A few examples of scams have already sprung up like mushrooms.
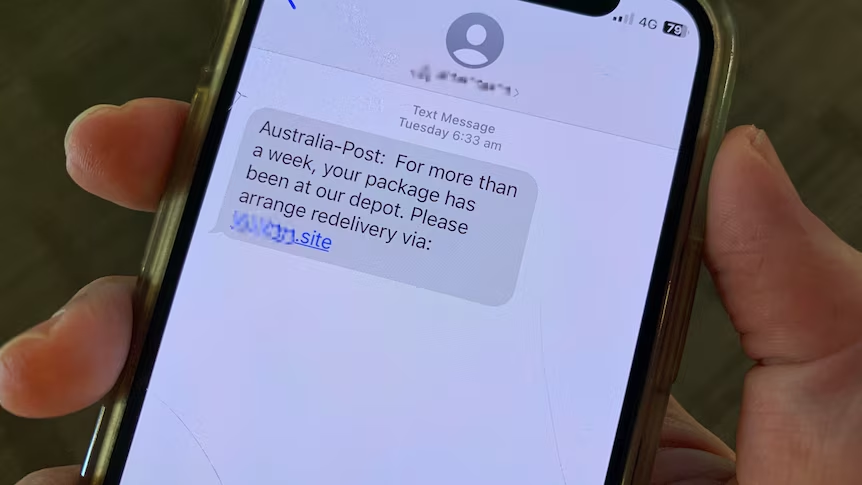
Have you ever received a text message like this? Do you think this message is really from the Australia Post? They could be a scam.
Scams against individuals
“Redelivery scams” and “Schedule delivery scams”
Several people had posted on the Little Red Book (a life platform) that they were scammed for wiping out all the money on the card by clicking a text message link about the redelivery package, which almost looked exactly like the one sent from the Australia Post official. Due to it differing from credit card fraud, the money cannot be recoverable. Many people in the comments section said they also have received messages or phone calls like this regarding their package.
By coincidence, many victims disclose that cyber criminals seem to know their personal information and the status of their packages, such as the name, and phone number. They did not receive their packages for various reasons. Subsequently, they received fraud messages. Scams usually follow a set of processes. The common scams include “Redelivery scams” and “Schedule delivery scams”(Maguire, 2022). First, scammers will send people a message claiming that a parcel could not be delivered, or your details need to be updated. Often scam texts or emails will include a link. It is asking to provide personal information or payment. These links will take victims to a website that looks official but is not. Scammers use these websites to get personal details.
Illegal use of artificial intelligence (AI) systems
If scammers gain access to victims’ personal information they may commit identity theft, fraud, or other misuse. Though sometimes scammers do not directly steal valuables, they collect personal information into AI systems, such as the black market and the dark web to facilitate broader identity fraud (Orsolya, 2024). Not a few victims believe that online fraud uses the leaked personal information of chosen people to build profiles and target them with convincing phishing messages. In most cases, personal information leakage is a primary cause of fraud and other malignant network crimes. Although there were no direct shreds of evidence that could prove that network information disclosure was related to these scams against individuals. But that does not mean internet security and individual information protection can be ignored.
Everything has two sides. AI technology is advancing rapidly while bringing positive change and growth for the thousand trades. But it is also exploited by cybercriminals. Some people might think these intrigues are so easy to expose. However, lawbreakers have myriad opportunities to practice fraud. It will be an incalculable loss when falling into a trap on one occasion.
