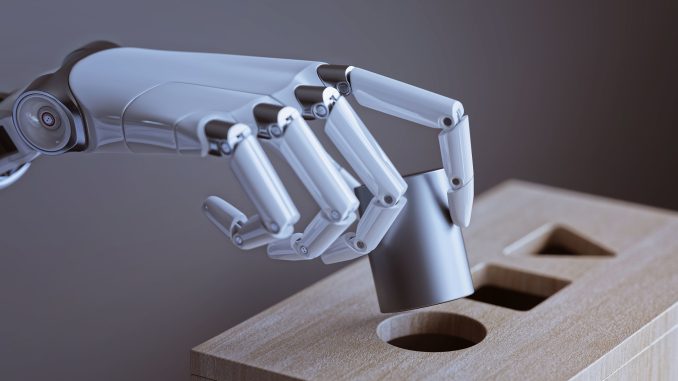
Introduction
Imagine one day after waking up, you receive an email from the government stating that you owe the government tens of thousands of dollars. Moreover, imagine having to undergo debt repayment while you still disputing the debt. Will your trust in the government be affected?
From 2016 to 2019, the Australian government utilized Centrelink’s employment income confirmation system to quickly check whether citizens’ reported data matches data gathered from other government agencies to help the federal government recover welfare debt. This Automated Decision-Making (ADM) system autonomously generates debt notices and does not require any further human review or explanation (Sarder, 2020). Ultimately, this initiative proved to be a disaster, erroneously collecting over 470,000 debts, approximately AUD$721 million (Sarder, 2020). The debt is called robodebt, and experts pointed out that this was a possible problem due to automated decision-making.
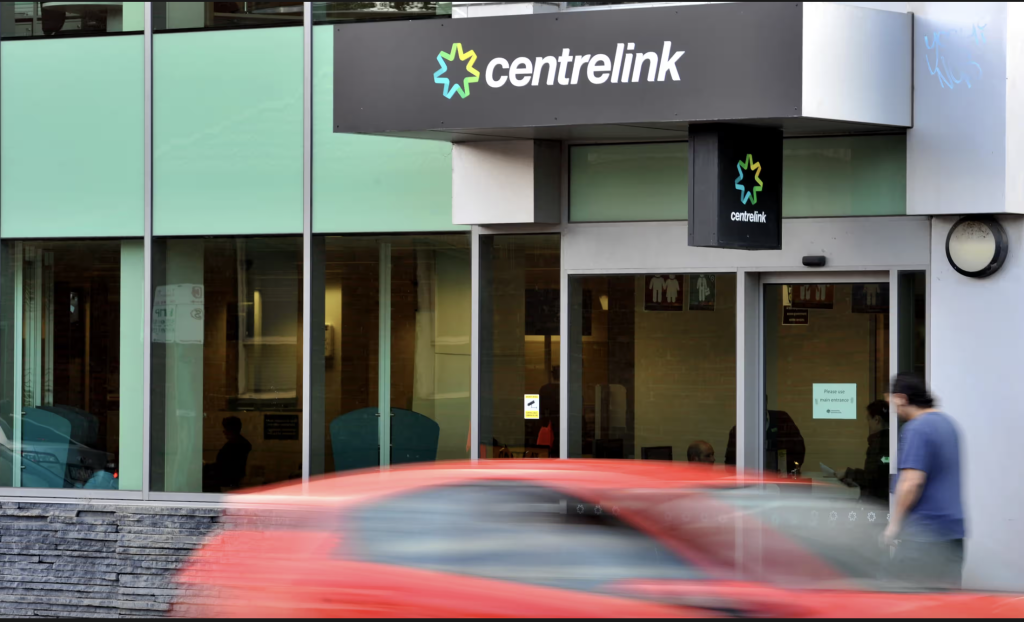
Millions of Australians rely on the income from Centrelink (Zhou,2018)
In the digital age, algorithms are uniquely changing the way of human decision-making. Nowadays, with the expansion of algorithmic applications and the impact of pandemics, algorithmic decision-making, an AI autonomous decision-making system centered on machine learning and deep learning algorithms (Zouridis et al, 2020, as cited in Evans & Hupe, 2020), is no longer stopping at the commercial domains where people are already maturely using it but is gradually transitioning from private to public life. These automated decisions determine the public choices, opportunities, and legal status in public areas such as immigration, education, and justice. Algorithms are deeply embedded in government governance being used to make important decisions, giving it social as well as political power (Elbanna & Engesmo, 2020).
