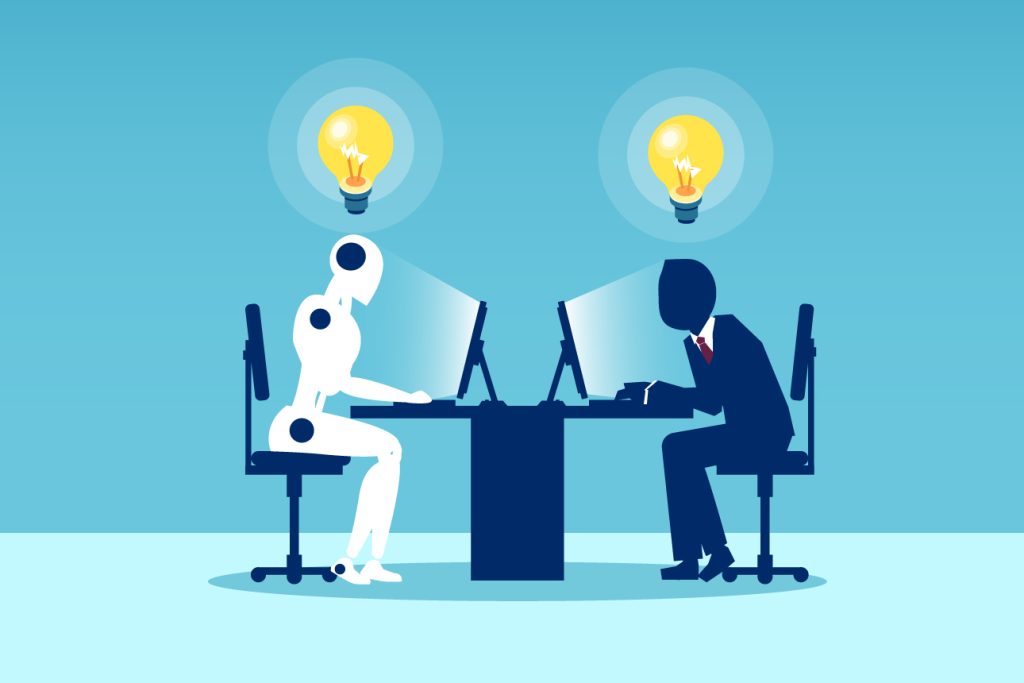
There is this concept of Technological Determinism that often surrounds discussions on AI where people see the implementation of AI and other technological innovations as a path to either a technological utopia or dystopia; But both extremes miss the mark.
All in all, AI isn’t some monkey’s paw that is doomed to cause problems or superpower that will herald in a new digital age. A sustainable future for AI is certainly possible—but has to be founded in human values. The Dutch childcare benefit scandal examplifies that algorithms themselves aren’t inherently good or evil; rather, it’s an echo of the surrounding governance frameworks and human decisions—magnifying their values and biases. And much like any revolutionary tool throughout human history—from fire to nuclear energy—it’s potential can be both negative and positive.
The key is therefore to embrace the algorithmic tools, but recognize and demand proportional responsibilities and governance.
We can already see the results of several promising approaches and legislations towards responsible AI implementation in various countries. For example, Finland introduced new legislations on automated decision-making within the public sector in 2023 that will require public-private partnerships for AI development. Similarly, Estonia is well on its way to become—perhaps the first— AI-driven personalised state, a possibility that is fueled by their comprehensive AI Support Toolbox and governance policies that protect democracy and trust in their digitalised society.
Creating comprehensive oversight mechanisms through collaboration and enforcing model designers to critically assess their solutions will allow us to harness AI sustainably to truly enhance public welfare rather than undermine it.
References
Algoritmes, Big Data en de overheid. (2025, April 4). Amnesty International. https://www.amnesty.nl/wat-we-doen/tech-en-mensenrechten/algoritmes-big-data-overheid
Amnesty International. (2021a). Xenophobic machines: Discrimination through unregulated use of algorithms in the Dutch childcare benefits scandal. In amnesty.org (EUR 35/4686/2021). Amnesty international. https://www.amnesty.org/en/documents/eur35/4686/2021/en/
Amnesty International. (2021b, November 1). Dutch childcare benefit scandal an urgent wake-up call to ban racist algorithms. https://www.amnesty.org/en/latest/news/2021/10/xenophobic-machines-dutch-child-benefit-scandal/
Artificial Intelligence 2024 – Finland | Global Practice Guides | Chambers and Partners. (n.d.). https://practiceguides.chambers.com/practice-guides/artificial-intelligence-2024/finland/trends-and-developments/
Belcic, I. (2024, November 25). Classification in Machine Learning. IBM. https://www.ibm.com/think/topics/classification-machine-learning
Bouwmeester, M. (2023). System failure in the digital welfare state. Recht Der Werkelijkheid, 44(2), 13–37. https://doi.org/10.5553/rdw/138064242023044002003
Flew, T. (2022). Regulating platforms. Polity.
Frederik, J. (2024, June 11). De compensatieregeling voor de toeslagenaffaire: onuitlegbaar, onuitvoerbaar, onbetaalbaar. De Correspondent. https://decorrespondent.nl/15377/de-compensatieregeling-voor-de-toeslagenaffaire-onuitlegbaar-onuitvoerbaar-onbetaalbaar/70474dc9-cd77-0461-2221-f63b9fa0cdc2
Kok, L. (2021, October 21). Kabinet: mogelijk meer dan 1115 kinderen in toeslagenaffaire gedwongen uit huis geplaatst. Het Parool. https://www.parool.nl/nederland/kabinet-mogelijk-meer-dan-1115-kinderen-in-toeslagenaffaire-gedwongen-uit-huis-geplaatst~b47b19d6/?referrer=https%3A%2F%2Fwww.google.com%2F&referrer=https%3A%2F%2Fkindertoeslagaffaire.nl%2F
NOS op 3. (2021, January 18). Toeslagenaffaire. De ellende uitgelegd. [Video]. YouTube. https://www.youtube.com/watch?v=rMDkzG9yHs8
Mäeots, K. (2024, March 13). AI use in public sector is a team sport. e-Estonia. https://e-estonia.com/ai-use-in-public-sector-is-a-team-sport/
Ongekend onrecht. (2020). In Ongekend Onrecht (pp. 1–132). https://www.tweedekamer.nl/sites/default/files/atoms/files/20201217_eindverslag_parlementaire_ondervragingscommissie_kinderopvangtoeslag.pdf
Be the first to comment