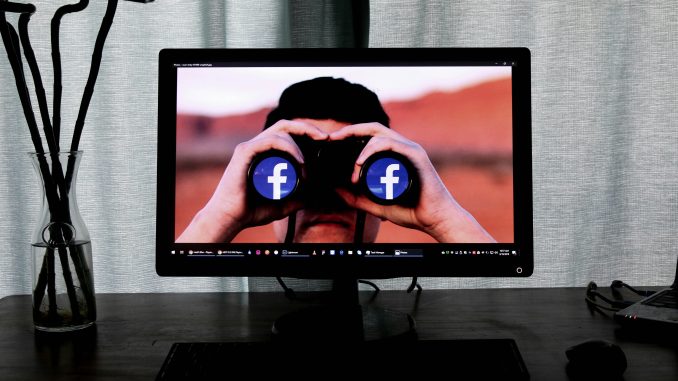
With the advent of the digital age, it seems like our lives cannot do without the internet. Whenever you shop online, you are always pushed towards things you want. As we continuously scroll through TikTok or interact on Tinder searching for true love, it inevitably makes us wonder: why is it so addictive?
The answer lies in data, which acts as the new oil of the era and is being greedily mined by major capitals. (Flew, 2021, P79) Our behaviors, preferences, online time, dwell time, and more exist in the form of data and are being analyzed. Platform predicts our preferences through our data, speculates on our behavioral patterns, or sells them for monetization to achieve various political purposes. (Reuning, 2022) (Hitlin & Rainie, 2019, P3) Due to the invisibility, opacity, and difficulty in tracking of data trading, our privacy is difficult to protect. With the introduction of algorithms and artificial intelligence technologies, more issues arise, and data privacy and security have become hot topics. The efficient use of personal data can bring economic benefits but also poses challenges to privacy and security.
Facebook is a social networking company founded by Mark Zuckerberg and is based in California, USA. (Figure 1) It is one of the largest social media platforms globally, with over 2 billion monthly active users. (Simplilearn, 2023) Facebook holds vast amounts of personal information and social networks of its users. This data is used to provide personalized content to users, analyze popular trends, and target advertisements. With a massive user base and excellent algorithms, Facebook continuously optimizes to provide users with a better experience and enhance its commercial value.
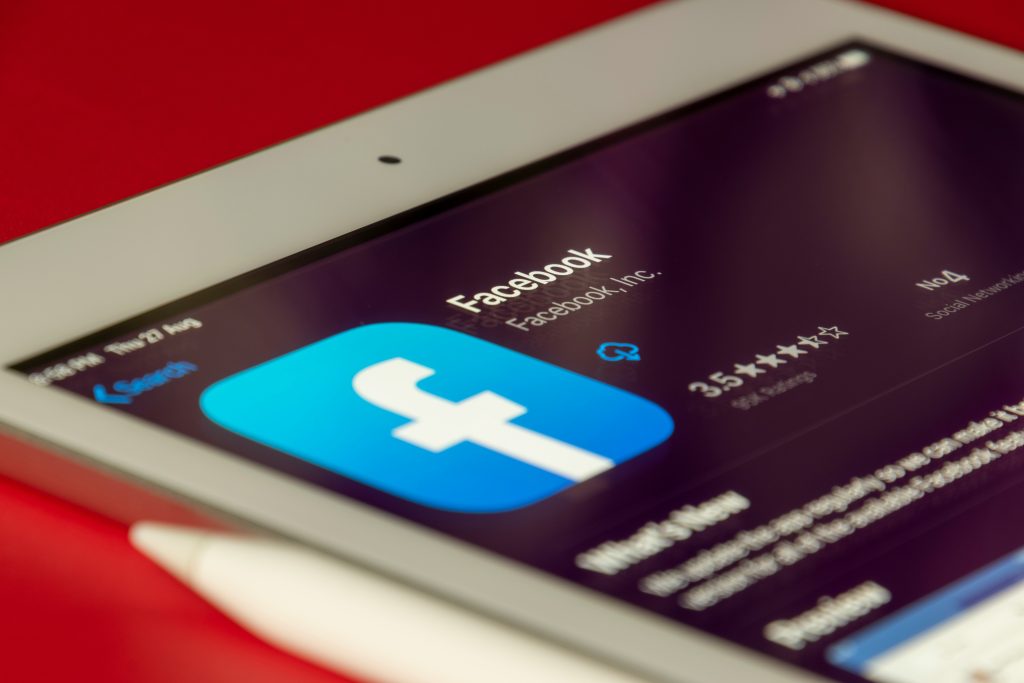
Figure 1 Image from: unsplash
Facebook’s big data predictive ability and information cocoon/echo chamber.
