Introduction
We’re often told that AI is objective, data-driven, and colorblind. But what happens when the data it learns from is soaked in societal bias? Imagine typing “Black girls” into Google’s image search. Not long ago, the top results were filled with hypersexualized, stereotyped images—more harmful than helpful, more biased than neutral. This isn’t a random glitch. It’s a next level form of algorithmic discrimination, one baked into the everyday design of digital platforms
Today, platforms like YouTube, TikTok, and Google don’t just reflect culture—they shape it. Their algorithms determine what’s visible and what’s buried, who gets heard and who disappears. And because these systems are optimized for engagement, not equality, they often reinforce racial hierarchies in subtle, automated ways. It’s discrimination without a face, but with real-world consequences.
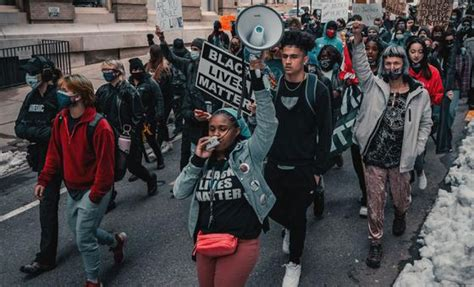
In this blog, I dive into how algorithmic bias persists—despite the illusion of AI neutrality—and how “colorblind” systems can still reproduce deeply racialized outcomes. Through real-world examples and academic research, I argue that algorithms are not just tools—they are cultural and political actors that demand scrutiny and reform.
How Is Bias Coded Into Algorithms?
We often hear people say, “AI doesn’t see race—it’s just math and code.” But here’s the truth: algorithms may not “see” skin color, but they can still learn racism.
Why? Because every algorithm learns from data—and that data reflects the real world. And let’s face it, our real world is full of inequality. So when machine learning models are trained on biased data, they don’t magically erase those biases. They replicate them. In fact, they often reinforce them—at scale and with speed.
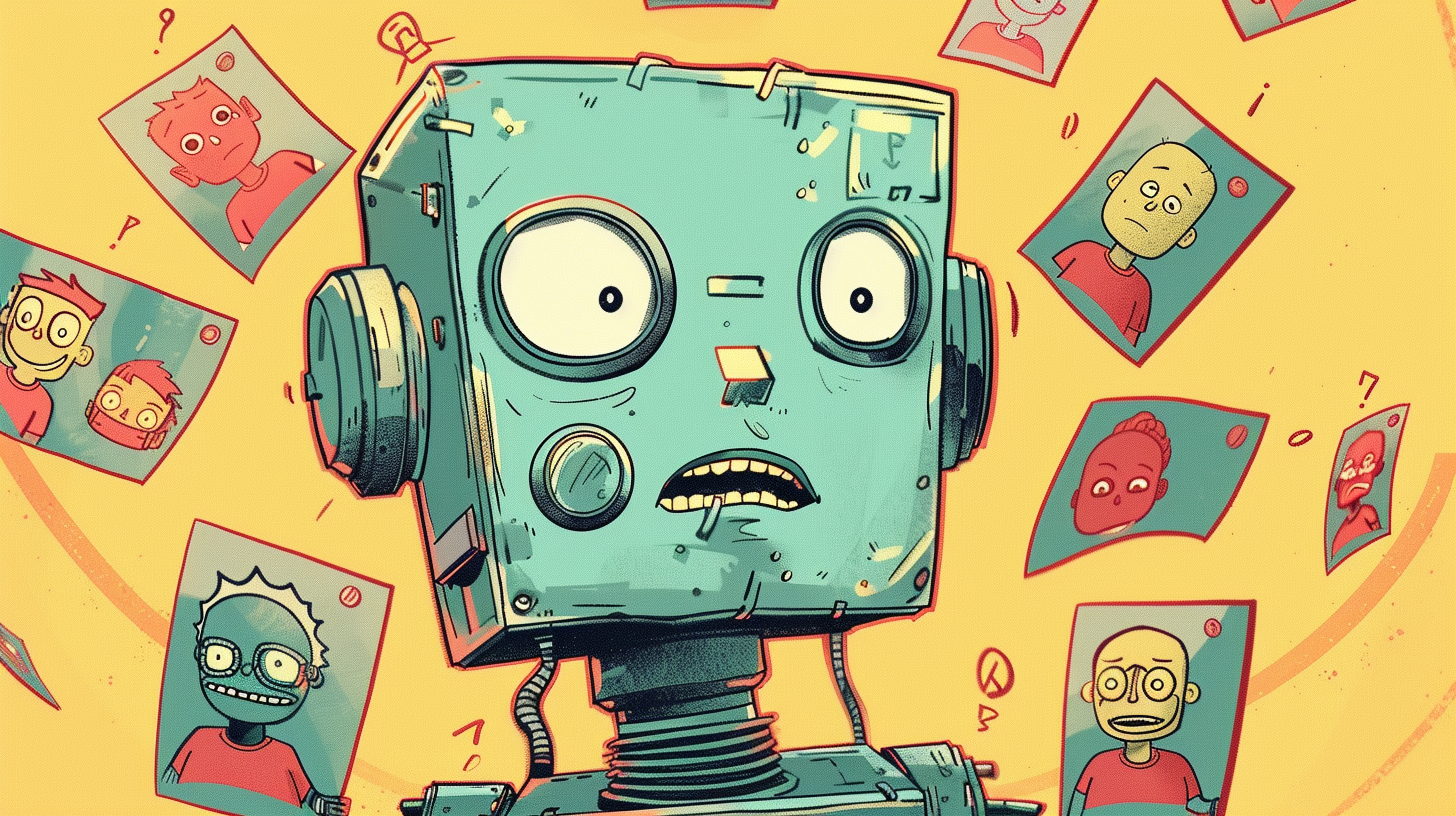
Kate Crawford (2021) refers to this phenomenon as the “politics of categorization”. She points out that every dataset carries a worldview. This means that when we build algorithms, we are actually encoding a judgment about what is important and what is not, who is counted and who is excluded. Algorithms aren’t just used to organize information-they also interpret it. And that, in turn, is the entry point for bias to creep in.
Let’s look at a few real-life examples:
Google Search Engine: Safiya Noble (2018) found that when you searched for “black girls” on Google, a large number of highly gendered, racially charged images used to appear — these images show stereotypes, not reality. This is not a systemic flaw, but a pattern. Algorithms are trained on biased, click-driven data. So what content is most likely to be clicked on? Often, it’s the worst content.
