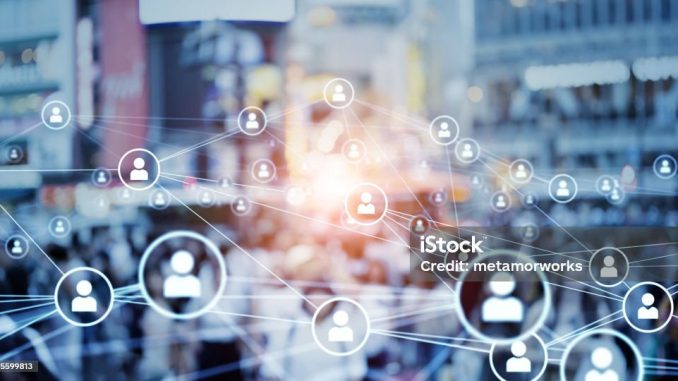
Little Red Book (the following is under the name Red) is a very popular social e-commerce platform in China, where users can share their experiences in shopping, beauty, life, and sports, and directly buy recommended products. Because of the breadth and authenticity of its posts, many young people now prefer to see Red as a search engine, thus replacing Google and Baidu.
However, with the increasing use of Red, in order to improve user stickiness and shopping experience, it uses big data algorithms to make personalized recommendations to users. One of the most common is to recommend relevant posts to users based on their browsing history, likes, favorites and comments. At the same time, Red will also recommend products that meet the user’s tastes and needs according to the purchase history to improve the purchase conversion rate.
Second, personalized recommendations will also be updated in real time, for example, after the user has recently searched for a travel place, Red will frequently recommend the travel tips of the destination, in order to maintain the freshness and accuracy of the recommendation. Sometimes Red takes users’ social connections into account. If one of a follower of the user buys or views something, the system will recommend the same content to that user.
Finally, what is shocking is that in order not to let users’ choices be limited, Red will also recommend content to users according to the algorithm that is similar to their interests to a certain extent, but not exactly the same, to avoid aesthetic fatigue. For example, when a user repeatedly searches for backcountry camping equipment, a few posts will occasionally link fishing and camping, making relevant recommendations to expand the user’s horizons and shopping options. “Processes of media automation go beyond conventional forms of content such as news and advertising to encompass the mediatization and informatization of daily life.” (Andrejevic, 2019, p29)
On one hand, personalized recommendation does bring great convenience to users. To a great extent, it improves user satisfaction and the shopping experience. When recommended, users can compare the same product horizontally and vertically to choose the most satisfactory one, which also maximizes the conversion rate of purchases. As a social platform, it also improves the platform user stickiness, engagement and interaction, and more to improve the competitiveness of the platform.
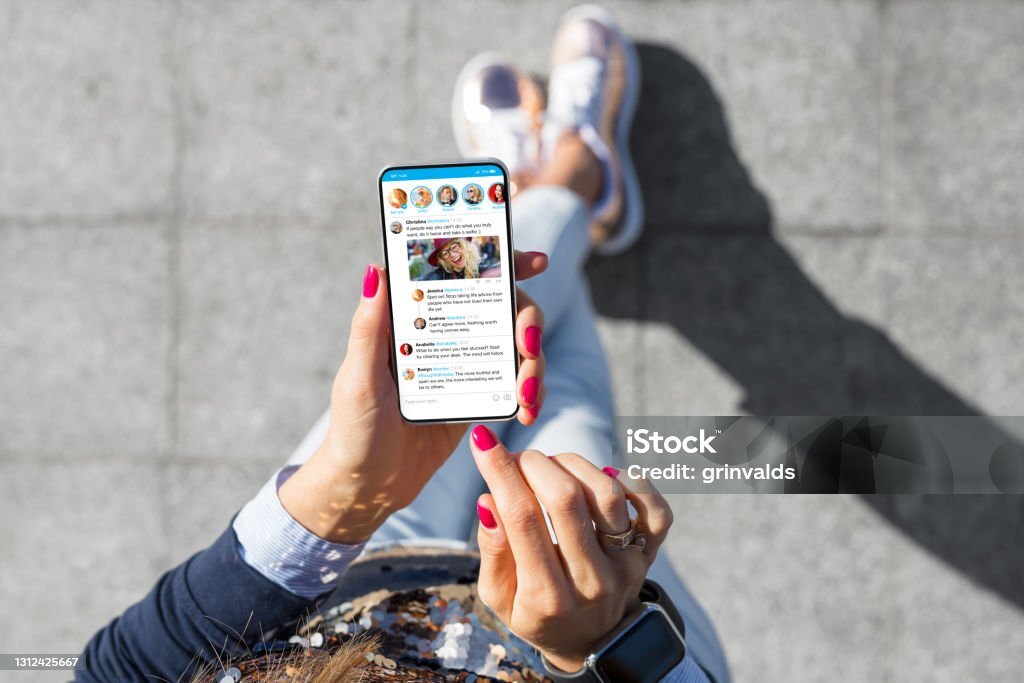
Artificial intelligence is like a giant robot with a high IQ, which has advantages and disadvantages “AI systems are not autonomous, rational, or able to discern anything without extensive, computationally intensive training with large datasets or predefined rules and rewards.” (Crawford, 2021, p8) This also involves the problem of algorithm regulation. First of all, the algorithm greatly affects the user’s behavior. We have to admit that big data algorithms are subtly changing our behavior and thinking. By analyzing user behavior data, preferences, and user relationships, it provides personalized recommendations and services to users, thereby influencing user decisions and behaviors. The fundamental purpose is for a more convenient user experience, but the degree of customization is difficult to grasp.
