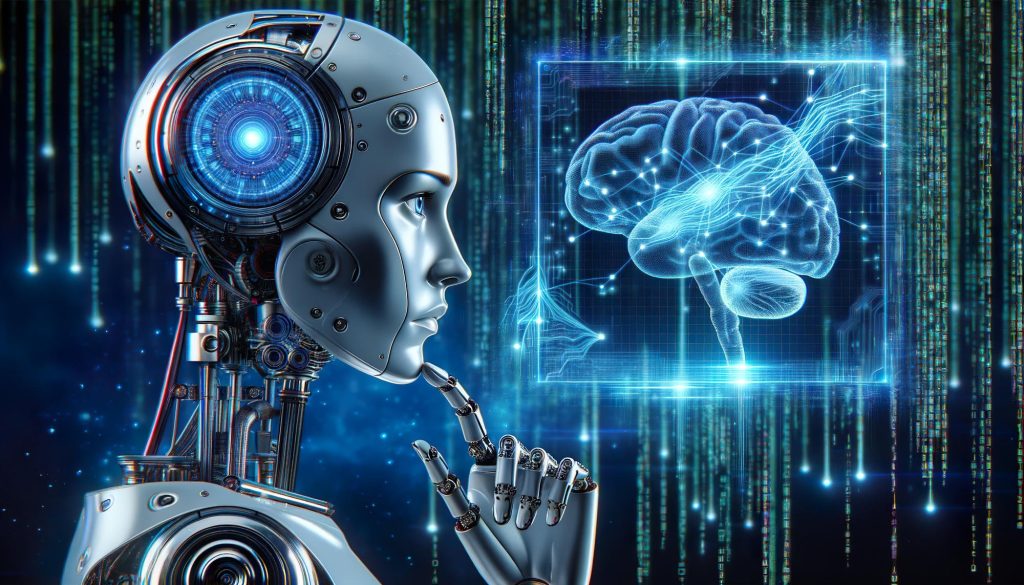
Introduction: When algorithms start to be biased
Have you ever swiped through a video platform and found that the content recommended to you has become more and more “one-dimensional”? Or even when you submit your resume, you don’t receive a response after a long time? Many people think that these phenomena are just a coincidence of data or a problem of their own ability. But in fact, behind them all lies a bigger problem, that is, whether the algorithm is really fair.
In an era where artificial intelligence rules recommendation, screening and evaluation, we rely more and more on those invisible and intangible “intelligent” systems to make decisions for us. But who trained these AIs? Humans. It is our past choices that inform big data. What affects the final outcome are our invisible “biases”. When these biases are silently written into the program, can the machine now be neutral?
In this blog, I’d like to take you on a deep dive into an issue that many people overlook, but which has a huge impact: algorithmic bias and AI discrimination. We’ll look behind real-world examples, academic studies and seemingly “objective” systems to discover that AI is not always the “rational machine” we think it is. And more importantly – what can we do about it?
In this blog, we’ll talk about it with this part:
What is algorithmic bias and why does AI discriminate against people?
Some real-world examples
Why algorithms are not neutral and whose power and values are hiding behind them?
And finally, what can we do about algorithmic injustice and what can we do about it?
What is “algorithmic bias” and why does AI discriminate?
Machines and AI are seen as rational and fair, but the truth is that they are far less “neutral” than we think. One survey found that when people searched for the term “black girl” in a search engine, the results were pages filled with pornography (Noble, 2018). When researchers helped children search for information about themselves and themselves, black girls were still fodder for erotic web sites, being into commodities, products, and sexually fulfilling objects (Noble, 2018). This is undoubtedly blatant racism and sexism. So, who is contributing to this subtle discrimination? Is it the algorithms themselves? And where does machine bias come from?
In fact, algorithmic bias is not so much about “broken machines” as it is about “viruses” in the data that machines use. Most AI systems, especially those on machine learning, are trained on large datasets collected from the real world. As scholars have said, “Algorithms are not actively racist or sexist, they simply inherit socially structured biases that are reflected in the data we provide” (Johnson, 2020).
Discrimination between AIs still exists in key initiatives. This may have more serious consequences. For example, if a recruitment system uses data from a company’s employees over the past decade, in which male executives account for the majority, AI may conclude that “men are more suitable for executive positions”, which is completely wrong. This is not its “intention”, but the “reality” it has learned. If this system continues to operate, gender inequality in the workplace will be aggravated, and women will lose their job opportunities, benefits, and social rights.
What’s even scarier is that it’s hard to see how these biases are formed. Many AI algorithms are so-called “black box” systems, such as the popular openAI, ChatGPT, etc (Kosinski, 2025). These AI models are trained on massive datasets through a complex deep learning process, and even their creators don’t fully understand how they work (Kosinski, 2025). This means that even if the results are biased, it’s hard to track down the bias or fix it. The user doesn’t know how the model came to its conclusions-what factors it considered, how it weighed them, and so on.
