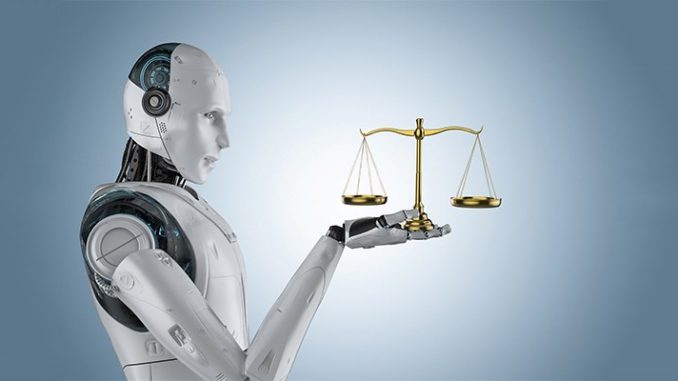
In the morning, you open your phone and the videos on Tiktok’s recommendation page perfectly match your interest; at noon, the algorithm of food delivery app plans the best route for the driver; at night, Alipay’s “Zhima Credit” scoring system silently evaluates your consumption behavior.
Have you ever wondered why the platform always recommends things that interest you? This is because the platform uses algorithms to calculate users’ interests and then recommends content based on each person’s interests.
Here is a question, what are algorithms? Algorithms are the processes and regulations created for machine driven tasks including data processing, computation, and automated decision-making. The method of assigning importance to information pieces in data collection by an automated, statistical review of privately produced data signals is known as algorithmic selection.
Nowadays, most people agree that algorithms on the internet are becoming increasingly important to society. Automated algorithmic selections are rapidly influencing a wide range of everyone’s activities in general and media consumption in particular. These technologies and techniques like algorithms and artificial intelligence now impact many social institutions, influencing their values and decisions making processes (Kate Crawford, 2021, p.8).
Famous situations include using search engines and news networks to choose online news or recommendation systems to consume music and video entertainment. Algorithms may seem neutral and rational, but actually, are they really not biased?
Gender trap under the illusion of ‘neutrality’
The development of algorithms, the use of data, and AI-generated decision making are all areas where gender bias in algorithms may show itself. The type of data entered can have a direct impact on the algorithm’s future decision-making.
Thus, if the data has bias at first, the algorithms may reproduce them, which, if employed for extended periods of time, may perpetuate the biases in the decision making process. Many researches show that women and girls are frequently seen as less technologically adept and less active than males, while technology is frequently associated with ‘men’s power’.
The gender gap in women’s engagement in related sectors may be increased by such misunderstandings. On October 21, 2013, the United Nations began an advertisement using “genuine Google searches,” led by the advertising firm Memac Ogilvy and Mather Dubai, to call attention to the unfair and unequal ways in which women are treated and rejected human rights (Noble, Safiya U, 2018, p.15).
The autosuggestions, which reflected the most common Google Search questions, came from different women. A lot of racist ideas have been added in the Google Search autosuggestions, including
·Women should not vote, work
·Women should stay at home, be in the kitchen
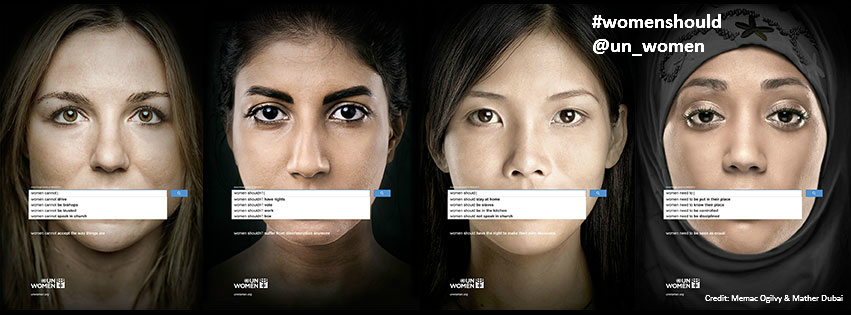
Maybe by accident, the campaign highlighted the huge influence of search engine results while using Google Search results to make a larger argument about the current level of public emotions about women. Users’ opinions and the fact that society still has a number of biases about women are reflected in search. Gender bias is the first choice when bias is established in the programming.