Amazon’s Recruiting AI: In 2018, Amazon was forced to shut down an internally-used AI recruiting tool because it systematically downgraded resumes that included the word “women’s,” such as “Women’s Chess Club.” Why? Because the model was trained using data from the last decade of male-dominated hiring. It “learned” that “male equals qualified” and automatically penalized any words that didn’t fit the pattern.
The logo of Amazon is seen at the company logistics centre in Boves, France, August 8, 2018.
Facial recognition: Joy Buolamwini and Timnit Gebru’s study “Gender Shades” revealed that commercial facial recognition systems had error rates as high as 35% for Black women, compared to under 1% for white men. These AI systems worked best for the faces they saw most—white, male, and Western. So no, bias isn’t just an edge case. It’s built in. And sometimes, it hides in plain sight.
Why does algorithmic bias happen?
The answer is uncomfortable but simple: because bias sells.
Most digital platforms – whether it’s YouTube, TikTok, Facebook, or Instagram – exist to do more than just “connect people” or “provide information”. “or provide information. Their core purpose is to make money. And what’s the most profitable way for them to do that? It’s user engagement. The longer you stay on the platform, the more ads they can show you. This is the basic logic of the “attention economy” (Andrejevic, 2020).
But the question arises: what kind of content makes people stay the longest? Certainly not calm, balanced, rational information. Platforms have “learned” that the more controversial, emotional, and hyperbolic content – especially content that reinforces stereotypes or provokes anger – the more clicks it gets, the more shares it gets, and the more profit it makes. the more it is shared, the more profitable it becomes (Pasquale, 2015; Noble, 2018). And this, in turn, is where bias creeps in. It’s not that some programmer in Silicon Valley is deliberately trying to spread racism, but that the algorithms themselves are designed for “results” rather than “fairness”. As Frank Pasquale points out in The Black Box Society (2015), tech companies intentionally hide the logic of how their algorithms work from the public in order to protect their business interests. This is because once it is publicly transparent, it means taking responsibility – and responsibility, in turn, can affect profitability.
