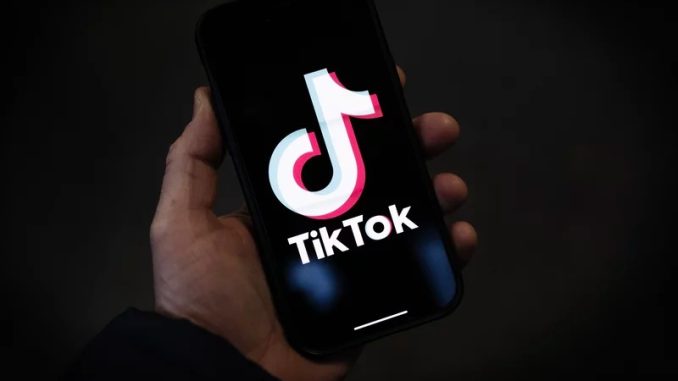
In the past, people usually obtained news or topics through active searching, and decided for themselves what information they wanted to know. However, as society becomes increasingly digitalised, algorithms are quietly replacing this initiative. Take TikTok, for example. One of the world’s most popular social media platforms, its algorithm system has been deeply embedded in content push and filter mechanisms, and is increasingly becoming a key regulator of users’ access to information. TikTok’s recommendation algorithm is based on artificial intelligence technology, which is responsible for screening, sorting and recommending content that users may be interested in. They determine what people see, believe, and even discuss. However, the decision-making logic behind this system is almost invisible to the outside world. Users have no way of knowing exactly how the algorithm makes its judgments, and there is no mechanism to oversee the fairness of its operation.
What is even more worrying is that when these algorithmic systems begin to influence the public opinion environment, public discussion, and even political choices, the platforms rarely take responsibility for their social consequences. This is not just a technical issue, but a core issue about user rights and platform governance. This blog post will use TikTok as a case study to explore its algorithmic transparency issues, and analyse the responsibilities and roles of policies, platforms and users in information governance.
The dominant player in information distribution: the rise of recommender system
On TikTok, the sheer volume of content has completely changed the way people access information. Users do not need to actively seek content—they’re fed an endless stream of videos the moment they open the app. and this content is not presented at random, but has been filtered by the recommender system behind the platform. It tends to show content that users like. Recommender systems are an algorithm-based personalised information filtering tool, and their goal is to optimise the experience for individual users. Specifically, recommender system analyse users’ behavioural data and use algorithms to filter through a set of known, discrete options to provide users with targeted suggestions. The essence is not to create information, but to personally reconstruct the information flow. (Burke et al., 2011). For users, this push mechanism does improve the TikTok experience, saves users the cost of searching for information, and improves efficiency. For TikTok, Recommender systems mean longer user retention, which can bring in more advertising revenue. As users use it, the content recommended by the platform will gradually match the user’s preferences. This increases the user’s dependence on TikTok.
On the surface, Recommender system improve the user experience and bring benefits to TikTok. However, behind this seemingly ‘mutually beneficial’ mechanism, there is a hidden shift of control over information and a lack of transparency. When users go from ‘finding content’ to ‘being found by content’, it means that they have given up active control over the information. What is even more worrying is that the logic behind the ‘choices’ made by the algorithm is completely opaque. We are shaped by algorithms every day, but we have no idea how they work.
Problems caused by the black-box of algorithms
As a core tool for pushing TikTok content, the recommender system almost universally influences the information users are exposed to. However, the operating logic of this system is generally in a ‘black-box’ state. A black-box is a system where users can only see the input and output, but it is completely unclear how it works internally (Pasquale, 2015). In TikTok, users cannot understand how the algorithm determines that they will like a certain piece of content, nor do they know what criteria the platform is using to sort and push content. As users continue to filter content, this lack of transparency in the algorithm mechanism will gradually become biased.
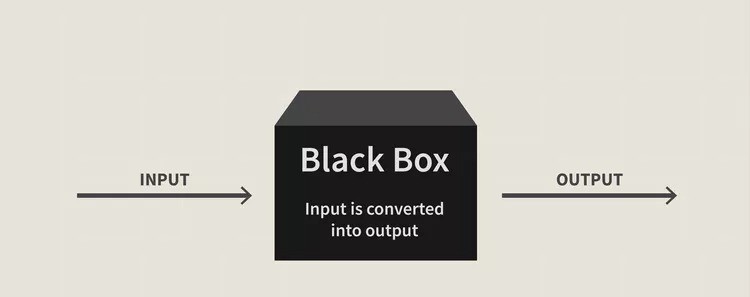
Put simply, when we browse TikTok content, the platform will use recommender systems to analyse our browsing, clicking, liking and other behaviours, in order to push content that we are more likely to like. Over time, the content and tendencies of the information users see will continue to solidify. This kind of content push based on ‘user preference feedback-algorithmic recommendation’ gradually strengthens users’ existing interests and positions, reducing their chances of being exposed to heterogeneous views, thus contributing to the formation of an “information cocoon”. The information cocoon is defined as a situation in which information is exchanged, and users focus on topics that interest them, forming a kind of “cocoon” that excludes or ignores other views and content (He et al., 2023).