Not only the search engine, but also the advertisement of goods. Women are constantly linked to more negative and less beneficial behaviors than men, because of the gender bias in society.
Since computer algorithms now make a large number of marketing choices for business, including those relating to product suggestions, advertisement targeting, and the creation of new products, biases that computers pick up from human language may unavoidably bring bias back into the marketplace.
When product offerings are shown to consumers in a gender-biased way, algorithmic biases in advertisements are reflected like men and women feel gender bias through limited choices. For example, women are more likely to receive advertisements targeting ‘irresponsible investors’ than those targeting ‘disciplined investors’(Shelly Rathee, Sachin Banker, Arul Mishra, Himanshu Mishra, 2023).
Although there are biases in search engines and advertising, this has no substantial impact on women. What really makes me angry is the discrimination against women in recruitment.
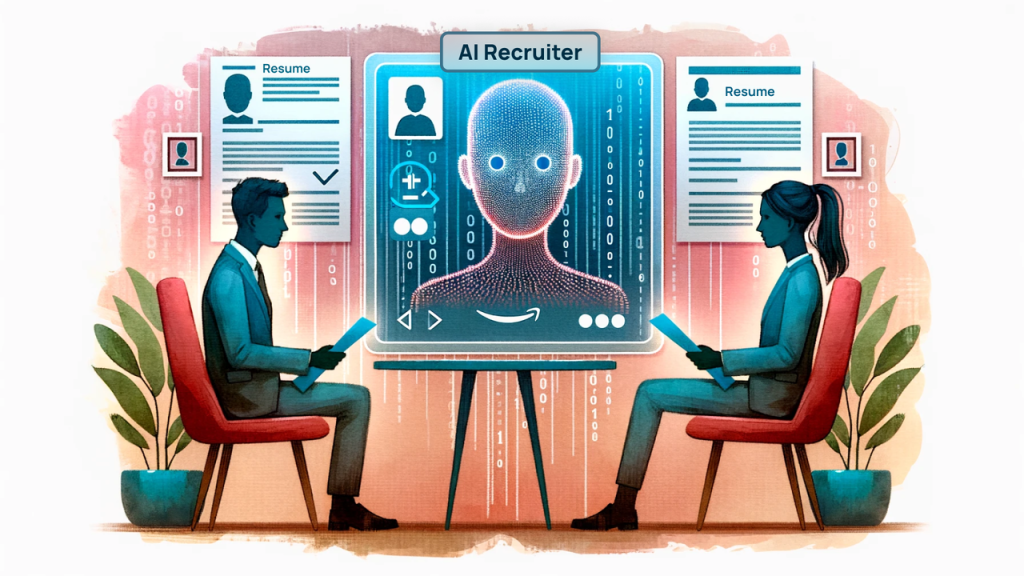
Here is an example, Amazon’s recruiting tool showed bias against women. Since 2014, Amazon’s team has been developing computer program to evaluate resumes in an effort to automate the process of finding the best employees.
Similar to how customers evaluate things on Amazon, the company’s experimental hiring tool employed artificial intelligence to provide job seekers scores between one and five stars.
However, one year later, the team found that men who applied were preferred, according to what Amazon’s algorithm has learned. Resumes that used the phrase ‘women’s’, such as ‘women’s chess club captain’, were rejected (Rachel Goodman, 2018). Additionally, it devalued graduates from two universities exclusively for women. In this case, outstanding female job seekers may lose interview opportunities.
Algorithms can be not only sexist, but also racist. Have you ever thought that facial recognition, which we often use, can also be racially discriminatory?
A white person could think that facial recognition software is unimportant. Digital gates at residential structures, schools, firms, and airports are just a few of the regular activities that use this technology, which has built into many technologies, including our phones.
The digital face of racism
However, because their faces are not even identified or because their facial features might be mistaken for those of other people, the experience may be used as an active reminder of the biases present in our societal system for black people or people of other minority groups.