Excessive customization can lead to information cocooning, which means that users can only see the information that is consistent with their own point of view, and ignore other points of view, thereby limiting their ability to think and judge. This phenomenon is often seen in posts on gender equality in Red. For example, one of the posts is about a day’s life of a couple, in which the girl is contracted to do all the housework in the family, including cooking, cleaning, and picking up and dropping off children from school. What is shocking is that this woman is not only a housewife, she also has her own job, but also manages to balance both family and career. The husband, on the other hand, is invisible in every household chore and only appears when eating and entertaining. “…the images of women in advertising and art are often constructed for viewing by a male subject…” (Noble, 2018, p58) The comments section is deeply polarized. Female commenters were more likely to see comments like “This life is worse than not getting married” or “The wife in the video is really good at managing her time.” Male viewers commented more on “Seeing this video and want to get married.” and “Wives should be like this kind of woman”. This is known as the information cocoon, in which both men and women are locked into a single thought, unable to see the opposite commentary, and even assume that everyone else’s thoughts are similar to their own. It is like letting people live in a huge virtual world, without opposition and criticism, it is impossible to progress. What’s more, personalized recommendations can easily trap users in the information filtering bubble, because the system automatically filters out some information that does not match the user’s interests, so that users miss out on potentially valuable content and products.
The lack of transparency in the recommendation process is also a fundamental problem. Sometimes because of the ambiguity and opacity of the algorithm, it is difficult for users to understand why they see certain recommended content, and thus lack trust in the recommendation results. Because big data algorithms rely too heavily on users’ historical behavior data, if a user suddenly shows a new interest, the recommendation system may not catch it in time. At the same time, for new users and new products, the algorithm cannot accurately recommend. For example, when users search for posts about Disney dolls, they usually search for the keywords “Disney” and “dolls”. However, it is not known whether it is because of the small number of users who recommend the doll alone, or the priority of the algorithm, usually the content of the search will be mixed with a large number of Disney rides. After all, the algorithm is emotionless, and it cannot judge the precise needs only from the user’s behavior, which leads to the user’s distrust and dissatisfaction with the system. However, in order to optimize this aspect, Red should try to improve the transparency of the algorithm, so that users can better understand the operating mechanism of the recommendation system. At the same time, providing users with interaction and feedback mechanisms with the system is also an essential part. In this regard, Weibo has a clear set of blocking keywords. Not only can you simply block a user, but also accurately block a keyword. For example, if a blogger’s post has the user-blocked-word, even if this user does not block this blogger, the post will not be seen.
