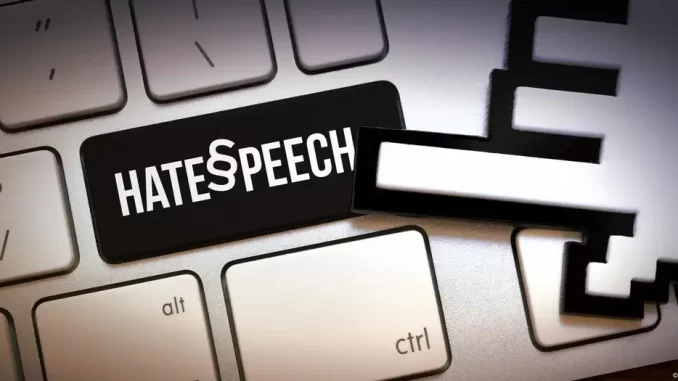
Recently, far-right online trolls and extremists seemed to become bolder after the US election. Following Donald Trump’s reelection, a notable increase in hate speeches and derogatory attacks aimed at women has been observed on social media platforms. These attacks often manifest in phrases such as “your body, my choice” and the derogatory “get back to the kitchen”, which seriously insult women’s rights.
The trigger for this surge in hate speech can be traced back to the evening of November 6, 2024, when White nationalist and Holocaust denier Nick Fuentes wrote on the X: “Your Body, My Choice, Forever” after Trump announced his victory. This statement is obviously a provocation to the slogan supporting women’s abortion rights – “My Body, My Choice”. At present, the post has more than 120 million views. Until now, remarks such as “Your body, my choice” and “We own your body now” continue to pour into the comment areas of female users on different platforms.
These viral slogans have even spread offline, becoming buzzwords among underage boys on campus and jokes used to intimidate female classmates in the corridors. At present, quite a few young girls and their parents have already shared examples of offline harassment on social media. On certain occasions, individuals on platform X and TikTok reacted to posts containing the phrase “your body, my choice” by issuing ambiguous threats of reprisal violence. Although the TikTok spokesperson made it clear that these hateful remarks violated the community guidelines and would be dealt with in a targeted manner, X did not respond.
Surge in Online Hate Speech: A Growing Global Reality
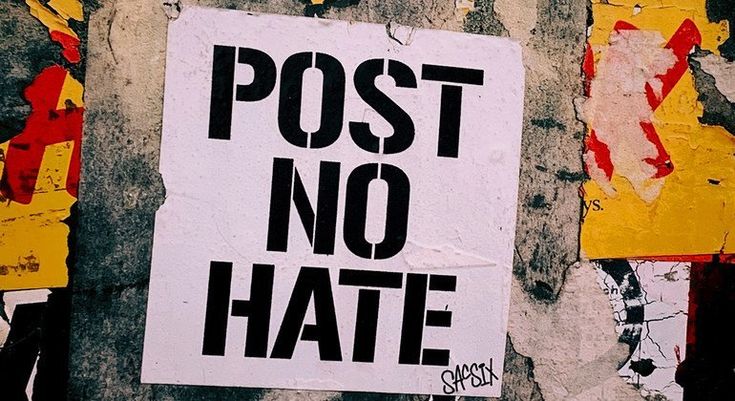
In recent years, far-right forces have been rising in Western politics, some of which support white supremacy and promote racial discrimination. Even more tragically, the contemporary media and social technology ecosystem has facilitated the rapid spread of alternative forms of knowledge production and identity propaganda, which not only makes the abortion debate appear more asymmetrical than it actually is, but also amplifies hate speech related to the abortion debate around the world. This further confirms that the rise of online hate speech, along with its amplification via digital platforms and social media, has emerged as a major and increasingly concerning issue (Flew, 2021).
Hate speech on the Internet is wrapped in a massive, anonymous, cross-border ocean of Internet content, and it presents multiple languages and forms, and is combined with emoticons, images, audio and video, etc. The content is becoming increasingly obscure, abstract, and symbolic, making it extremely difficult for computers to quickly identify it. To avoid being blocked by social media platforms, users often use misspelled or altered words to express hate online. This makes it harder to detect, collect, and label hate speech, as it is often incomplete and defined differently by different people (Mansur, Omar, & Tiun, 2023).