As algorithms are deciding to have a huge impact on a wide range of things in the lives of ordinary people, the issue of risk in algorithmic decision-making is also attracting more and more vigilance and discussion. The Robot Debt Fiasco primarily resulted from biases inherent in the autonomous decision-making system, leading to algorithmic biases, discrimination, and exacerbation of inequalities (Redden, 2022). It also violates the human rights of citizens. Many families experienced life breakdowns due to being wrongly accused of welfare fraud, leading to severe depression and anxiety. Some even claimed that Centrelink had “robbed” them. Moreover, the Royal Commission vividly depicted the government’s irresponsibility in the robodebt incident (O’Donovan, 2023). The lack of accountability and strong oversight gives the government an excuse to shirk its responsibilities, suggesting that there is room for improvement in governance.
Algorithmic systems have altered decision-making processes and potential forms of public oversight (Harkens, 2020). Faced with varying degrees of threats posed by algorithmic decision-making processes, human-machine co-governance is an important means of improving the effectiveness of public decision-making while minimizing the risks posed by algorithms.
Rise of Robotic Decision-Making
To promote the digitization of government processes, governments actively promote the application of algorithms. On the one hand, the data generation and processing capabilities of Artificial Intelligence offer rich decision-making information for algorithmic decisions in the public domain. In the face of the massive amount of information that fills various complex fields as well as activities, Artificial Intelligence, compared to humans with high costs, slow speed, and lower accuracy, can rapidly, conveniently, and massively process information, reducing significant costs and enhancing efficiency (Roose, 2019). On the other hand, the predictive analytics of Artificial Intelligence can provide direct technical support for algorithmic decision-making. Kleinberg et al. (2015) states that algorithms are expected to make decisions more accurately, fairly, and consistently than humans. The government is gradually looking at the technicality of the algorithms as a way of guaranteeing impartiality (Flew, 2021).
However, while algorithmic decision-making significantly improves the rationality of public decision-making processes, it also continually challenges the boundaries of law and ethics. As Artificial Intelligence continues to penetrate public governance, attributing responsibility becomes increasingly difficult (AI-Amoudi & Latsis, 2019), allowing persons in charge in the public sectors to use Artificial Intelligence algorithms as excuses to evade accountability (AI-Amoudi, 2021).
Concerns in Algorithm Decision-Making
Algorithmic black box
The “black box” and opaque nature of algorithmic decision-making makes policy implementation less transparent and participatory, leading to unclear responsibilities and difficulties in accountability. On the one hand, the complex Artificial Intelligence algorithms themselves are a “black box” (Burrell, 2016), with inherent opacity. The algorithm-driven public decision-making process also has the nonlinear, highly interactive features present in real policy processes in the public sectors. So, it involves a large number of variables in the computational process, making the prediction process complex and difficult to understand for government department users. And then they have to choose to believe in algorithms’ accurate and fair, making algorithmic decision-making errors difficult to supervise and correct effectively and immediately.
On the other hand, the black-box nature of the process does not guarantee openness and fairness as well as democratic participation. Government departments are unable to accurately explain the algorithmic decision-making process to the public, making it difficult to gain public support and trust in algorithmic decision-making. The information asymmetry caused by the “algorithm black box” may also affect individuals’ basic rights, such as the right to know and participation and so on.