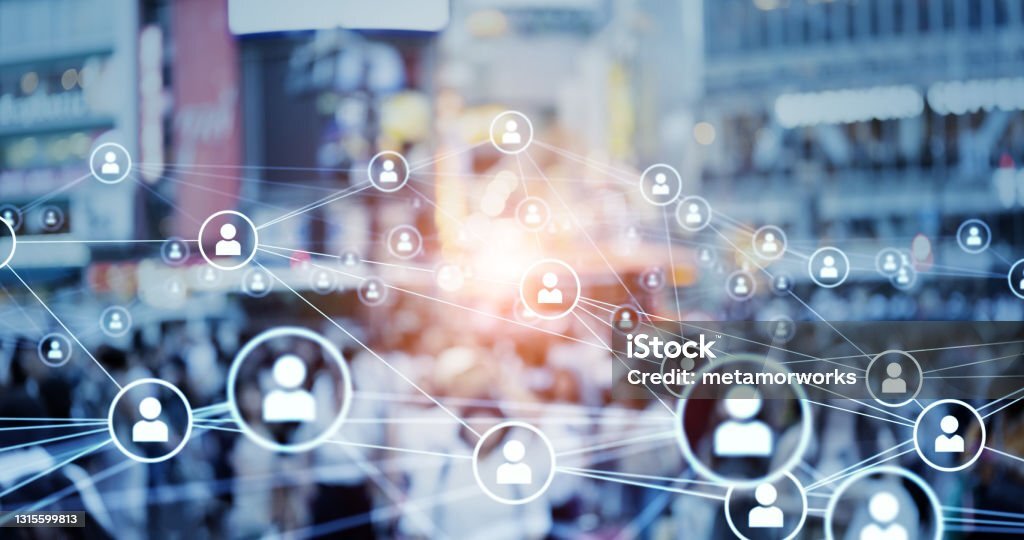
Algorithms can be biased. Because the recommendation is based on the user’s personal characteristics and social behavior, it may unconsciously lead to racial, gender, regional bias, and exacerbate social inequality. For example, older people seem to be more likely to read about topics related to health care, kids are more likely to read about toys and games, and girls seem to be more likely to read about feminism. One of the reasons cannot be ruled out is the content range set by the system according to the age and gender of the user. It is more likely that because the user is interested in a certain topic, he will browse more time on this topic than other aspects, and even occasionally comments, likes and favorites, which makes it easier for the system to recommend relevant content. What’s more, there will always be some groups whose data is larger and more accessible to get, leading the recommendation system to favor the interests and preferences of these groups over others. This also greatly limits the user’s ability to see and access comprehensive information, exacerbating the cognitive bias toward a particular idea or product. “For many, the rules of social media platforms represent arbitrary value judgments about particular standards of decency that limit their ability to express themselves and perpetuate harmful prejudices.” (Suzor, 2019, p22)
Last but not least, who is responsible for a series of problems arising from the collection of big data and manufacturing algorithms made by artificial intelligence? With the development of science and technology, big data has become an inevitable phenomenon in our lives. However, due to the opacity of the algorithm and the imperfect supervision mechanism, this is jeopardizing user safety and experience. “Recommendation engines at Amazon and You Tube affect an automated familiarity, gently suggesting offerings they think we’ll like.” Pasquale said, “But don’t discount the significance of that perhaps. The economic, political, and cultural agendas behind their suggestions are hard to unravel.” (2015, p5) The issue of data privacy is inevitable. The basis of personalized recommendations is undoubtedly a large amount of personal data of users, including browsing history and purchase history. This often leads users to worry about data breaches and misuse.
Also worrying are shopping platforms, taking Taobao as an example, personalized recommendations also seriously endanger personal privacy. First of all, from the user registration on Taobao, all the information has been collected by this platform. This information is then subjected to complex and detailed data analysis and algorithmic processing to identify user behavior patterns and purchasing preferences. This can even involve information that users don’t want to be made public.