Safiya Noble (2018) goes even further in her book Algorithms of Oppression, pointing out that Google’s and YouTube’s ranking systems repeatedly amplify racist and sexist content – simply because it performs well. For example, she points out that when a user searches for “black girls,” the most likely thing to appear at the top of the search results is often a highly gendered, offensive stereotype. This is not because the algorithms are “intentionally” racist, but because this type of content attracts clicks. And in the eyes of the platforms, clicks equal money.
In 2020, the #StopHateForProfit campaign brought this issue into the spotlight. Over 1,000 major companies—including Coca-Cola, Adidas, and Unilever—paused their advertising on Facebook to protest the platform’s failure to curb hate speech and misinformation, particularly toward marginalized communities. Their message was clear: when platforms profit from hate, brands can’t stay silent (BBC News, 2020).
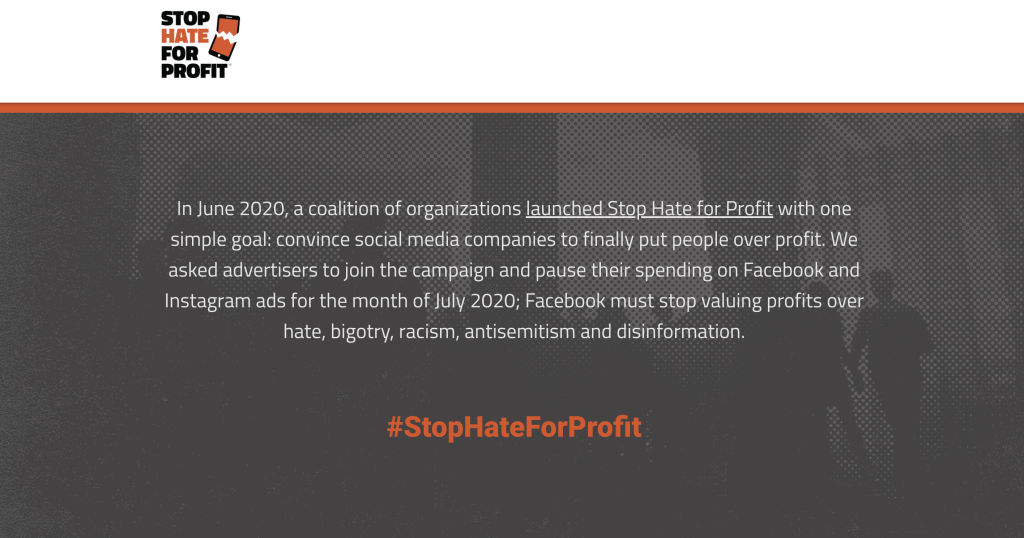
The harsh reality is that bias is not a “fault” of the program, it’s a “feature” itself – and a profitable one at that. When an algorithm is trained to “maximize user engagement no matter what,” it tends to push content that provokes the most reaction-no matter how much social harm it may cause. And since marginalized groups are often the easiest targets for cyberattacks, the algorithm’s operating logic unwittingly reinforces the social hierarchies we should be working to dismantle in the first place.
So the next time you see a sensationalized headline or a racially-charged fan image go viral, ask yourself: is this content being retweeted because it tells the truth, or because it keeps us from stopping our swiping fingers?