So, AI bias isn’t a bug, it’s a “feature”, it mimics our world in a way that’s all too real. The more we believe in AI’s “objectivity,” the more easily we are misled by its “perfectly disguised biases. There are many real examples in real life that let us know how AI discrimination and algorithmic bias are frequently integrated into our lives.
Real-World Examples of Algorithmic Bias
Case 1: Amazon’s AI Recruitment System
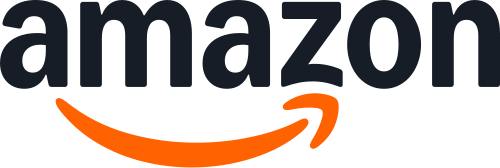
Source: Wikipedia
Amazon used to have an AI system specifically made for recruiting. The original intention of this system was to automatically screen out the best candidates through machine learning. But a few months later, Amazon was surprised to find out: it severely discriminated against women (2018).
According to Reuters, by 2015 it was clear that the system was not rating candidates in a gender-neutral way, as it was built on data accumulated from resumes submitted by the company, which were predominantly from men (2018). The system penalized resumes that included the word “female.” (2018). Eventually, Amazon had to scrap the system, but the system was not as effective as it could be. But this example has made everyone realize that AI replicates social bias. During this period, many women lost the job opportunities they should have had. If this problem is not discovered in time, the consequences will be more serious.
Case 2: Credit scoring and lending
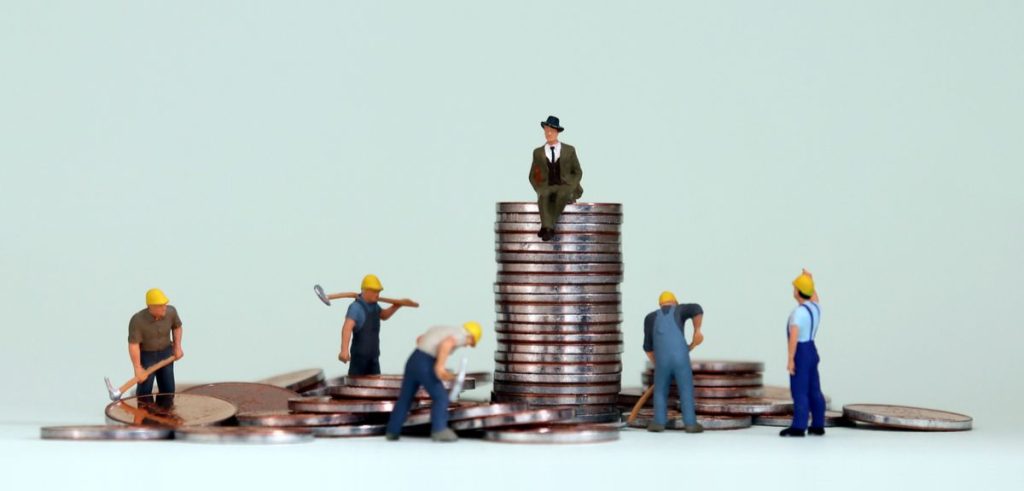
Source: University of Oxford
In addition to the justice and hiring fields, the problem of algorithmic bias in credit scoring systems is just as serious. An article from the Stanford Human Centre’s AI Institute points out that many of the AI models used to determine loan eligibility rely on flawed, incomplete credit data, and that these biases are particularly common among low-income, people of colour, and other groups (Andrews, 2021).
For example, some people simply can’t establish a “good credit history” because of the nature of their jobs, which don’t have regular incomes, or because they come from neighbourhoods without banking systems. But AI doesn’t understand these complex social contexts, it will simply “take for granted” a low score, or even reject a loan application outright. As the researchers put it, “These models operate on the wrong foundation, so they will inevitably exacerbate inequality.” (Andrews, 2021). In other words, it’s not that they don’t have credit, it’s that they don’t have the right to be recorded.
Why is “data neutrality” an illusion?
Scholars point out that while digital platforms “decentralize” information, they also break down the authority of traditional media and institutions, making information more subjective and pluralistic in its judgments, but this freedom makes people more inclined to accept only the content that conforms to their preconceived notions content (Livingston and Bennett 2020). So, when algorithms are linked to recommender systems and AI models, they inherit the property that algorithms will be more likely to recommend content that users want to see, and more likely to amplify pre-existing social biases. Exaggerating a single push content provides profits for capitalists, while users will lose the diversity of content they obtain and the ability to discern correct information. Fake news will become more rampant.