This issue is largely caused by the lack of variety in the photos that are used to supply data that teach artificial intelligence based devices. Due to the limited range of types like skin color, hairstyle and eye color, this kind of technology is far more likely to be mistaken. For example, the faces of two black people is easier to be mistaken than two white people.
These kind of cases come below the category of ‘algorithmic racism’(EloInsights, 2022). in digital settings, this phenomenon refers to the way our unconscious biases appear in algorithms that control how devices work and finally maintain inequity.
Racial bias are also happened on Google Search engine. When searching Black people, Google’s autosuggest results are ‘why are black people so lazy’, ‘why are black people so rude’. But when searching White people, Google’s autosuggest results are ‘why are white people so pretty’, ‘why are white women so perfect’(Noble, Safiya U, 2018). We can see that it is true that Google Search engine is racial bias.
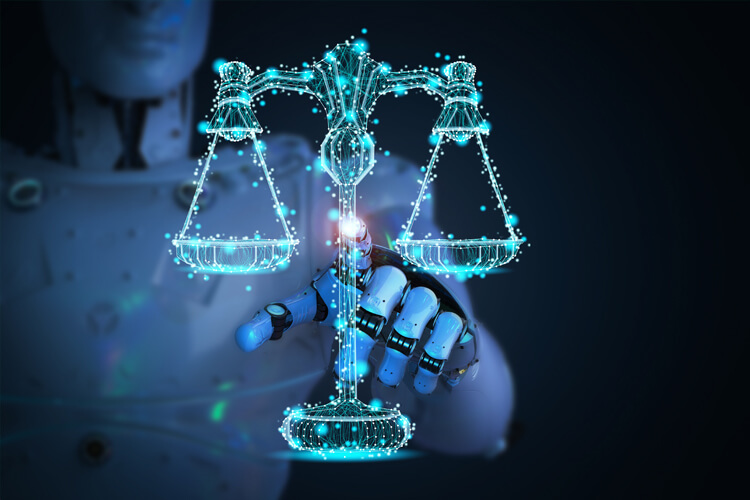
Here is an example of algorithm racial bias in America. COMPAS is an algorithm widely used in the United States to guide sentencing by predicting the likelihood that an offender will re-offend. Propublic began to evaluate Northpointe’s COMPAS in order to check the recrimination algorithm’s basic certainty and to identify whether it was biased against particular social groups.
According to the COMPAS research, white defendants were more likely than black defendants to be mistakenly marked as low risk, while black defendants were much likely than white defendants to be mistakenly evaluated to be at a higher risk of criminal behavior.
They examined over 10000 criminal defendants in Broward County, Florida, and compared their expected and actual recidivism rates over a two-year period. Most defendants submit up a COMPAS questionnaires when they are arrested, and the COMPAS software uses their responses to produce a number of scores, like estimations of ‘Risk of Violent Recidivism’ and ‘Risk off Recidivism’.
When the real recidivism rates of those charged in the two years following their scoring were compared to the recidivism risk groups expected by the COMPAS tool, they discovered that while the score accurately predicted an offender’s recidivism 61% of the time, it only accurately predicted violent recidivism 20% of the time.
The system made quite different mistakes when predicting who would re-offend, but it correctly identified recidivism for black and white offenders at about the same proportion (59% of white defendants and 63% for black defendants).
When analyzed during a two-year period, it incorrectly sorts the white and black criminals in different ways. Black offenders were frequently anticipated to have a greater possibility of return than they really did, according to their data. Unlike to their white counterparts, black prisoners who did not re-offend during a two-year period were almost twice as likely to be incorrectly identified as higher risk (45% and 23%), according to their findings.
It was frequently assumed that white offenders would be less dangerous than they actually were. According to the data, white defendants who committed new crimes over the next two years were incorrectly classified as ow risk nearly twice as frequently as black re-offenders (48% and 28%) (Jeff Larson, Surya Mattu, Lauren Kirchner and Julia Angwin, 2016).
This examples shows the algorithm which often be considered the fairest existence has racial bias.