Besides, the transnational nature of the Internet makes it easier for individuals and organizations to escape and evade government regulation, so a large number of hate websites have emerged around the world in just a few years. Due to the instability of global politics in recent years, the rise of populism, and the outbreak of various social movements, global online hatred has ushered in a new surge.
In this context, the state-led governance model has failed, and social media platforms have gradually become the main actors in regulating online hate speech. This has moved the focus of regulation from legal legislation to corporate policies, platform responsibilities and technical ethics. However, as most of the major platforms are in the US, their relaxed speech culture and “corporate self-defined rules” have led to the governance of hate speech falling into a gray area, with vague standards and the right of interpretation in the hands of technology companies.
Practical Strategies of Platform: Taking X as an Example
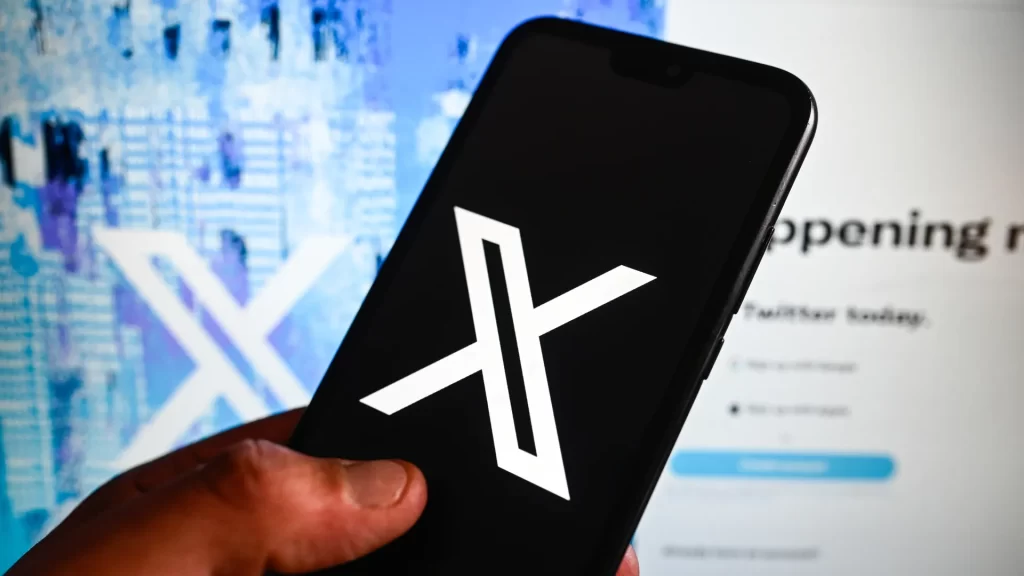
X has always avoided defining “hate speech” and created the concept of “hateful conduct” as a regulation in the user agreement. Its definition of “hateful conduct” is: “Users are not allowed to directly attack others based on their race, ethnicity, nationality, caste, sexual orientation, gender, gender identity, religious affiliation, age, disability or serious illness”.
Using the concept of hateful conduct, X argued that what they banned was not “speech” but “behavior”. Therefore, offensive content is still allowed to be posted, and hate speech only constitutes “hateful behavior” if it is directed at a specific user. For example, some scholars believe that its guiding principles and definitions are vague and too broad, and users have long complained that the rules are inconsistent and ineffective, and fail to protect relevant groups from harassment.
X’s user speech regulation is mainly based on voluntary user reporting and public labeling. Its system screening mainly relies on manual work, and introduces an AI automatic recognition system for harmful content based on images. This system will prompt users that the content may be harmful, but it is up to the user to choose whether to continue watching the content. Meanwhile, users can also mark whether the content is harmful. However, X often just bans the account after receiving a report. This neutral attitude has obviously accelerated the loss of X users, especially celebrities and opinion leaders who are most severely affected by verbal violence, and directly led to the shrinkage of X’s content ecosystem.