Fraud on large-scale enterprises
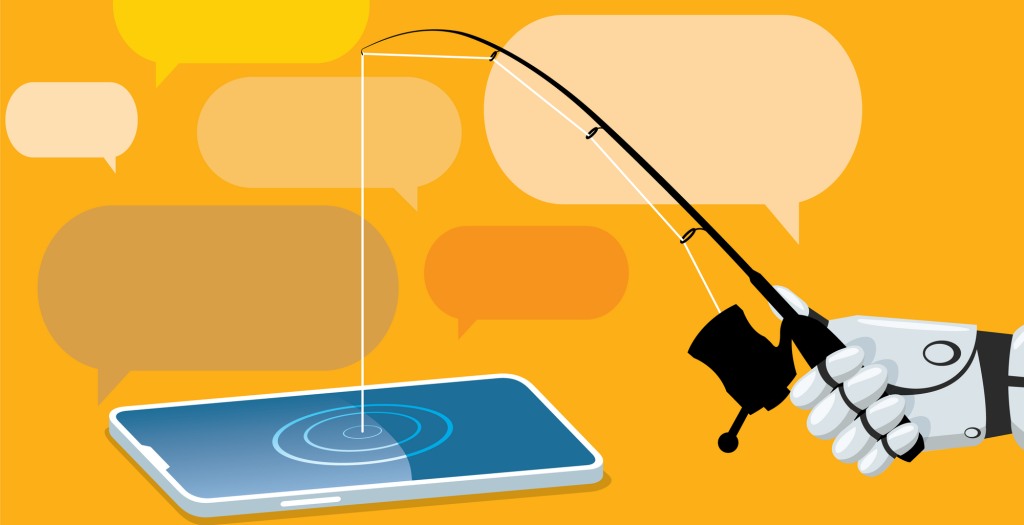
The scams do not only target individuals but are also aimed at large-scale enterprises. According to the report of Cable News Network (2024), earlier this year, a finance worker in a Hong Kong multinational company was involved in a fraud that led this firm to transfer $25.5 million to an attacker who posed as the company’s chief financial officer on an AI-generated deepfake technology conference call. At first, this employee expressed considerable doubt about all this, but he soon dispelled his doubts after the video call. As a matter of fact, there was not one real human during this multi-person video conference, fraudsters used deepfake technology to “modify publicly available video and other footage to cheat”. The police also mentioned that “AI deepfakes had been used to trick facial recognition programs by imitating the people pictured on the identity cards” at least 20 times (Chen & Magramo, 2024). This incident has raised concerns among chief information officers and cybersecurity leaders about the potential for an increase in sophisticated phishing emails and deepfake attacks, and existing technology makes it difficult to detect deceptive behaviour.
What is the impact of AI on society?
AI, Algorithms, and platform: ChatGPT
The above cases are linked to AI, prompting an inquiry into its essence. Crawford (2021) cited Donald Michie’s (1978) technical definition of AI, stating that it can generate a level of codification reliability and competence surpassing even the highest capability achievable by unaided human experts. AI has three main components: algorithms, hardware, and data (Megorskaya, 2022). Machine learning, a subset of AI, as described by McKinsey & Company (2023), involves the development of AI models capable of autonomously “learning” from data patterns without direct human intervention. The growth in the scale and intricacy of data, which exceeds human capacity to manage, has amplified both the potential and necessity of machine learning.
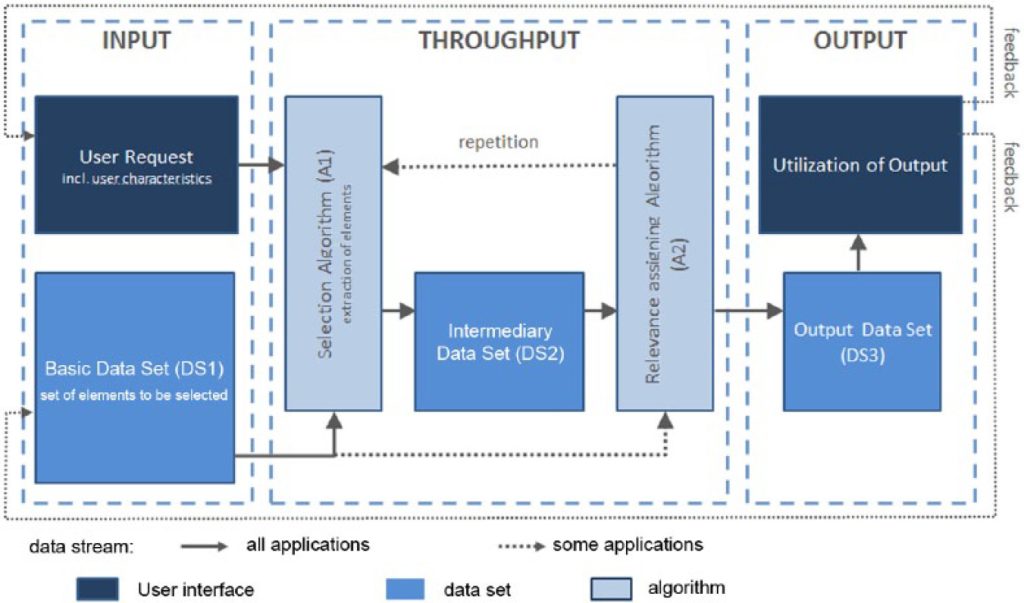
Original Source: Latzer et al. (2014). Cited from Just et al. (2017).
This system aggregates large amounts of data, and using the method of machine learning, algorithms are programmed to identify inter-dependencies within this data and apply this logic to new data encountered (Just et al., 2017). Since the end of 2022, ChatGPT has emerged as the most prominent large language model (LLM), with its ability to deal with natural language processing, handle intricate queries, compose articles, and even program. Algorithms such as generative AI, headed by ChatGPT, are considered tools that can revolutionize human life, thus capturing global attention and provoking widespread public debate.