Two Real-World Cases of Algorithmic Harm
First Cases: Meta faces £1.8 billion lawsuit over allegations of inciting violence in Ethiopia
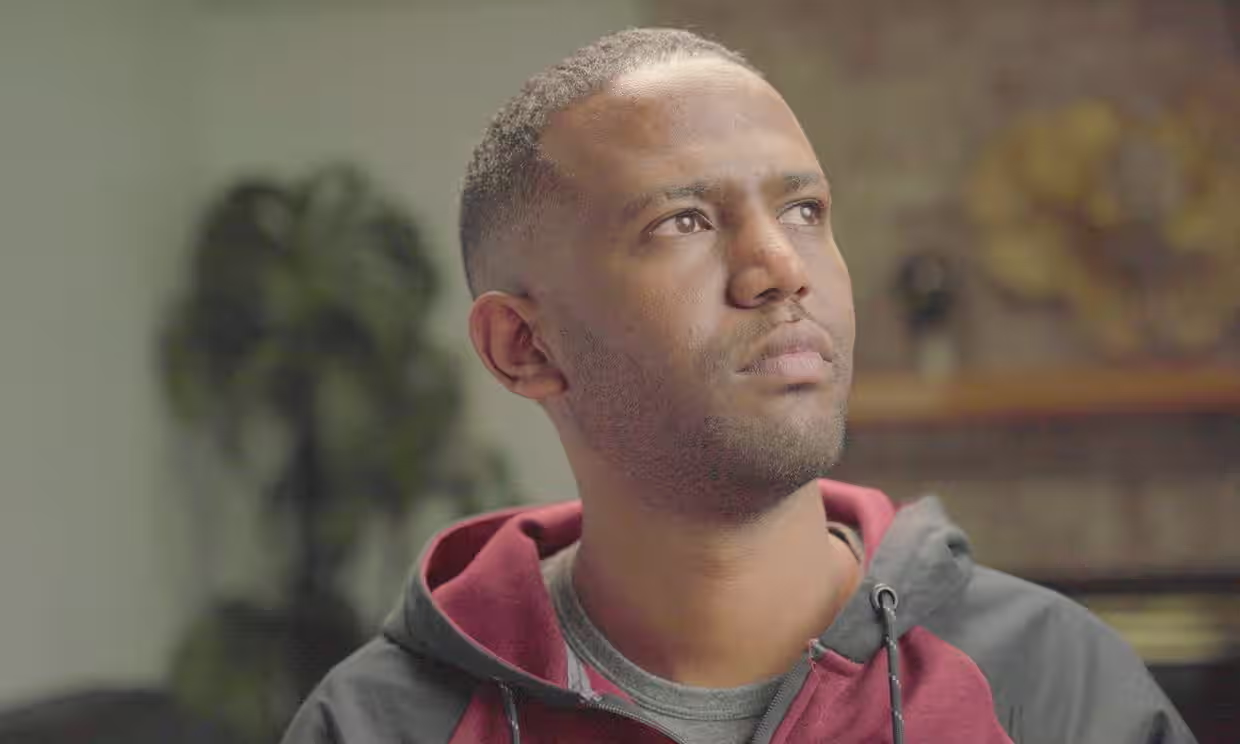
When we talk about algorithmic bias and racial injustice, Facebook’s case in Ethiopia is certainly a devastating real-life example. The platform’s algorithms have been accused of amplifying hate speech, which ultimately sparked real-world violence – and even led to death.
As civil war erupted in Ethiopia’s northern Tigray region between 2020 and 2022, and ethnic tensions escalated across the country, Facebook, one of the most widely used social platforms in the region, became a key channel for the dissemination of information – as well as a hotbed of disinformation and inflammatory content. A large number of hate-mongering posts were widely circulated on the platform, further exacerbating the conflict.
One of the most heartbreaking of these incidents occurred with Meareg Amare, a chemistry professor at Bahir Dar University.2021 In 2021, defamatory posts began circulating on Facebook against him, not only leaking his personal information but also falsely accusing him of supporting the Tigrayan People’s Liberation Front (TPLF). The posts spread quickly, and a few days later, Prof. Amare was shot dead by unidentified gunmen in front of his house.
His family repeatedly reported the threatening content to Facebook, but the platform failed to remove it in time. According to The Guardian, the warnings were not addressed until the tragedy.
What Caused It?—The Algorithm Behind the Violence
Facebook’s algorithm is designed to maximize user engagement and time spent on the platform. Unfortunately, this design tends to prioritize sensational, emotional, and inflammatory content—because it keeps people scrolling, clicking, and reacting. In Ethiopia, this translated to the widespread promotion of hate speech and misinformation, which escalated ethnic tensions offline.
Internal documents revealed that Facebook had known as early as 2019 that its platform was vulnerable to abuse in regions like Africa. Yet, the company allocated only 16% of its global content moderation budget to areas outside the United States, leaving much of the world under-protected from harmful content.
