Have you ever searched something on Google, only to wonder why those particular results showed up first? Or maybe you noticed how TikTok seems to know what you’ll watch next, better than your best friend? That’s not magic. It’s algorithms. These digital tools are quietly shaping our lives, deciding what we see, read, and even believe. But unlike laws passed by governments, we don’t get to vote on these rules.
We often don’t see these systems working—but they are everywhere. From deciding what ads you see on Instagram, to how your credit score is calculated, to whether your job application is even reviewed by a human. Algorithms have become so embedded in everyday decisions that their influence can feel invisible. But invisible power is still power.
In this blog, we’ll look at how algorithms are becoming powerful tools of control in our digital lives. We’ll talk about how they can be biased, how they affect democracy, and what we can do to make them more fair and transparent.
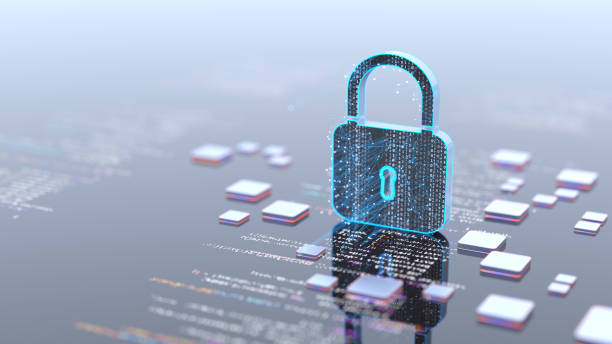
Image credit: iStock
Algorithms Are Not Neutral
Many people think algorithms are just math—fair and logical. But in reality, they are built by people, trained on data, and full of human choices. That means they can reflect the same biases found in society.
As Roberts (2019) argues, even the most advanced algorithmic systems often rely on hidden human labor—such as low-paid workers moderating content or labeling data behind the scenes. These invisible roles raise questions about fairness and exploitation, even when a system appears automated.
Safiya Noble, in her book Algorithms of Oppression, gives a powerful example. When someone searched “Black girls” on Google a few years ago, the top results were mostly harmful and sexualized content. These results didn’t show up by accident. They were created by algorithms trained on biased data, influenced by what people click on, and shaped by advertising (Noble, 2018).
Frank Pasquale, author of The Black Box Society, argues that these systems are not just biased—they’re secretive. Companies don’t explain how their algorithms work. This makes it hard to know why certain results appear or why some content is blocked (Pasquale, 2015).
In fact, bias can enter systems in surprising ways. In one case, Amazon built an internal hiring algorithm that was meant to filter through job applications automatically. But because it was trained on past resumes—most of which came from men—it learned to penalize resumes that included the word “women’s” (as in “women’s chess club captain”) and gave lower scores to graduates from all-women’s colleges.
Algorithms are not just organizing information. They are making decisions that affect real lives, and they often do so without transparency or accountability.
A New Kind of Governance
Governance usually means laws, rules, and policies made by governments. But today, tech companies are creating their own rules—through code.
As Just and Latzer (2017) explain, “governance by algorithms” refers to the way automated systems now shape how people access information, interact with media, and make decisions. These algorithms play a powerful role in determining what users see online, what products they are offered, and even what news they consume. They act like invisible institutions, influencing behavior without most people realizing it.
Crawford (2021) adds that this digital power doesn’t exist in isolation. AI systems rely on public infrastructure like energy, water, and data, and yet the benefits of these systems mainly go to private companies. This creates a situation where corporations have massive influence over public life—but without democratic accountability.
So we have a system where private companies are setting the rules for how we live online—but without the checks and balances we expect in a democracy.
As Suzor (2019) explains, digital platforms have taken on a quasi-governmental role—making decisions about what content is allowed, which users are prioritized, and how information flows. Yet these rules are developed and enforced by private companies without democratic input, transparency, or meaningful appeal.