The formation of an information cocoon is accelerated by recommender system. And because the algorithm’s operating logic is in a black-box, users are unlikely to realise the bias of the content they receive. This lack of information makes it difficult for users to detect the formation of an information cocoon. Under the long-term influence of the information cocoon, people’s continued exposure to homogeneous information will accelerate the tendency towards extreme views. This cognitive closed loop not only strengthens the group’s absolute identification with its own position, but also leads to a value opposition between different groups. Some studies have pointed out that during the 2024 U.S. presidential race, TikTok had a ‘negative partisan bias’ when recommending political content, that is, the platform was more inclined to recommend content attacking the opposing party than promoting content supporting the party (Ibrahim et al., 2025). This also proves that the long-term information cocooning effect will weaken the consensus foundation of society and exacerbate the division between groups. On the other hand, it is quite difficult to crack an information cocoon. As Professor Andrejevic said, the difficulty of information cocoon governance is its unpredictability. When supporters of one side are exposed to the facts, ideas, ideologies or values held by the other side, when people are exposed to opposing views and evidence, it rarely really triggers thoughtful reflection. Instead, it often makes people’s positions even stronger (Andrejevic, 2019). Due to the unpredictability caused by the opacity of algorithms, it is difficult for regulators to understand how algorithms categorise users and what rules they use to make recommendations. Therefore, it is also difficult to design effective intervention and regulation mechanisms.
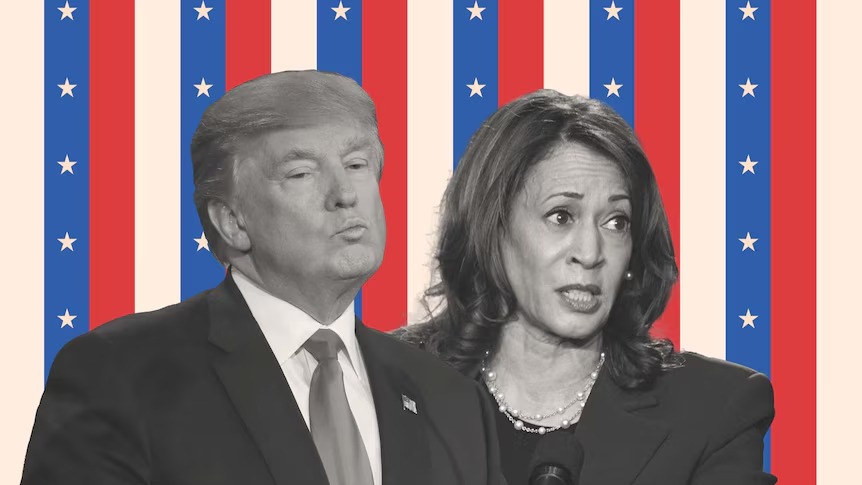
Recommender systems not only determine what content users can see, but also hide content from users. This is the core mechanism of ‘shadowbanning’. The phenomenon of repeated shadowbanning of marginal groups on the ‘For you’ page in TikTok reveals the algorithmic bias that may be embedded in these recommender system (Delmonaco et al., 2024). This algorithmic bias will continue and even exacerbate real-world biases, resulting in some groups being systematically marginalised, with no way to appeal or rectify. And due to the black-box nature of the algorithm, this phenomenon is difficult to detect and impossible to regulate.
Because recommender system profoundly affect the way people access information and the structure of social cognition, the opacity of their algorithms is particularly alarming. When these ‘invisible screening’ mechanisms begin to influence public opinion, public discourse, and even political judgments, algorithms are no longer just a technical tool. Their decision-making mechanisms are quietly reshaping the way society as a whole operates. Faced with this situation, policymakers have gradually realised that self-regulation by platforms is no longer sufficient to deal with the increasingly complex impact of algorithms. Therefore, policies on how to effectively govern TikTok’s algorithm system have increasingly become the focus of policymaking.
Policy response: the practice and limitations of algorithmic governance
In recent years, many regions, including the European Union, have attempted to regulate platform algorithm systems through legal means. In Europe, the General Data Protection Regulation (GDPR) “issued by the European Union stipulates that platforms need to explain to users the existence, operating logic, decision-making mechanism and expected impact of the recommender system (GDPR, 2016.). In Asia, China’s Personal Information Protection Law of the People’s Republic of China (PIPL) stipulates that users have the right to request platforms to explain the decision-making logic of their recommender system and to refuse decisions made by platforms solely through algorithms (PIPL, 2021.). The goal of these laws is to make platforms more responsible for their content recommendation and filter mechanisms, and to make algorithms transparent and controllable for users.