From technological fixes to digital human rights
Gender and racial bias in algorithms is a very important issue. How to solve or optimize algorithms is the direction that governments and companies are working towards.
For platforms and companies that develop algorithms, they need to collect diverse data. A company called IBM has developed a fairness toolkit called AI Fairness 360.
AI Fairness 360 is an open-sources toolkit that includes modern algorithms to reduce bias and a wide range of measures to check for unexpected bias in data and machine learning models. It is used and contributed to both platforms and algorithm development business to help build confidence in AI and create a more equal society for all.
Nine distinct algorithms were created by the large algorithmic fairness research group to reduce that bias in this first version of the AI Fairness 360 Python module (Kush Varshney, 2018). Solving algorithmic unfairness through diverse date is a more effective method.
Not only companies can reduce algorithmic bias by adding more data, but governments can also formulate laws and regulations to govern artificial intelligence.
EU passed a act called AI Act in 2024. The AI Act is the world’s first complete law around AI. The regulations were created to support reliable AI all over Europe.
For specific uses of AI, the AI Act gives forth an exact list of risk-based regulations for AI creators and developers. The AI Act is a component of a large set of laws that focus on promoting the creation of correct AI, which also includes the Coordinated Plan on AI, the AI Innovation Package, and the development of AI Factories.
When put together, these policies ensure humane AI, safety, and basic rights while supporting use of AI, investment, and innovation in the Europe (European Commission, 2025).
This act lists AI used job recruitment and judicial administration as high-risk AI. This act requires that high-risk AI needs to collect higher-quality data sets as much as possible before it is put on the market to minimize the risk of discrimination.
Conclusion
Algorithmic bias is a digital reflection of the natural bias in human society rather than an error in technology.
Whether it is Google searches suggesting that women should be confined to the home, or the high-risk incorrect judgement of black defendants by the COMPAS system, it exposes the fact that algorithms automate and scale inequality by ‘learning’ biased historical data.
Women, ethnic minorities, and other groups have experienced double unfairness as a result of this bias, which has created a ‘digital discrimination chain’ in important domains like employment and justice improve system fairness through varied data collection (like AI Fairness 360 tools) and algorithm information on a technical level.
On an institutional level, create laws to limit high-risk AI applications (like the EU AI Act) and define developer responsibilities.
However, a deeper change in thinking is required since algorithm fairness is a social justice issue as well as a technological one. Algorithmic justice needs to go beyond technology governance in the future and look for solutions in diverse social evaluation, public engagement, and the development of international digital human rights standards.
Reference
Crawford, Kate (2021) The Atlas of AI: Power, Politics, and the Planetary Costs of Artificial Intelligence. New Haven, CT: Yale University Press, p.8.
EloInsights. (2022, November 16). What is algorithmic racism and how to overcome it. https://elogroup.com/en/insights/how-to-overcome-algorithmic-racism/
European Commission. (2024). AI Act. European Commission. https://digital-strategy.ec.europa.eu/en/policies/regulatory-framework-ai
Goodman, R. (2018, October 12). Why Amazon’s Automated Hiring Tool Discriminated against Women | News & Commentary. American Civil Liberties Union. https://www.aclu.org/news/womens-rights/why-amazons-automated-hiring-tool-discriminated-against
Larson, J., Mattu, S., Kirchner, L., & Angwin, J. (2016, May 23). How We Analyzed the COMPAS Recidivism Algorithm. ProPublica. https://www.propublica.org/article/how-we-analyzed-the-compas-recidivism-algorithm
Noble, Safiya U. (2018) A society, searching. In Algorithms of Oppression: How search engines reinforce racism. New York: New York University. p.15,21 .
Rathee, S., Banker, S., Mishra, A., & Mishra, H. (2023). Algorithms propagate gender bias in the marketplace—with consumers’ cooperation. Journal of Consumer Psychology, 33(4).https://doi.org/10.1002/jcpy.1351
Varshney, Kush. (2018). Introducing AI Fairness 360. IBM Research. IBM. September 19, 2018.https://research.ibm.com/blog/ai-fairness-360.
Be the first to comment