Additionally, the algorithm black box makes it difficult to define responsibility for execution errors, increasing governance difficulties. For example, in 2018, the UK breast cancer screening failure, mutual accusations between the National Health Service (NHS), the Public Health Agency (PHE), and Hitachi Consulting which responsible for software development (Donnelly, 2018), reflect the difficulty in attributing responsibility when algorithms cause social problems, leading to lengthy and difficult accountability processes.
Algorithmic Discrimination
Algorithmic discrimination may also be one of the main risks associated with algorithmic decision-making. Although decisions based on algorithm logic can achieve relative fairness, the algorithm rules themselves do not inherently possess neutrality, posing challenges to social fairness. This is because algorithm programmers have different cultural cognitive bases and may have some inherent biases themselves, leading to these systems potentially having discriminatory designs (Martin, 2019). Consequently, results derived from algorithmic decision-making are inevitably biased, leading to systemic discrimination in evaluating public decisions. Algorithms may perpetuate existing discrimination (Elbanna & Engesmo, 2020) or even amplify it as they continue to learn and optimize based on past “non-representative” data.
This is likely to result in discrimination and harm to corresponding data populations, leading to a loss of credibility in governance fairness. O’Donovan (2022) claims that the core idea of robodebt is the tactical imposition of administrative burdens on vulnerable groups. The more vulnerable these groups are, the more likely they are to be subjected to disability bullying. Such arithmetic decision-making is also becoming increasingly common in the public sectors for predicting and analysing welfare fraud. In 2019, the Dutch Tax and Customs Administration also used it, resulting in tens of thousands of victims. They considered “dual nationality” and “low income” as significant risk indicators; people of “non-Western appearance” were also among the key targets of the tax authorities (HEIKKILÄ, 2022). Since it is difficult for complex algorithmic decision-making to be completely accurate and reliable in real-life situations, coupled with the difficulty of eliminating human factors in important decisions (especially welfare policies), letting some biases and false data be used in algorithmic systems then leads to biased algorithmic decision-making. Both scandals were eventually declared illegal.
Algorithmic Governance
Human Collaboration and Public Oversight
The essential characteristic of algorithmic decision-making in the public sectors is reflected in the “public,” which includes not only the fairness and justice of decision results but also the openness of decision processes and public participation.
Due to the objective existence of “algorithm black boxes” and “algorithm discrimination,” it is difficult to govern at the level of algorithms themselves. Therefore, human decision-makers need to make fair judgments, interventions, and controls based on real situations during the algorithmic assistance process to ensure that the results of algorithmic decision-making are combined with ethical, legal, and social considerations.
Bullock (2019) mentions that in today’s mainstream view, the advantages of artificial intelligence in decision-making are mainly reflected in the highly repetitive, mechanical, or daily public procedural scenarios. Therefore, especially in public decision-making systems involving citizens, such as life safety, personal freedom, social rights and so on, it is necessary to determine the relationship between humans and algorithms, and humans should have the dominant role in routine decision-making, and algorithms should play an auxiliary and supportive role. Even in some necessary high-risk public policies, algorithmic decision-making should even be avoided. In the European Union, the General Data Protection Regulation (GDPR) requires that algorithmic decisions involving significant impact on any person must have a human review component (Sarder, 2020) or even prohibits the complete automation of certain types of decisions.
Moreover, there is increasing evidence showing that the public has the ability and enthusiasm to participate in the development, deployment, and management of algorithms (Aitken, 2020). Nowadays, it is not enough to inform the public what kind of algorithmic decision-making is being used. Based on algorithm transparency, public governance at the public level should be achieved through negotiation, investigation, voting, and other forms of public participation. By combining the rapid information processing and analysis capabilities of algorithms with human unique insights and thinking methods, fairness and transparency in algorithmic decision-making can be promoted.
Institutional Accountability
Establish specialized institutions to define the consequences of algorithmic decision-making choices in the public sectors and how to hold accountable for algorithmic decision-making choices is also one of the main means of governance.