Every time we open Facebook, we are amazed by its unique push mechanism. You can see people you know in real life on the homepage and add them as friends, receive ads that interest you, or come across a video in your first language on the reel feature. Why is it always so accurate? Because it leverages the advantage of big data. Facebook’s vast amount of data forces it to be efficient in processing information. Unlike other social apps, Facebook users tend to display more genuine information about themselves (such as name, profile picture, school, location, occupation, etc.) to express “sincerity” towards other users (Dwyer, 2007), which allows Facebook to further understand its users. Not only personal information, but also non-personal information such as Deidentified or pseudonymous and aggregated data is collected when users are in anonymous status. (Flew, 2021, P79) This digitizes users’ social actions and is a continuous, opaque behavior. (Flew, 2021, P80) The next step after digitized social behavior is monetization, where Facebook brings in a large number of users and opportunities for many advertisers and achieves a high conversion rate through algorithmic screening.
This seems like a win-win-win situation: Facebook commercializes social behavior, users get a better user experience, and advertisers get a higher customer conversion rate. However, the use of opaque, untraceable data not only increases users’ security risks but also steers social behavior into an information cocoon. (Li, 2022) With its user data, Facebook can easily predict user behavior and preferences. This makes its algorithm intentionally “please” users, in exchange for longer usage time and frequency, thereby gaining more user data and forming a cycle. For example, Facebook pushes political topics on the homepage because its user base is mainly outside China. Therefore, posts in Chinese may be pushed with speculative or derogatory meanings, but after users show disinterest, Facebook will push positive evaluations about China. Such algorithms and the use of big data fuel the growth of extremism, causing people to get stuck in their pre-existing stereotypes and find like-minded groups. This could lead to more extreme behaviors such as hate speech and physical threats. (Flew, 2021, P91) This is also common on TikTok, where people only see what they want to see and become addicted to it. TikTok’s success has led Facebook to adopt similar practices, and these similar algorithms have made them successful in business. However, morally, it reinforces people’s stereotypical influences, incites mutual conflicts, and causes antagonism, which is unethical behavior. This is another reminder that algorithms are influenced by various interests and are not absolutely fair or rational. ” And due to the capital required to build AI at scale and the ways of seeing that it optimizes AI systems are ultimately designed to serve existing dominant interests. In this sense, artificial intelligence is a registry of power.” (Crawford, 2021)
Algorithm and AI: Who is making all the decisions?
Massive data, if not effectively utilized, becomes a huge data exhaust. (Bronson, 2015) Therefore, algorithms are crucial for processing data. Facebook’s algorithm is kept as a trade secret and not publicly disclosed, making users feel like Truman (from the movie “The Truman Show,” where Truman is the protagonist of the film and everything he sees is arranged. It’s a reality show, only Truman doesn’t know). They can’t decide what they see, but unlike Truman, Facebook’s users may not be dealing with people, but with AI robots, which analyze massive user data and give them rating grades to provide users with a customized experience. (Bodle, 2014) However, this is all reverse engineering by researchers, and the real algorithm remains a black box to the outside world. (Flew, 2021) The highly customized content leads to the further marginalization and unfair treatment of minority groups. The algorithm exacerbates this phenomenon, as the content pushed to minority groups may be determined by identity rather than content, leading to the opinions or views expressed by minority groups being pushed to only a few people (Hitlin & Rainie, 2019, P3), further marginalizing their identity.
Additionally, the generation of algorithms labels users and even changes the traditional concept of the younger generation. Tinder is a well-known online dating app that has attracted a large number of users with its simple user interface and easy operation, especially among young people. However, with the proliferation of user data, images with sexualized bodies tend to make users pause and gain more exposure, and the algorithm is more willing to push them to related users. Over time, this makes users pay more attention to appearance, forming a distorted aesthetic concept and unhealthy intimate relationships. Furthermore, platforms adopt a logic similar to gambling to increase users’ usage frequency and time. User behavior is very simple, requiring only simple gestures. All other dazzling content is provided by the algorithms. This further reduces users’ thinking ability, solely for the sake of gaining more sensory experiences.
Algorithms also lead to content homogeneity. The algorithm acts like a directional filtering system, scoring posts and pushing them to the audience. However, this process is opaque, and the push strength is largely determined by the number of likes. When people come across a video on Facebook reels with hundreds of thousands of likes from a famous influencer, they are more likely to pause, watch the video, and even join the likes. However, when they accidentally come across a video with only a few likes, they tend to swipe away. The result is an unequal attention to create content, making content more homogeneous and diminishing ordinary people’s desire to share.
Facebook is not a safe box: Facebook–Cambridge Analytica data scandal.