Real-World Consequences
As Suzor (2019) puts it, platforms and automated systems are governed by secret rules—often developed by corporate actors without meaningful public input. When those rules are applied in areas like welfare or criminal justice, the consequences can be devastating.
Let’s look at a real example from Australia: the Robodebt scandal.
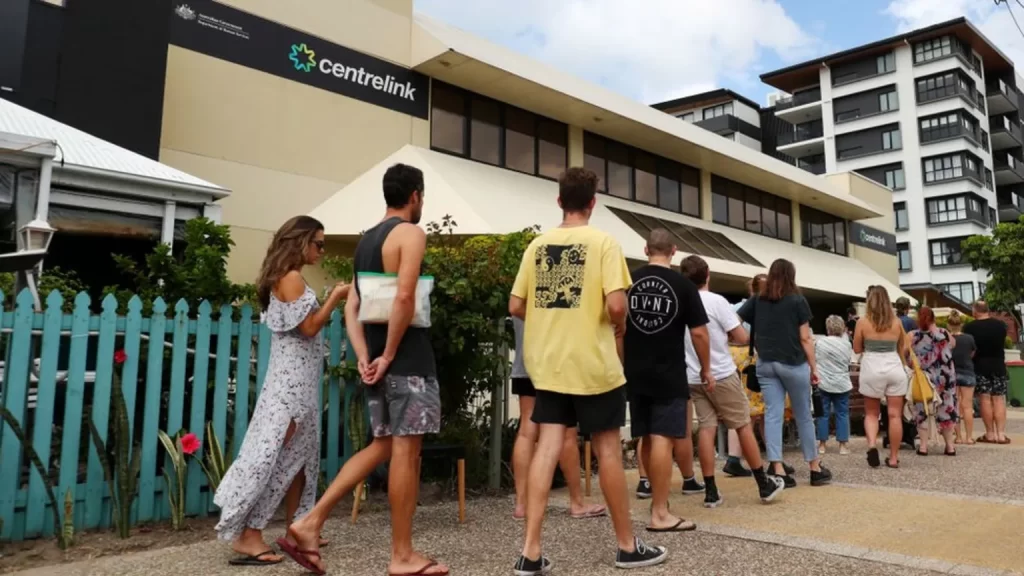
Image credit: Getty Images
Between 2015 and 2019, the Australian government used an automated system to detect overpayments in welfare. The idea was to find people who were receiving more money than they should. But the algorithm made mistakes. It sent debt notices to hundreds of thousands of people—many of whom didn’t owe anything.
This caused stress, financial hardship, and even contributed to mental health crises. Eventually, the program was ruled unlawful, and the government had to refund over $700 million (Mao, 2023).
The fallout from Robodebt led to a major public inquiry in Australia. The Royal Commission found that senior officials ignored warnings that the system was likely unlawful and caused harm. Public outrage grew as more stories emerged of people who suffered from false debt notices. The case became a turning point in discussions about automation in government, showing that even well-intended programs can go badly wrong if they ignore social context and ethical safeguards.
What went wrong? The system was automated, but it lacked human oversight. It treated people like data points, not individuals. And because it was run by a computer, many assumed it must be correct—even when it wasn’t.
This case shows the danger of using algorithms without transparency, fairness, or accountability. When machines make decisions about our lives, we need to ask: who is responsible when things go wrong?
Another high-profile example comes from the United States, where the COMPAS algorithm was used to predict recidivism risk. According to a ProPublica investigation, the system was more likely to falsely flag Black defendants as high-risk compared to white defendants, even when their prior records were similar (Angwin, Larson, Mattu, & Kirchner, 2016). This highlights how algorithmic decision-making can reproduce racial biases on a systemic scale.
International organizations have also raised concerns about algorithmic governance. For example, Amnesty International (2025) warns that the increasing use of algorithms and big data by governments can lead to discrimination, loss of privacy, and unfair decision-making. Without transparency and public oversight, such systems risk undermining fundamental human rights.