Large language models can harm: “FraudGPT” or “BadGPT”
Using dark web hacking tools, the malicious user could create a build definition to execute arbitrary code, thereby gaining control of the server and stealing data. Lin (2024) reported that over 200 large-language model hacking services are being sold and disseminated on the dark web, emerging shortly after the public release of ChatGPT. These tools are referred to as “FraudGPT” or “BadGPT”, many of them utilize “versions of open-source AI models” such as Meta’s Llama 2 or unauthorized versions of models developed by companies like OpenAI and Anthropic. These unauthorized models, known as “jailbroken” models, have been manipulated using techniques like “prompt injection” to circumvent their built-in security systems. Lin also emphasized that in a report from cybersecurity vendor Slash Next, phishing emails increased by 1,265% within the 12 months since ChatGPT was publicly released. It means that an average of 31,000 phishing attacks were sent every day.
Urgent Need for Algorithmic Governance
Law, policy, and regulation
To a certain degree, increasing the cost of network crime can deter cyberattacks and lower the crime rate. Therefore, establishing robust network regulatory frameworks appears to be particularly important. Though the development of regulations specific to AI is still at an early stage, various countries formulate policies or laws suitable for national conditions. Such examples include but are not limited to the Human Rights Commission in Australia, the “first-ever legal framework on AI” in Europe and UNESCO’s global policy framework. Most worthy of mention is the Singapore Model AI Governance Framework. According to McCarthy Tétrault Blog (2020), this model framework does not simply expose the ethical principles but also links them to precise measures for implementation. It includes explainability, transparency, fairness in decision-making processes, and a focus on human-centric AI solutions to enhance human capabilities and well-being.
This framework stands on four key pillars:
(1) Internal Governance Structures and Measures, including clear role allocation, risk control, decision-making models, monitoring, communication channels, and staff training.
(2) Determining AI Decision-Making Models, involving coherence of commercial objectives with organizational values, risk assessment, and determining the level of human involvement based on the severity of potential risk.
(3) Operations Management, covering data preparation, ensuring unbiased and representative datasets, algorithm application, and enhancing explainability, repeatability, and traceability of AI algorithms.
(4) Stakeholder Communications, emphasizing transparency, easy-to-understand language, opt-out options, and feedback channels.
Governance by software
Gorwa (2019) has argued that forms of governance for emerging platform regulation include Self-Governance, external governance, and co-governance. Government interventions are labelled as external governance. “Algorithms on the internet are software.” Governance by software is known as the most direct and effective method of Self-Governance and deserves to be taken seriously. Just (2017) has emphasized that software governance is both a governance mechanism and an instrument used to exert power. With the drawing recognition of the importance of software technology in the development of communication systems, technical issues are increasingly viewed as policy, also in the cases of algorithmic. For example, the case of predictive policing. In other words, Algorithmic governance is essentially an example of technical governance. Based on examining vast datasets, it can predict crimes that include details such as the essence, timing, and location of potential incidents. Then, implementing appropriate preventive measures. Algorithmic governance prioritizes the results of managing rather than finding the root causes. That verified the idea of governing AI with AI becomes possible.
Other challenges of Algorithmic Governance
(1) Legal and ethical issues
Based on Algorithmic decision-making, the public raises legal and moral questions regarding the responsibilities and obligations of algorithm developers and users. Especially in cases of adverse outcomes or discriminatory practices, who should be responsible for decisions made by automated systems? Ensuring that algorithmic processes abide by existing laws and ethical guidelines is equally important to maintain public trust and safeguard individual rights. According to McKinsey & Company, (2023), incorporating human oversight in the model’s output verification process and refraining from using generative AI for critical decisions can help minimize risks, which have the same view as the McCarthy Tétrault Blog (2020). The algorithmic decision-making process should conform to “explainable, transparent and fair” and “human-centric”. The primary consideration of designing is not to injure human beings and to ensure their well-being and safety.
(2) Algorithmic bias and unfairness
These models normally produce compelling answers. But sometimes they can be inaccurate or biased. Because of the special characteristics of algorithmics, integration of the existing biases in society and big data is inevitable. This inherent misinformation may exacerbate existing social inequalities. Therefore, algorithmic bias raises the public’s concerns. There is an example related to algorithmic bias leading to inappropriate public decisions. William and Andi (2017) criticized the embedded bias that racial discrimination unfairly favours communities of colour during the process of predictive policing. Resolving algorithmic bias and unfairness is a significant step in promoting fairness, transparency, and equity. To ensure representativeness and diversity, methods for mitigating bias include carefully selecting and preprocessing training data. To foster more equitable outcomes, the diverse data in the teams responsible for developing algorithms can help. It is worth mentioning that is a challenge for private enterprises to expose proprietary algorithms. To a certain extent, organizations must preserve ethical standards and promote fairness and justice by addressing algorithmic bias and unfairness.