Imperfect AI: “Necessary”, “Precise” & “Fragile”
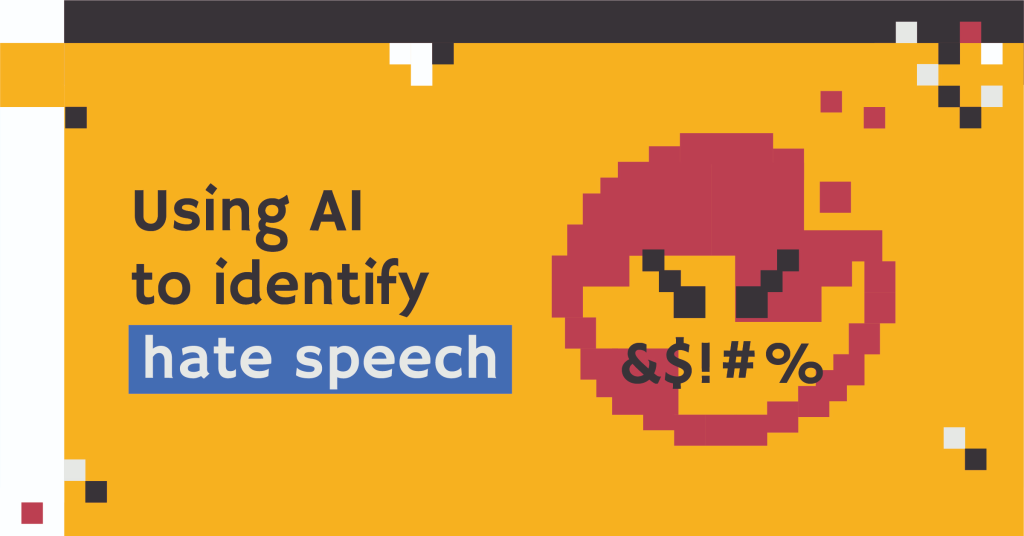
In addition to the trouble of formulating an overall hate speech strategy, Internet companies also face the tedious work of identifying, filtering, and cleaning hate speech every day. Therefore, artificial intelligence technology based on Automated Content Recognition has long been introduced into the regulation of hate speech.
AI is not an effective way to detect hate speech because the system lacks the emotions and judgment skills that humans possess. Hate speech is deeply shaped by specific contexts, making it essential to rely on comprehensive understanding in order to effectively identify and respond to it (Sinpeng, Martin, Gelber, & Shields, 2021). All of these models encountered significant difficulties and a notable drop in accuracy when it came to capturing the specific targets of hate speech or analyzing its nuanced characteristics (Kumarage, Bhattacharjee, & Garland, 2024). Although AI is not perfect yet, it is improving rapidly. Companies continue to emphasize the importance and necessity of applying AI to hate speech identification. For example, Facebook has been working hard to claim that its intelligent recognition technology has been rapidly improving and that it will proactively delete most hate speech before users report it.
However, the actual data is not so stable and optimistic. Data from Facebook in 2018 showed that the AI system was able to delete 99% of terrorist propaganda and 96% of nude content, but only 52% of hate speech. Corresponding to the low recognition efficiency, there are also misidentifications. Facebook’s algorithm once made a mistake and mistakenly deleted the Declaration of Independence as hate speech. It even labeled a video of a black person as a “primate”, sparking public outrage.
The vulnerability of AI also lies in the fact that it can only respond to known patterns and has difficulty identifying emerging expressions of hate, especially in multimodal content and multilingual environments. To compensate for this limitation, most platforms still rely heavily on manual review and even need to recruit specialized reviewers for specific countries. Therefore, the role of AI in platform governance presents a dilemma: it is shaped as a key solution, but it also constantly exposes its structural fragility and governance boundaries.