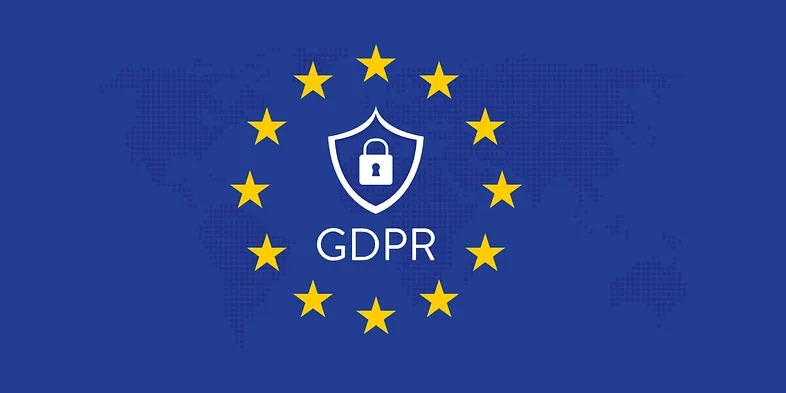
These policies represent the main approaches to algorithmic governance, but they are not enough to solve the problems caused by algorithmic opacity. Take TikTok as an example. The platform has long regarded its recommendation algorithm as its core competitiveness, and it usually reserves its position on algorithmic transparency on the grounds of ‘commercial secrets’. Although policies such as the GDPR have begun to require platforms to provide a certain degree of algorithmic transparency, they still face multiple challenges at the implementation level, such as insufficient cooperation from the platform, insufficient user awareness, and immature regulatory mechanisms.
This leads us back to a more fundamental question: does TikTok need to take responsibility for its algorithmic systems? If the platform continues to regard the recommender system as purely technical and ignores its impact on society, then even if policies require the disclosure of algorithmic logic, it may be reduced to a mere formality. In this sense, whether algorithmic transparency can be truly achieved depends on whether TikTok fulfils its governance responsibilities.
Platform roles: from technology intermediary to community manager
If TikTok continues to avoid its social responsibilities, then there is no way to talk about algorithmic transparency. The reason for this is that the platform lacks sufficient incentive to govern itself. From the perspective of the platform, the recommendation system and the algorithmic logic behind it are highly controllable technical means that can increase user retention, optimise advertising, and even invisibly guide public opinion. However, these algorithms have long since transcended their instrumental nature and have become an important mechanism for platforms to govern user behaviour and shape public discourse. Platforms have in fact come to play a role in the digital space similar to that of a ‘community administrator’ – they determine how information flows and which voices are amplified, profoundly influencing user perceptions and social discussions.
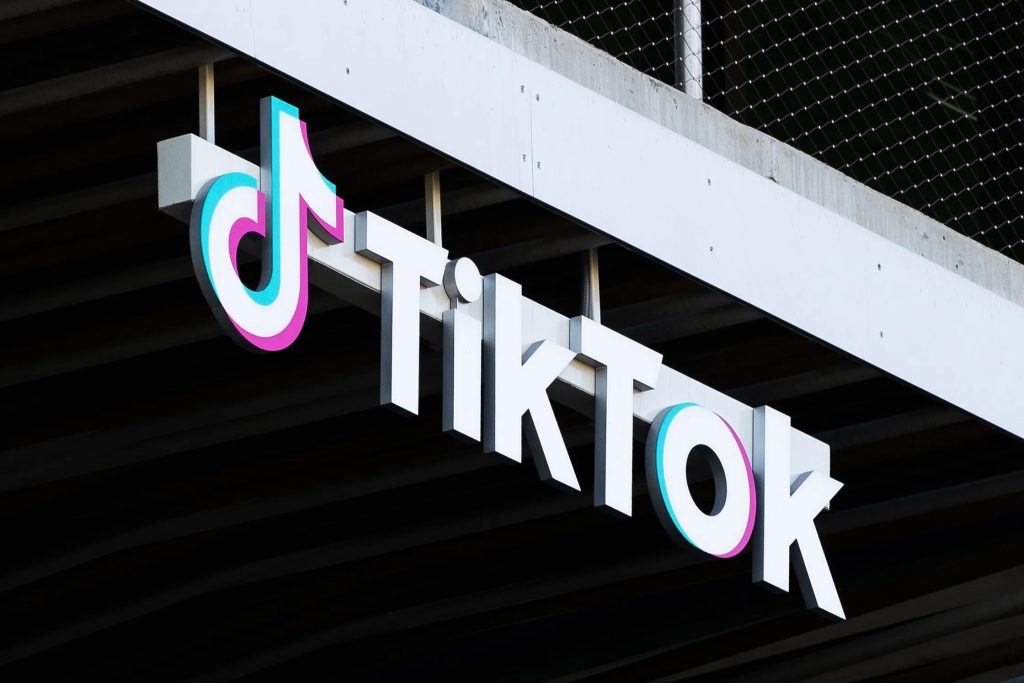
Therefore, TikTok should not only bear corresponding responsibility for the design goals and potential consequences of the algorithm system, ensuring the fairness of the mechanism and the user’s right to know and choose, but also bear substantive responsibility at the institutional and governance levels. Only in this way can the algorithm system go beyond technical embellishment, truly open up to the public, and create a fair and trustworthy information environment under the framework of legal and social supervision.