Similarly, argues that the business logic of social platforms is based on the core metric of “user stickiness”, so the system is biased towards pushing more emotional and polarized content to increase engagement (Flew 2021). The problem is that this algorithmic mechanism not only affects news, but also may affect AI scores, social ranking, and even credit assessment.
So, algorithms and AI they’re not just a string of techy code, power and capital are manipulating them while providing convenience to humans. It also becomes an extension of power, a cultural mechanism. Hidden behind it are the assumptions of the developers, the business goals of the platforms, the sedimented biases of the social structure. The so-called data neutrality is simply an “illusion”.
If algorithms discriminate, what can we do?
In reality, AI and algorithms are used in everything from Google searches to TikTok video content, to algorithmic models used by banks to approve loans, by companies to screen resumes, and even by the police to determine if you are a “potential offender”. It’s simply not possible to stop using AI and algorithms altogether. That’s why we need algorithmic governance.
What can we do? First, there is transparency. The platform’s algorithms don’t tell you “Why this is being pushed” on their own (Bruns et al., 2021). Without transparent logic, there is no possibility of accountability. We need more “explainable” AI systems, not just for engineers, but for the average user to understand the logic of how AI works, and how AI and algorithms work needs to be “seen” by users. The second is to introduce diverse data sources. Low-income people, people of color, freelancers, etc., can be treated fairly.
Furthermore, and most importantly, there is a need to strengthen regulatory mechanisms. In its draft AI regulation, the EU has proposed to include certain “high-risk AI systems” into the scope of special regulation, such as recruitment, education, justice and other important areas involving the fate of individuals (2023). This is to ensure that the majority of the public’s life will not be caused by the bias and mistakes of machines that lead to bad results. This is also an attempt to intervene institutionally in the power structure of algorithms and provides us with a governance paradigm to follow.
Finally, social and ethical education is indispensable. We need to teach engineers not only “how to write good algorithms”, but also “why to question algorithms”. The general public should also be aware of what algorithms decide and their right to question the legitimacy of the technology. As Massanari emphasizes, platform culture and algorithm design are inextricably linked. Changing AI bias is not just an engineering problem, but a political, cultural, and civic issue (Massanari, 2016).
Conclusion:
When we question why algorithms discriminate and why AI is unfair, what we should really be looking at is actually us who shape these technologies. Algorithms aren’t created out of thin air, they are nourished by specific social structures, business logics, and cultural biases. In other words, they inherit our imperfections.
A “machine” is never a mere technological entity, but an extension of human choices: who trains it, what data is fed into it, what optimization goals are set, and in which areas it is allowed to make decisions – all of these are human decisions.
Therefore, solving the problem of “algorithmic discrimination” is not just a technical challenge, but also a social, ethical and political one. We must demand more transparent systems, more accountable platforms, more binding regulation, and broader public participation.
Reference
Andrews, E. L. (2021, August 6). How flawed data aggravates inequality in credit. Stanford HAI. https://hai.stanford.edu/news/how-flawed-data-aggravates-inequality-credit
BBC. (2018, October 10). Amazon scrapped “sexist AI” tool. BBC News. https://www.bbc.com/news/technology-45809919
Bruns, A., Harrington, S., & Hurcombe, E. (2021). ‘Corona? 5G? or both?’: The dynamics of COVID-19/5G conspiracy theories on Facebook. Media International Australia, 177(1), 12–29. https://doi.org/10.1177/1329878X211005889
EU AI act: First regulation on artificial intelligence: Topics: European parliament. Topics | European Parliament. (2023, June 8). https://www.europarl.europa.eu/topics/en/article/20230601STO93804/eu-ai-act-first-regulation-on-artificial-intelligence
Flew, T. (2021). Disinformation and Fake News. In Regulating Platforms (pp. 86–91). Cambridge: Polity Press.
Kosinski, M. (2025, January 15). What is black box AI and how does it work?. IBM. https://www.ibm.com/think/topics/black-box-ai
Livingston, S., & Bennett, W. L. (2020). A Brief History of the Disinformation Age: Information Wars and the Decline of Institutional Authority. In S. Livingston & W. L. Bennett (Eds.), The Disinformation Age: Politics, Technology, and Disruptive Communication in the United States (pp. 3–40). Cambridge: Cambridge University Press.
Massanari, A. (2016). #Gamergate and the fappening: How reddit’s algorithm, Governance, and Culture Support Toxic Technocultures. New Media & Society, 19(3), 329–346. https://doi.org/10.1177/1461444815608807
Noble, Safiya U. (2018). A society, searching. Algorithms of Oppression, 1–63. https://doi.org/10.2307/j.ctt1pwt9w5.5
Be the first to comment