Invisible Algorithm “Black Box”: Original Sin, Bias & Countermeasures
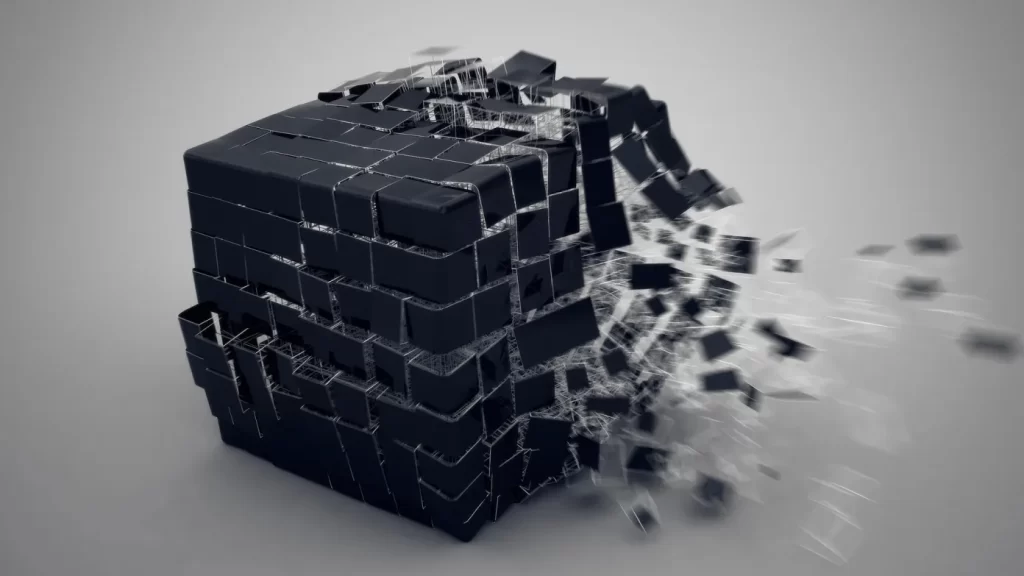
In computer science, algorithms are also likened to “black boxes”. It means that the process from data input to algorithmic judgment output is like passing through an opaque, unknown black box. We cannot observe and supervise the entire algorithmic processing process like we can observe, understand, trace back, correct, and supervise legal procedures. People have no way of knowing the goals, intentions, and rules of the algorithm, nor the background and content of the algorithm design. This information asymmetry also makes algorithmic logic criticized as a terrible, invisible power.
In the increasingly prominent reality of the eruption of global online hatred, the Internet and algorithms are often imagined as a kind of “original sin” that tolerates, parasitizes, and even produces and promotes the division and hatred of human society. The Internet gives individuals the ability to speak horrific words, because it is easier for people to express hatred when they do not have to deal with the consequences of their words. In addition, algorithms provide conditions for hatred to be continuously enhanced and reproduced. Algorithms continue to produce information cocoon effects through machine learning, making the polarization of opinions increasingly prominent.
The Internet is playing the role of a “hate amplifier”. Algorithmic bias may boost user engagement by triggering fear or anger, which can unintentionally promote extremist ideas and expose users to radical content. Extremist groups like IS and al-Qaeda have used social media to spread propaganda and recruit members. IS uses platforms like X and Telegram to build a sense of community and share emotional content that encourages radical views. Al-Qaeda shares speeches and training videos on YouTube, often with encrypted links. On the far-right side, TikTok has been used to promote extremist content, as its “For You” page can lead users into algorithmic rabbit holes that amplify extremist ideas. Without clear and comprehensive definitions of extremism, radicalisation, and hate speech, malicious actors will keep exploiting weaknesses in content moderation (Govers, Feldman, Dant, & Patros, 2023).
Algorithms are created by humans. There is no neutral algorithm and algorithms themselves mean discrimination and prejudice. The political prejudices of race, gender, and minority groups that exist in reality have almost been proven to exist in algorithmic identification. In 2019, researchers at Facebook found that if the activity track of Instagram users is identified as black by the algorithm, their accounts are 50% more likely to be automatically disabled by the review system than those identified as white users. A study by Cornell University found through analysis of X data sets that tweets judged by the algorithm to be written by African Americans are more likely to be marked as hate speech.
In addition, the algorithm is constantly updated based on machine learning of massive texts, but these data themselves contain social stereotypes. For example, Facebook allows gender discrimination in job ads: ads for machinist positions are mainly for men, while job ads for kindergarten teacher positions are mainly for women. So, what exactly is the output of the algorithm black box? Is it a hidden script of a pre-existing human society, or a “beautiful new world” that has been purified and processed?