In April 2025, a high court in Kenya ruled that Meta, Facebook’s parent company, could be sued in Kenya for failing to act on content that allegedly fueled ethnic violence in Ethiopia. The plaintiffs are demanding structural changes to Meta’s algorithm, more content moderators for African regions, and a victim compensation fund for affected families.
The Facebook-Ethiopia case highlights the urgent need for social media platforms to take accountability for how their algorithms operate—especially in regions with political and ethnic volatility. Design choices shouldn’t be guided solely by engagement metrics, but by an ethical responsibility to prevent harm.
This case also raises broader questions about how tech giants operate in developing nations. Companies like Meta must pay closer attention to the sociopolitical environments they affect—and ensure their platforms aren’t being used as tools for hate and violence.
Second Cases: When Search Results Kill – The Dylann Roof Tragedy
In 2015, Dylann Roof, a 21-year-old white supremacist, carried out a mass shooting at the Emanuel African Methodist Episcopal Church in Charleston, South Carolina, killing nine Black worshippers. His radicalization didn’t start in person or through organized hate groups—it began online. According to his own manifesto, Roof’s racial “awakening” was triggered by Google searches like “Black on White crime.” What he found at the top of his search results were not government data or balanced journalism—but links to white supremacist propaganda sites like those run by the Council of Conservative Citizens (CCC)
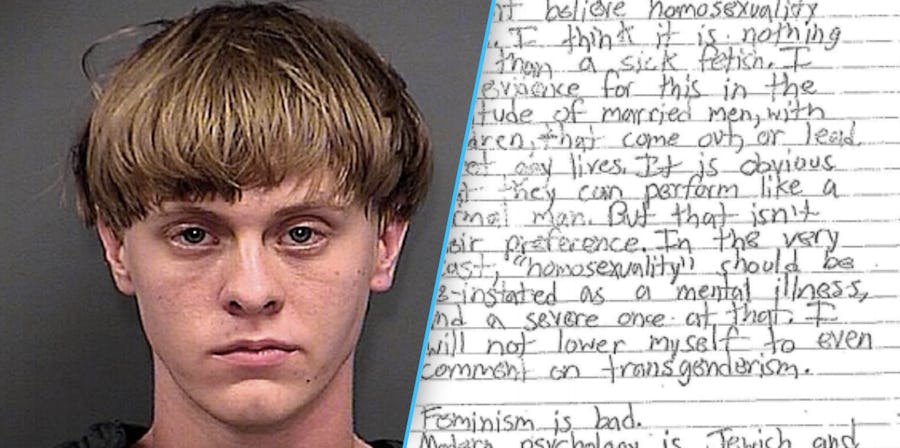
Because the algorithm didn’t direct him to counterpoints or anti-racist education. Instead, it funneled him deeper into a one-sided, extremist view of reality. As Safiya Noble (2018) points out in Algorithms of Oppression, Google’s search engine often prioritizes content not by accuracy or public value, but by popularity and ad-driven logic. This means extremist content—designed to provoke, mislead, or enrage—can rise to the top simply because it generates more clicks.
How does this relate to algorithmic bias?
This case is a clear example of what Kate Crawford (2021) calls “worldview encoding.” Algorithms don’t just reflect reality—they construct it. When an AI system elevates biased, dangerous, or one-sided content, it doesn’t need to “want” to be racist. It only needs to follow the logic it was trained on: prioritize what gets the most engagement. That logic, in a deeply unequal society, leads to unequal outcomes—sometimes tragically so.
Moreover, Noble argues that such search results reinforce a “white racial frame,” a conceptual worldview that centers whiteness and marginalizes non-white perspectives. Search engines, she warns, are not neutral—they are cultural products shaped by commercial interests and coded assumptions.
Dylann Roof’s path to violence wasn’t caused by an algorithm alone—but the algorithm played a silent, powerful role in reinforcing his views. This real-world case forces us to confront the dangers of algorithmic bias not just as a theoretical issue, but as a life-and-death matter.
If tech companies insist their systems are neutral while those very systems amplify hate, misinformation, and racism—they are complicit, even if unintentional. The stakes are too high for “glitches.” This is next-level bias, and it demands next-level accountability.