Another high-profile example comes from the United States, where the COMPAS algorithm was used to predict recidivism risk. According to a ProPublica investigation, the system was more likely to falsely flag Black defendants as high-risk compared to white defendants, even when their prior records were similar (Angwin, Larson, Mattu, & Kirchner, 2016). This highlights how algorithmic decision-making can reproduce racial biases on a systemic scale.
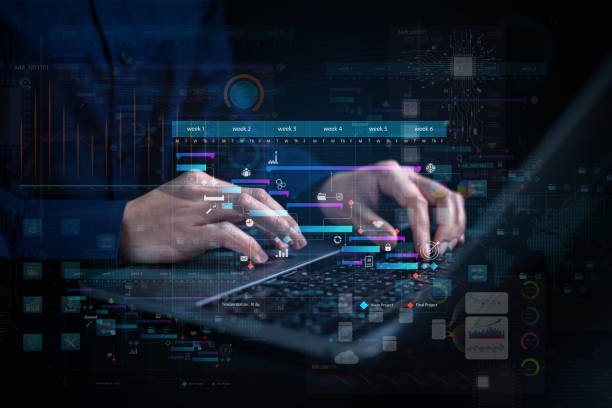
Image credit: iStock
Task-Specific Trust in Algorithms
Interestingly, not all algorithmic decision-making is rejected by the public. People are often willing to follow algorithmic advice, especially when the task is clear-cut or data-driven. According to Kaufmann, Chacon, Kausel, Herrera, and Reyes (2019), users are more likely to accept algorithmic suggestions in repetitive or objective tasks (like calculating routes or sorting data) than in subjective ones (like judging someone’s personality or job performance).
This finding is important. It shows that people can and do trust algorithms—but that trust depends on the situation. For example, using AI to recommend a movie is very different from using it to decide who gets a loan or welfare support. When algorithms are used in sensitive areas, people expect more transparency and human involvement.
This supports the idea that not all automation is bad—but it must be used wisely, and always with an awareness of its social impact.
This is why many people are fine letting Spotify recommend songs or Google Maps suggest a route—but feel deeply uncomfortable letting an algorithm decide whether they qualify for a mortgage or welfare. The stakes are different, and so is the level of acceptable risk.
What Can We Do?
As Karppinen (2017) explains, digital technologies like algorithms must be understood not just as tools for efficiency or innovation, but also as systems with profound implications for human rights. Their design and deployment shape access to information, freedom of expression, and democratic participation.
First, we need more transparency. Companies and governments should explain how their algorithms work, especially when they affect public life.
Second, we need stronger laws. Just like we have rules for clean water or safe cars, we should have rules for safe and fair algorithms. These rules could include regular audits, public reporting, and ethical guidelines (Pasquale, 2015).
Third, we need more public awareness. People should understand how algorithms shape their digital experiences. Education about algorithmic bias and data privacy should start early—in schools, not just in universities (Noble, 2018). Civic engagement matters. Ordinary people can push for better algorithmic systems by supporting regulation, asking questions, and demanding transparency from both governments and corporations. We’ve seen how public pressure led to change in the Robodebt case—similar activism can help shape how AI is governed in the future. Speaking up about fairness and accountability isn’t just for experts; it’s something everyone can be part of.
Fourth, we need to use algorithms in ways that match the task. As Kaufmann et al. (2019) explain, people are more likely to accept algorithmic advice when the context is appropriate. This means we need to be thoughtful about where and how we apply AI.
Finally, we need to include diverse voices in tech. Many of today’s systems were built without input from the communities they affect most. That needs to change (Crawford, 2021). As Noble and Whittaker (2020) argue, building a fairer digital future will require more than just better technology—it also demands policy reforms, worker organizing, and stronger public accountability structures.
Encouragingly, some governments are beginning to take action. In 2024, the European Commission passed the AI Act, a legal framework to regulate the use of artificial intelligence within the EU. The law includes risk classifications, transparency requirements, and rules to protect fundamental rights in high-risk systems (European Commission, 2024).