Summary and Outlook
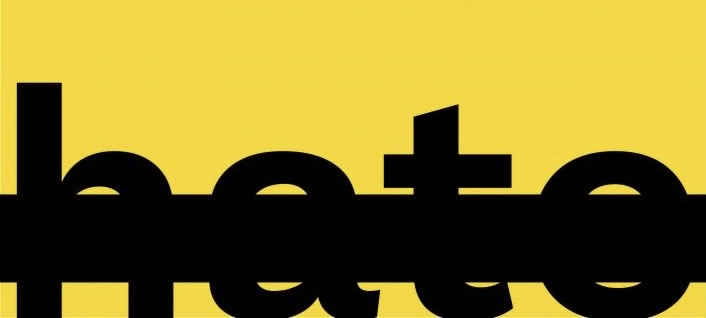
Driven by the Internet, “hate speech” has broken through the original boundaries of expression and quickly become a global problem. Faced with the spread of online hate speech, technology companies have gradually replaced the state and become the main governance subject, but they have also frequently had friction with the government, advertisers and the public in the process of regulating hate speech. The lack of industry consensus and vague regulatory standards have put large platforms under greater pressure and forced them to find a balance between risk avoidance, corporate responsibility and technology application.
In governance practice, technology companies need to accurately define “hate speech” to adapt to the governance needs of different countries and cultural backgrounds. Besides, artificial intelligence and algorithms are regarded as the core means of identifying and controlling hatred. However, these technologies often cause controversy due to bias, opacity and misjudgment, and may instead promote the spread of hatred and even push the platform into the role of a new “political entity”.
Platforms, governments and the public need to form a collaborative governance. As platforms should establish transparent speech recognition standards, strengthen localized content review capabilities, promote industry collaboration, use algorithms with caution, and balance efficiency and responsibility. Governments should build a flexible regulatory mechanism, form a cooperative governance relationship with the platform, while maintaining the dominant position of public power and promoting the simultaneous evolution of legislation and technical specifications. The public needs to improve digital literacy, express rationally, actively report, and participate in building an inclusive public opinion space. Only through tripartite collaboration can we effectively respond to the cultural conflicts and governance challenges caused by online hate speech and rebuild a safer and more rational digital public space.
Ultimately, this has led to thinking about deeper issues: How is hatred produced, continued and amplified? To what extent do technology and algorithms affect human emotional expression and historical memory? In the context of digital governance, the intersection of technology and humanities should not be just a technical repair, but should become an entry point for rethinking social order and the future of mankind.
References
Flew, T. (2021). Hate speech and online abuse. In Regulating platforms (pp. 91-96; pp. 115-118). Polity.
Sinpeng, A., Martin, F., Gelber, K., & Shields, K. (2021, July 5). Facebook: Regulating hate speech in the Asia Pacific. Final Report to Facebook under the auspices of its Content Policy Research on Social Media Platforms Award. Department of Media and Communication, University of Sydney, and School of Political Science and International Studies, University of Queensland.
Mansur, Z., Omar, N., & Tiun, S. (2023). Twitter hate speech detection: A systematic review of methods, taxonomy analysis, challenges, and opportunities. IEEE Access, 11, 16226-16249.
Govers, J., Feldman, P., Dant, A., & Patros, P. (2023). Down the rabbit hole: Detecting online extremism, radicalisation, and politicised hate speech. ACM Computing Surveys, 55(14s), 1-35.
Kumarage, T., Bhattacharjee, A., & Garland, J. (2024). Harnessing artificial intelligence to combat online hate: Exploring the challenges and opportunities of large language models in hate speech detection. arXiv preprint arXiv:2403.08035.
X (2023). X’s policy on hateful conduct | X Help. [online] help.x.com. Available at: https://help.x.com/en/rules-and-policies/hateful-conduct-policy.
CCDH (2023). Twitter fails to act on 99% of Twitter Blue accounts tweeting hate. [online] Center for Countering Digital Hate | CCDH. Available at: https://counterhate.com/research/twitter-fails-to-act-on-twitter-blue-accounts-tweeting-hate/.
Duffy, C. (2024). ‘Your body, my choice’: Attacks on women surge on social media following election. [online] CNN. Available at: https://edition.cnn.com/2024/11/11/business/your-body-my-choice-movement-election/index.html.
Meta (2025). Log in or sign up to view. [online] transparency.meta.com. Available at: https://transparency.meta.com/reports/community-standards-enforcement/hate-speech/facebook/.
Awasthi, S. (2025). From clicks to chaos: How social media algorithms amplify extremism. [online] orfonline.org. Available at: https://www.orfonline.org/expert-speak/from-clicks-to-chaos-how-social-media-algorithms-amplify-extremism.
Be the first to comment