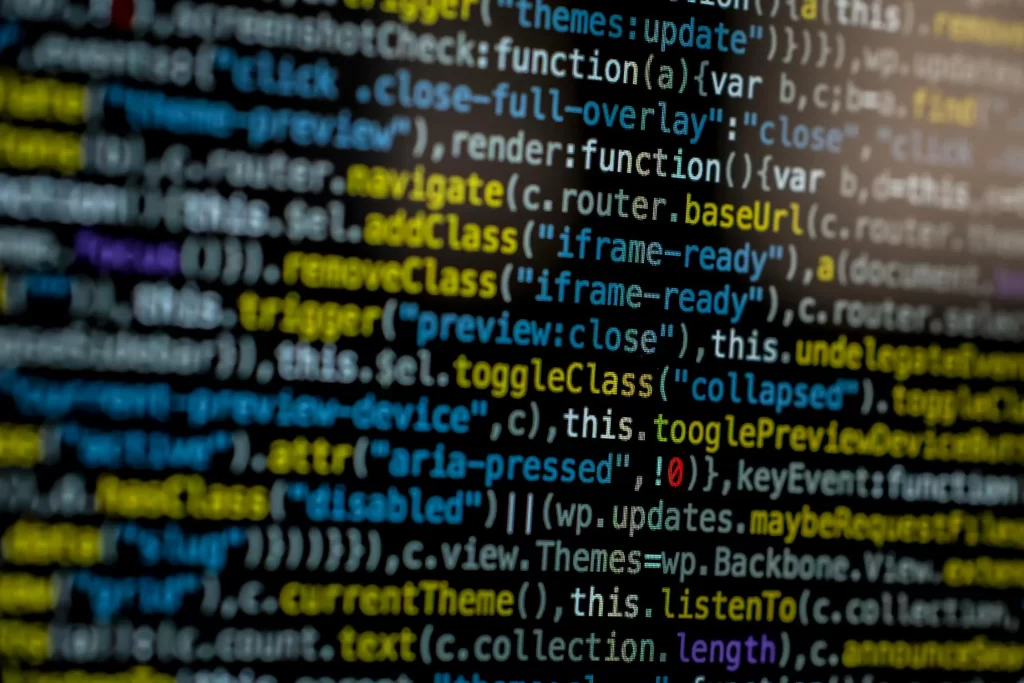
Some time ago, Taobao suddenly launched a new function, called Friend Circle. Taobao will not inform the user in advance, directly post all the purchase of the user in the Friend Circle, which means that there is no privacy at all and all your friends can see what you get. Once the feature was launched, it was resisted by all users. Taobao originally wanted to use this function to make personalized recommendations to users, but did not expect to have the opposite effect.
At the same time, information sharing is also a major concern. Taobao can not only collect personal data from other platforms, such as monitoring WeChat to learn about users’ shopping needs. One user just said yesterday that he wants a certain product, the next day Taobao will automatically recommend the product on the home page to him, which is a very common example in Taobao. Although this behavior is more convenient for users to a certain extent, it has to be admitted that this is a complete violation of user privacy. Moreover, Taobao will also share the information collected with third parties, who may use the information for activities such as advertising targeting, thus further violating users’ privacy. What’s more, even if the data is not leaking when it is collected and used, it is likely to be used for improper purposes, such as discriminatory pricing, which means flexible pricing based on how often users search for a product.
In order to effectively improve the hidden dangers brought by big data algorithms to users. The first step is to change within ourselves. Read the privacy policy before signing up for the software. Learn more about user privacy protection and improve data ethics, and give timely feedback when encountering personalized recommendation bias and privacy leakage.
Second, the platform should also be managed. They should be more transparent about their algorithmic rules and give users more control over whether to participate in personalized recommendations. In fact, a lot of software is now slowly implementing this improvement. Before the user uses, the platform will ask whether the data of other platforms can be synchronized to the software, and the user has two options: yes and no. Although it cannot be said that data collection and sharing can be eliminated, it also protects user security to a certain extent.
“Consistent with the growing awareness of the significance of (software) technology in the evolution of communication systems, technological issues are increasingly seen and treated as policy issues, also in the case of algorithms.” (Just &Latzer, 2017, p242) The state should pay more attention to this situation. Establish laws and regulations on supervision mechanisms and complaint channels to ensure that personalized recommendation systems operate legally and handle user feedback and complaints in a timely manner.
Reference:
- Andrejevic, M. (2019). Automated Media. Routledge.
- Crawford, K. (2021). The atlas of AI power, politics, and the planetary costs of artificial intelligence. Yale University Press.
- Noble, S. U. (2018). Algorithms of oppression : how search engines reinforce racism. New York University Press.
- Suzor, N. P. (2019). Lawless : the secret rules that govern our digital lives. Cambridge University Press.
- Pasquale, F. (2015). The black box society : the secret algorithms that control money and information. Harvard University Press.
- Just, N., & Latzer, M. (2017). Governance by algorithms: reality construction by algorithmic selection on the Internet. Media, Culture & Society, 39(2), 238–258. https://doi.org/10.1177/0163443716643157
Be the first to comment