Final Thoughts
Imagine a world where algorithms are built with community input, are regularly audited, and are legally required to be explainable. In such a world, algorithmic systems could support—not replace—democratic values. They could help us make decisions better, not just faster. But to get there, we need pressure from below: from people who understand how this invisible system works and are ready to demand something better.
As Suzor (2019) warns, when platforms become the de facto rule-makers of the digital world, the lack of democratic oversight becomes a threat to justice and civic life. That’s why pushing for public interest technology and accountability isn’t optional—it’s essential.
Algorithms are not just tools. They are powerful systems that shape how we see the world—and how the world sees us. Right now, they often operate in the dark, guided by profit rather than fairness.
But it doesn’t have to be this way. If we bring transparency, accountability, and ethics into the design of digital systems, we can make algorithms work for everyone—not just the powerful few.
Let’s start asking better questions, demanding better systems, and remembering that behind every line of code is a human choice. And those choices should serve the public good.
References
Amnesty International. (2025, April 4). Algoritmes, Big Data en de overheid. https://www.amnesty.nl/wat-we-doen/tech-en-mensenrechten/algoritmes-big-data-overheid
Angwin, J., Larson, J., Mattu, S., & Kirchner, L. (2016, May 23). Machine bias: There’s software used across the country to predict future criminals. And it’s biased against blacks. ProPublica. https://www.propublica.org/article/machine-bias-risk-assessments-in-criminal-sentencing
Crawford, K. (2021). The Atlas of AI: Power, politics, and the planetary costs of artificial intelligence. Yale University Press. https://ebookcentral.proquest.com/lib/usyd/detail.action?docID=6478659
European Commission. (2024). AI Act. European Commission. https://digital-strategy.ec.europa.eu/en/policies/regulatory-framework-ai
Jonker, A., & Rogers, J. (2024, September 20). What is algorithmic bias? IBM. https://www.ibm.com/think/topics/algorithmic-bias
Just, N., & Latzer, M. (2017). Governance by algorithms: Reality construction by algorithmic selection on the Internet. Media, Culture & Society, 39(2), 238–258. https://doi.org/10.1177/0163443716643157
Karppinen, K. (2017). Human rights and the digital. In H. Tumber & S. Waisbord (Eds.), The Routledge Companion to Media and Human Rights (pp. 95–103). https://doi.org/10.4324/9781315619835
Kaufmann, E., Chacon, A., Kausel, E. E., Herrera, N., & Reyes, T. (2019). Task-specific algorithm advice acceptance: A review and directions for future research. Current Opinion in Psychology, 31, 110–115. https://doi.org/10.1016/j.copsyc.2019.09.007
Mao, F. (2023, July 7). Robodebt: Illegal Australian welfare hunt drove people to despair. BBC News. https://www.bbc.com/news/world-australia-66130105
Noble, S. U. (2018). Algorithms of oppression: How search engines reinforce racism. NYU Press. https://ebookcentral.proquest.com/lib/usyd/detail.action?docID=4834260
Noble, S. U., & Whittaker, M. (2020, June 23). Holding to account: Safiya Umoja Noble and Meredith Whittaker on building a more just tech future. Logic Magazine. https://logicmag.io/beacons/holding-to-account-safiya-umoja-noble-and-meredith-whittaker/
Pasquale, F. (2015). The Black box society: The secret algorithms that control money and information. Harvard University Press. https://www.jstor.org/stable/j.ctt13x0hch
Roberts, S. T. (2019). Behind the screen: Content moderation in the shadows of social media (pp. 33–72). Yale University Press. https://ebookcentral.proquest.com/lib/usyd/detail.action?docID=5783696
Suzor, N. P. (2019). Who Makes the Rules? In Lawless: The secret rules that govern our digital lives (pp. 10–24). Cambridge University Press. https://doi.org/10.1017/9781108666428
Be the first to comment