Although the purposes and application domains of the algorithmic systems used in Robodebt and the A-levels fiasco are different, they have a common feature which is the implementation of each system lacks sufficient supervision and clear legitimacy. Government officials blame the algorithms for these crises and position themselves as victims (O’Donovan, 2023). Therefore, there is a need to establish relevant institutions to clarify mechanisms for post-event accountability. It should first be clarified that the government bears the primary responsibility for algorithmic decision-making in public sectors. Otherwise, public trust in the government will be further undermined, and democracy itself will be damaged.
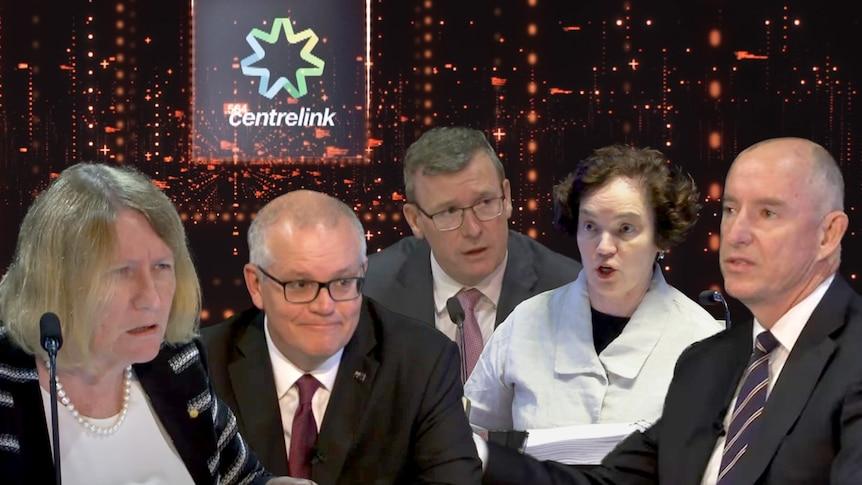
The politicians repeat the same phrases: “I did not know”. (Iorio, k., & Colasimone, D., 2023)
In addition, algorithm companies which provide technical support for commercial purposes also need to be supervised by relevant institutions to prevent them from utilizing technical advantages for potential risks and bringing hidden dangers to government governance.
Furthermore, external forces such as experts in the data field, programmers, and staff with practical experience in government public sectors, can be utilized to form a multifunctional team to supervise algorithmic decision-making to minimize the problems inherent in algorithms and ensure that the government’s core moral principles of “putting people first” are upheld.
Conclusion
The data value and power of algorithms can bring benefits to society, improve government efficiency, and enhance people’s quality of life. However, the relevant authorities should not lose the initiative to make decisions, and the development and implementation of algorithms also need to be transparent, making fair decisions through democratic and fair processes. And through the supervision of multiple related organizations and consideration of ethical issues, the government should assume the corresponding responsibility and clarify the purpose of human-centeredness, so that the algorithmic decision-making is effective and justified.
In today’s government digital transformation, for automated decision-making in the public sectors, it is necessary to build clear accountability and robust supervision, implement human-machine joint decision-making, and find a balance between the dual contradictions of protecting citizens from potential algorithmic harm obligations and improving administrative efficiency.