The concentration and high-frequency use of massive data are not safe. Users’ social activities generate data for Facebook, but that doesn’t mean Facebook has the right to violate users’ privacy. One notorious incident is the Facebook–Cambridge Analytica data scandal. Cambridge Analytica utilized an app called “This Is Your Digital Life” through Facebook’s platform to collect personal data from millions of users. This app not only gathered data from these users, but also acquired data from their Facebook friends, resulting in unauthorized data collection of millions of users. This data included personal information, likes, interests, and social relationships, creating detailed user profiles used for targeted advertising. (Brittany, n.d.) This data was used for analysis and precise ad targeting, primarily for political ads. All of this was done quietly, without users’ knowledge. The subsequent exposure of the event sparked widespread discontent, leading to the #DeleteFacebook movement on Twitter. (Brittany, n.d.) However, this data was still collected by another platform. This makes us wonder, does our data really not belong to us? How can we break free from this vicious cycle?
The widespread use of big data and algorithms by capital is like a double-edged sword, with personalized content and privacy protection seeming to be at odds and unbalanced. Avoiding overexposure of personal information while using social software is not a unilateral binary issue but requires joint solutions. Firstly, from the perspective of enterprises, when taking actions that involve user data, they need to ensure the security and transparency of the process. This prevents the abuse of user privacy and enhances user trust in the platform. Additionally, platforms need to consider ethical aspects in their push mechanisms, reduce the use of inducing and addictive mechanisms, and ensure users’ psychological well-being. Making parts of the algorithm public is also crucial, as it can reduce bias. Secondly, platforms need to carefully use user data and audit third-party platforms to prevent data misuse. However, the mainstream platforms’ attitudes towards data are often unclear, with privacy-related terms hidden deep in the software, intentionally causing users to overlook privacy concerns or forcing users to release partial permissions and data under the guise of opening privacy for functionality. On the government side, regulation of companies with large amounts of user data is necessary to avoid lagging behind (Flew, 2021, P91). Additionally, relevant government departments need to enhance their understanding of big data. As users, we spend a considerable amount of time on various platforms, “Every 60 seconds, 136,000 photos are uploaded, 510,000 comments are posted, and 293,000 status updates are posted.” (Simplilearn, 2023) This means platforms have a constant stream of fresh, active data. On one hand, we need to safeguard our privacy from interference by others on the platform, and on the other hand, we need to ensure our data is handled reasonably by the platform. In short, when it comes to balancing data abuse and privacy protection in the digital age, we need to consider the responsibilities and roles of businesses, governments, and individuals, as well as the interplay of their interests and ethical considerations.
Conclusion
In the context of the digital age, platforms seem to be changing our lives in ways we never anticipated. With personal data being collected, analyzed, and utilized on a massive scale, our emotions and individual characteristics seem to be stripped away, turning into predictable, controllable digital models. Social behavior, intimate relationships, and even personal identities are being digitized, becoming inputs and outputs of data algorithms. It’s as if there’s an invisible eye watching everything about you. Behind this digitization lie significant ethical challenges and societal issues.
While personal data bring us more convenient experiences and economic growth, it also comes with more challenges. We urgently need to be vigilant and cautious in how we handle and protect personal data. Platforms should be more transparent in their use of data and be subject to user oversight to ensure the fair use of data. In this digital age, we need not just technological advancements, but also respect for individual privacy and rights.
We need eyes that look at each other equally, not hidden eyes peeking from the shadows.
Reference:
Brittany Kaiser. (n.d.). #DeleteFacebook movement. Wikipedia.
Bronson, N., Lento, T., & Wiener, J. L. (2015, April). Open data challenges at Facebook. In 2015 IEEE 31st international conference on data engineering (pp. 1516-1519). IEEE.
Bodle, R. (2014). Predictive algorithms and personalization services on social network sites: implications for users and society. In The Ubiquitous Internet (pp. 130-145). Routledge.
Crawford, K. (2021). The atlas of AI power, politics, and the planetary costs of artificial intelligence. Yale University Press.
Dwyer, C., Hiltz, S., & Passerini, K. (2007). Trust and privacy concern within social networking sites: A comparison of Facebook and MySpace. AMCIS 2007 proceedings, 339.
Flew, T. (2021). Regulating platforms. Polity Press.
Hitlin, P., & Rainie, L. (2019). Facebook algorithms and personal data. Pew Research Center, 16, 1-22.
Li, N., Gao, C., Piao, J., Huang, X., Yue, A., Zhou, L., … & Li, Y. (2022, October). An Exploratory Study of Information Cocoon on Short-form Video Platform. In Proceedings of the 31st ACM International Conference on Information & Knowledge Management (pp. 4178-4182).
Reuning, K., Whitesell, A., & Hannah, A. L. (2022). Facebook algorithm changes may have amplified local republican parties. Research & Politics, 9(2). https://doi.org/10.1177/20531680221103809
Sprout Social Insights. (n.d.). Social media algorithms: How they work and how to outsmart them. Retrieved from https://sproutsocial.com/insights/social-media-algorithms/
Simplilearn. (2023). How Facebook is Using Big Data. Retrieved from https://www.simplilearn.com/how-facebook-is-using-big-data-article
Be the first to comment