5. What Can We Do?
You know what? Changing algorithmic bias starts with each and every one of us. Many people think that social media platforms just push us the content we “love to read,” but that’s usually not true. Algorithms learn from our daily clicks and browsing habits, even if we don’t realize it ourselves, and Safiya Noble (2018) mentions that many personalized recommender systems, in fact, follow a biased data trajectory that quietly reinforces racial or gender stereotypes. While we can protect ourselves with gadgets like ad blockers or turning off personalized recommendations, it’s crucial to figure out how these systems work. Once you get it, you can fight back. Like when black TikTok creators stood up to the platform’s suppression of their content, and didn’t TikTok end up having to change the rules? (BBC News, 2020)
Then there are the platform companies themselves. They always say that “our system is neutral”, but that’s not a good argument, as Frank Pasquale makes particularly clear in The Black Box Society: algorithms are a reflection of the minds and business objectives of the people who designed them. The core logic of many platforms recommending content these days is that the longer you watch it, the more money they make. In order to achieve this goal, the platforms will favor content that stirs up emotions, and is even a bit controversial and discriminatory. Because this content is more eye-catching. But really, platforms could have chosen to do something else entirely. For example, hiring a more diverse design team, doing regular bias reviews, or letting users choose which recommendations they want – these are all practical ways to make the system fairer (AlgorithmWatch, 2024).
What about the government? It shouldn’t be deciding what we watch or think, but it does have a responsibility to set the rules of the game and hold platforms somewhat accountable. The European Union passed the Digital Services Act a while back, requiring big platforms to disclose their algorithmic logic, assess risks, and give users the option to turn off personalized push. I’ve heard that Australia and the U.S. are getting ready to follow suit. In fact, it’s not just about regulation, it’s about protecting our right to know. Independent watchdogs, funding for public interest technologies, public participation in monitoring – these are all important to ensure that the future is not just about “code written by companies”, but also about “values shared by society” (European Commission, 2009). “(European Commission, 2023; AlgorithmWatch, 2024).
I’d like to end with one final thought. Change doesn’t start with machines—it starts with us.
Reference
AlgorithmWatch. (2024). A year of challenging choices – 2024 in review. https://algorithmwatch.org/en/a-year-of-challenging-choices-2024-in-review/
AlgorithmWatch. (2024). New audits for the greatest benefits possible. https://algorithmwatch.org/en/new-audits-greatest-benefits-possible/
BBC News. (2020, June 30). Third of advertisers may boycott Facebook in hate speech revolt. https://www.bbc.com/news/technology-53230105
Buolamwini, J., & Gebru, T. (2018). Gender shades: Intersectional accuracy disparities in commercial gender classification. Proceedings of Machine Learning Research, 81, 1–15. https://proceedings.mlr.press/v81/buolamwini18a.html
Crawford, K. (2021). Atlas of AI: Power, politics, and the planetary costs of artificial intelligence. Yale University Press.
European Commission. (2023). The Digital Services Act: Ensuring a safe and accountable online environment. https://commission.europa.eu/strategy-and-policy/priorities-2019-2024/europe-fit-digital-age/digital-services-act_en
Noble, S. U. (2018). Algorithms of oppression: How search engines reinforce racism. NYU Press.
Pasquale, F. (2015). The black box society: The secret algorithms that control money and information. Harvard University Press.
Reuters. (2025, April 4). Meta can be sued in Kenya over posts related to Ethiopia violence, court rules. https://www.reuters.com/technology/meta-can-be-sued-kenya-over-posts-related-ethiopia-violence-court-rules-2025-04-04/
The Guardian. (2025, April 3). Meta faces £1.8bn lawsuit over claims it inflamed violence in Ethiopia. https://www.theguardian.com/technology/2025/apr/03/meta-faces-18bn-lawsuit-over-claims-it-inflamed-violence-in-ethiopia
The Washington Post. (2015, June 20). Dylann Roof’s racist manifesto: ‘I have no choice’. https://www.washingtonpost.com/national/health-science/authorities-investigate-whether-racist-manifesto-was-written-by-sc-gunman/2015/06/20/f0bd3052-1762-11e5-9ddc-e3353542100c_story.html
Be the first to comment