References
Al-Amoudi, I., & Latsis, J. (2019). Anormative black boxes: Artificial intelligence and health policy. In Post-Human Institutions and Organizations (1st ed., pp. 119–142). Routledge. https://doi.org/10.4324/9781351233477-7
AI-Amoudi, I. (2021, March 17). Artificial intelligence and algorithmic irresponsibility: the devil in the machine? The conversation. https://theconversation.com/artificial-intelligence-and-algorithmic-irresponsibility-the-devil-in-the-machine-157128
Aitken, M. (2020, September 9). Government algorithms are undermining democracy – let’s open up their design to the people. The conversation. https://theconversation.com/government-algorithms-are-undermining-democracy-lets-open-up-their-design-to-the-people-145515
Bullock, J. B. (2019). Artificial Intelligence, Discretion, and Bureaucracy. American Review of Public Administration, 49(7), 751–761. https://doi.org/10.1177/0275074019856123
Burrell, J. (2016). How the machine ‘thinks’: Understanding opacity in machine learning algorithms. Big Data & Society, 3(1), 205395171562251-. https://doi.org/10.1177/2053951715622512
Donnelly, L. (2018, May 4). Breast screening scandal deepens as IT firm says senior health officials ignored its warnings. The telegraph. https://www.telegraph.co.uk/news/2018/05/04/breast-screening-scandal-deepens-firm-says-senior-health-officials/
Elbanna., A & Engesmo, J. (2020, August 19).A-level results: why algorithms get things so wrong – and what we can do to fix them. The conversation. https://theconversation.com/a-level-results-why-algorithms-get-things-so-wrong-and-what-we-can-do-to-fix-them-142879
Evans, T., & Hupe, P. (2020). Discretion and the Quest for Controlled Freedom (1st ed. 2020.). Springer International Publishing. https://doi.org/10.1007/978-3-030-19566-3
Flew, T. (2021). Regulating platforms. Polity Press.ch.3
Harkens, A. (2020, September 4). Not just A-levels: unfair algorithms are being used to make all sorts of government decisions. The conversation. https://theconversation.com/not-just-a-levels-unfair-algorithms-are-being-used-to-make-all-sorts-of-government-decisions-145138
HEIKKILÄ, M. (2022, March 29). Dutch scandal serves as a warning for Europe over risks of using algorithms. Politico. https://www.politico.eu/article/dutch-scandal-serves-as-a-warning-for-europe-over-risks-of-using-algorithms/
Iorio, k., & Colasimone, D. (2023, July 7). Robodebt royal commission final report has been tabled in parliament, as it happened. The ABC news. https://www.abc.net.au/news/2023-07-07/robodebt-royal-commission-final-report-live-updates/102488602
Kleinberg, J., Lakkaraju, H., Leskovec, J., Ludwig, J., & Mullainathan, S. (2018). HUMAN DECISIONS AND MACHINE PREDICTIONS. The Quarterly Journal of Economics, 133(1), 237–293. https://doi.org/10.1093/qje/qjx032
Martin, K. (2019). Ethical Implications and Accountability of Algorithms. Journal of Business Ethics, 160(4), 835–850. https://doi.org/10.1007/s10551-018-3921-3
O’Donovan, D. (2023, March 10). ‘Amateurish, rushed and disastrous’: royal commission exposes robodebt as ethically indefensible policy targeting vulnerable people. The conversation. https://theconversation.com/amateurish-rushed-and-disastrous-royal-commission-exposes-robodebt-as-ethically-indefensible-policy-targeting-vulnerable-people-201165
Redden, J. (2022, September 21). Governments’ use of automated decision-making systems reflects systemic issues of injustice and inequality. The conversation. https://theconversation.com/governments-use-of-automated-decision-making-systems-reflects-systemic-issues-of-injustice-and-inequality-185953
Roose, K. (2019, January 25). The Hidden Automation Agenda of the Davos Elite. The New York Times. https://www.nytimes.com/2019/01/25/technology/automation-davos-world-economic-forum.html
Sarder, M. (2020, June 5). From robodebt to racism: what can go wrong when governments let algorithms make the decisions. The conversation. https://theconversation.com/from-robodebt-to-racism-what-can-go-wrong-when-governments-let-algorithms-make-the-decisions-132594
Zhou, N. (2018, June 24). Centrelink automation hurting Australia’s most vulnerable – Anglicare. The Guardian. https://www.theguardian.com/australia-news/2018/jun/25/centrelink-automation-hurting-australias-most-vulnerable-anglicare
Be the first to comment