(3) Impacts on human behaviors
Algorithmic decision-making has potentially influenced the process of human behaviours, perceptions, and decision-making in significant ways. Katzenbach& Ulbricht (2019) affirms this view and is called as “data deluge”. Though the development of algorithmic tools aims to “hear more voices”, its purpose is to improve the relationship between users and platforms or citizens and political elites. However, there is automated content moderation on social media platforms. Intelligent information recommending systems can kick out user-useless information using filtering algorithms and user profiles, which is the opposite of the original intention. This indicates that algorithms will intensify people’s inherent cognition. Katzenbach& Ulbricht (2019) also emphasizes the risks of datafication and surveillance that create the chance for “social sorting, discrimination, state oppression and the manipulation of consumers and citizens”. Based on “‘data doubles”, to monitor entire populations and compile a comprehensive personal file. Unrestricted surveillance poses a significant threat to human rights and interests, including freedom of expression, collective freedom, and individual privacy.
Conclusion and Suggestions
Due to the generative AI models being a newcomer, the public has yet to see the long-term consequences of it. But it has already posed both known and unknown risks. Some of them can be avoided through a variety of measures. As generative AI becomes more integrated into various fields, policymakers, technologists, and stakeholders should adequately supervise, and work toward developing responsible and impartial algorithmic governance frameworks that promote trust, fairness, and accountability in automated decision-making processes. Given the evolving nature of this field with new models emerging continuously, the authority must remain vigilant.
References
Chen, H. & Magramo, K. (2024, February 4). Finance worker pays out $25 million after video call with deepfake ‘chief financial officer’. Cable News Network. https://edition.cnn.com/2024/02/04/asia/deepfake-cfo-scam-hong-kong-intl-hnk/index.html
Crawford, K. (2021). The Atlas of AI: Power, Politics, and the Planetary Costs of Artificial Intelligence. New Haven, CT: Yale University Press, pp. 1-21.
Gorwa, R. (2019). What is platform governance? Information, Communication & Society, 22(6), 854–871. https://doi.org/10.1080/1369118X.2019.1573914
Just, N., & Latzer, M. (2017). Governance by algorithms: reality construction by algorithmic selection on the Internet. Sage Journals Home. Media, Culture & Society 39 (2), 238-258. https://doi.org/10.1177/0163443716643157
Katzenbach, C. & Ulbricht, L. (2019). Algorithmic governance. Internet Policy Review, 8(4). https://doi.org/10.14763/2019.4.1424
Little Red Book. (2023). About being swindled by AUpost. https://www.xiaohongshu.com/explore/66003561000000001203158a
Lin, B. (2024, February 28). Welcome to the Era of BadGPTs. The Wall Street Journal. https://www.wsj.com/articles/welcome-to-the-era-of-badgpts-a104afa8
Maguire, D. (2022, December 23). Have you been getting messages about unclaimed packages? They could be a scam. ABC News. https://www.abc.net.au/news/2022-12-23/australia-post-unclaimed-mail-scams-christmas-warning/101793048
McKinsey & Company. (2023, January). What is generative AI? McKinsey Global Institute. https://www.mckinsey.com/featured-insights/mckinsey-explainers/what-is-generative-ai#/
Megorskaya, O. (2022, June 22). Training Data: The Overlooked Problem Of Modern AI. Forbes Technology Council. https://www.forbes.com/sites/forbestechcouncil/2022/06/27/training-data-the-overlooked-problem-of-modern-ai/?sh=66d5b2fc218b
Orsolya, T. (2024, March 6). Don’t Get Duped! How the Australia Post Missing Address Scam Targets Victims. MalwareTips. https://malwaretips.com/blogs/australia-post-missing-address-scam/
McCarthy Tétrault Blog. (2020). Singapore model AI governance framework. Newstex. https://www.proquest.com/blogs-podcasts-websites/singapore-model-ai-governance-framework/docview/2389346086/se-2
William, I., & Andi, D. (2017, May 10). Column: Why big data analysis of police activity is inherently biased. PWS News Hour. https://www.pbs.org/newshour/nation/column-big-data-analysis-police-activity-inherently-biased
Be the first to comment