Collaborative governance: towards a transparent and accountable algorithmic future
In an algorithmic filter information environment, each of us is constantly being ‘guessed’ and ‘shaped’ by the system. The filtering and pushing of information is no longer just a matter of technology, but also a governance issue about who has the right to speak, who sets the standards, and who supervises power. When TikTok intervenes in the public space through a recommender system, it is not just a commercial company, but also a key shaper of the digital society. The platform should take the initiative to assume its responsibilities, not only due to compliance pressures, but also based on a conscious response to the impact on society. At the same time, users need to improve their understanding of algorithms, realise that algorithmic content recommendations are not neutral, question information sources, enhance their thinking about recommendation logic, and actively break out of the information cocoon constructed by algorithms.
Improving the transparency of algorithms is a long and complex process that cannot be achieved overnight. However, only by establishing a mechanism for sharing responsibility and disclosing information between the law, platforms and the public can the social conflicts and information cocoon caused by algorithms be mitigated. Transparency in algorithms means visibility of power, traceability of decisions and controllability of consequences. We may not be able to read every line of code in an algorithm, but we have the right to know how it is affecting our world.
Reference
Andrejevic, M. (2019). Automated Media. Routledge. https://doi.org/10.4324/9780429242595
Bobby Allyn. (2023). The Biden administration demands that TikTok be sold, or risk a nationwide ban. https://www.npr.org/2023/03/15/1163782845/tiktok-bytedance-sell-biden-administration
Burke, R., Felfernig, A., & Göker, M. H. (2011). Recommender Systems: An Overview. AI Magazine, 32(3), 13–18. https://doi.org/10.1609/aimag.v32i3.2361
Cory Doctorow. (2023). The ‘Enshittification’ of TikTok | WIRED. https://www.wired.com/story/tiktok-platforms-cory-doctorow/
Delmonaco, D., Mayworm, S., Thach, H., Guberman, J., Augusta, A., & Haimson, O. L. (2024). ‘What are you doing, TikTok?’: How Marginalized Social Media Users Perceive, Theorize, and ‘Prove’ Shadowbanning. Proc. ACM Hum.-Comput. Interact., 8(CSCW1), 154:1-154:39. https://doi.org/10.1145/3637431
Elissa Steedman. (2024). Here’s a simple guide to how presidential elections work in the US. ABC News. https://www.abc.net.au/news/2024-10-12/when-is-the-us-election-/104063256
GDPR. (2016). General Data Protection Regulation (GDPR) – Legal Text. https://gdpr-info.eu/
He, Y., Liu, D., Guo, R., & Guo, S. (2023). Information Cocoons on Short Video Platforms and Its Influence on Depression Among the Elderly: A Moderated Mediation Model. Psychology Research and Behavior Management, 16, 2469–2480. https://doi.org/10.2147/PRBM.S415832
Ibrahim, H., Jang, H. D., Aldahoul, N., Kaufman, A. R., Rahwan, T., & Zaki, Y. (2025). TikTok’s recommendations skewed towards Republican content during the 2024 U.S. presidential race. https://doi.org/10.48550/arXiv.2501.17831
Pasquale, F. (2015). The Black Box Society: The Secret Algorithms That Control Money and Information. Harvard University Press. https://www.jstor.org/stable/j.ctt13x0hch
PIPL. (2021). Personal Information Protection Law of the People’s Republic of China. Retrieved 10 April 2025, from https://personalinformationprotectionlaw.com/
Thilanka Dilakshi. (2021). GDPR and Web Development. https://medium.com/emblatech/gdpr-and-web-development-5a63654686e8
Will Kenton. (2024). What Is a Black Box Model? https://www.investopedia.com/terms/b/blackbox.asp
Be the first to comment